Variable-length CNNs evolved by digitized chimp optimization algorithm for deep learning applications
MULTIMEDIA TOOLS AND APPLICATIONS(2024)
摘要
One of the most reliable deep learning approaches for image classification challenges is deep Conventional Conv neural networks (DCNNs); however, identifying the appropriate DCNN architecture for a given application can be quite challenging. This study focuses on finding the optimal DCNN architecture automatically using an improved version of the Chimp Optimization Algorithm (ChOA). Three changes based on the baseline ChOA are developed to accomplish the objectives. As a first step, a digitized-based coding strategy is created, making it easier for chimp vectors to encode DCNN layers. Then, to achieve variable-length DCNNs, a disabled layer is recommended to cover some chimp vector dimensions. As a third contribution, a mechanism is developed to assess the fitness function using only a part of the dataset instead of the whole dataset. In order to assess the developed model’s performance, the comparison is made against 23 classifiers, including the top state-of-the-art approaches, using nine benchmark image datasets. The proposed model presents the best performance in the Fashion dataset with an error percentage of 5.08, while it is the second-best model with 750 k parameters. Also, for other datasets, the experimental findings indicate that the suggested method’s classification accuracy outperforms other benchmarks in 87 out of 95 investigations. This variable-length approach is the first effort of its kind, employing ChOA to evolve the architectures of DCNNs autonomously.
更多查看译文
关键词
Chimp optimization algorithm,Image classification,Digitized-based coding,ChOA
AI 理解论文
溯源树
样例
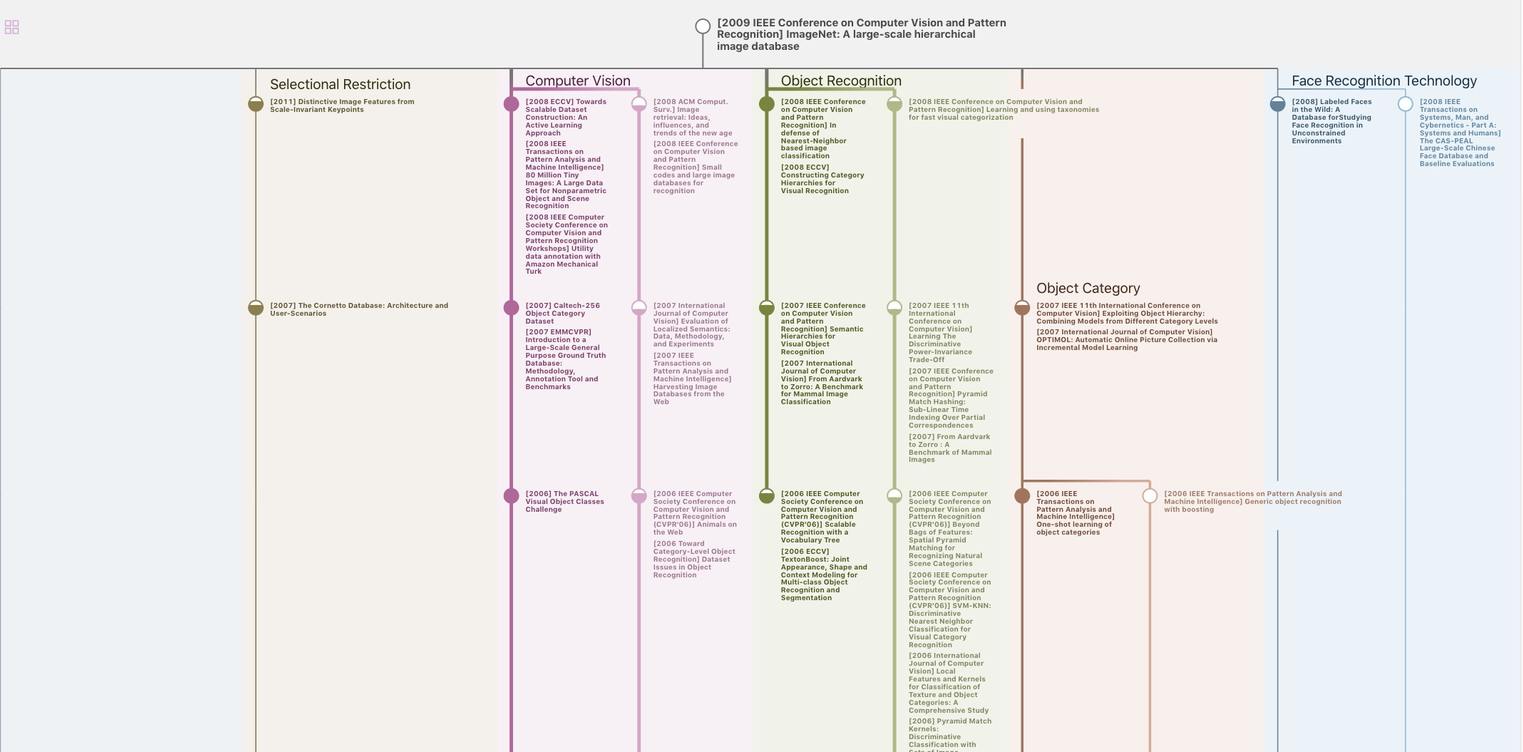
生成溯源树,研究论文发展脉络
Chat Paper
正在生成论文摘要