Machine Learning Prediction Models of Optimal Time for Aortic Valve Replacement in Asymptomatic Patients
INTELLIGENT AUTOMATION AND SOFT COMPUTING(2023)
摘要
Currently, the decision of aortic valve replacement surgery time for asymptomatic patients with moderate-to-severe aortic stenosis (AS) is made by healthcare professionals based on the patient's clinical biometric records. A delay in surgical aortic valve replacement (SAVR) can potentially affect patients' quality of life. By using ML algorithms, this study aims to predict the optimal SAVR timing and determine the enhancement in moderate-to-severe AS patient survival following surgery. This study represents a novel approach that has the potential to improve decision-making and, ultimately, improve patient outcomes. We analyze data from 176 patients with moderate-to-severe aortic stenosis who had undergone or were indicated for SAVR. We divide the data into two groups: those who died within the first year after SAVR and those who survived for more than one year or were still alive at the last followup. We then use six different ML algorithms, Support Vector Machine (SVM), Classification and Regression Tree (C and R tree), Generalized Linear (GL), work (ANN), and Linear Regression (LR), to generate predictions for the best timing for SAVR. The results showed that the SVM algorithm is the best model for predicting the optimal timing for SAVR and for predicting the postsurgery survival period. By optimizing the timing of SAVR surgery using the SVM algorithm, we observed a significant improvement in the survival period after SAVR. Our study demonstrates that ML algorithms generate reliable models for predicting the optimal timing of SAVR in asymptomatic patients with moderate-to-severe AS.
更多查看译文
关键词
Aortic stenosis,aortic valve replacement,machine learning,survival period enhancement,artificial intelligence in cardiology
AI 理解论文
溯源树
样例
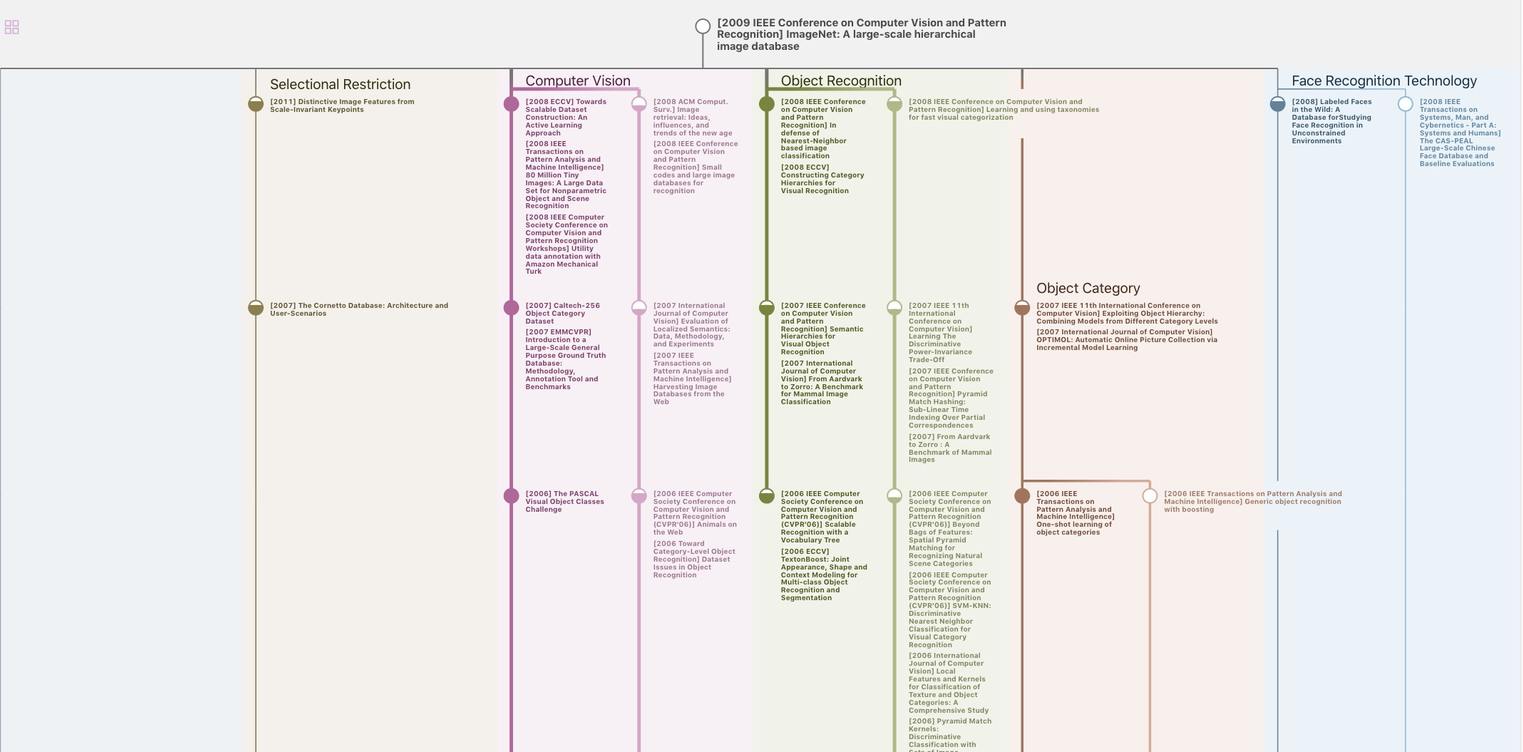
生成溯源树,研究论文发展脉络
Chat Paper
正在生成论文摘要