A novel spatio-temporal hybrid neural network for remaining useful life prediction
JOURNAL OF SUPERCOMPUTING(2023)
摘要
Remaining useful life (RUL) prediction is a crucial mission for the prognostic and health management (PHM) of machinery equipment in modern industry. Due to ignorance of the spatial relationship among multiple sensors, the current RUL prediction methods do not perform adequately in terms of accuracy and comprehensive performance. In order to improve the performance, this paper proposes a novel spatio-temporal hybrid neural network (STHNN) method to capture spatio-temporal features of the time sequence signals from multi-sensors at different locations on mechanical equipment. In this method, the spatial relationship among sensors is constructed by a sensor graph based on the cosine similarity among them. Spatial features of the sensor graph are then extracted by the improved residual graph attention module, which is the first part of the spatio-temporal hybrid neural network. As the second part of the STHNN, the Transformer encoder module is employed to obtain the temporal features. At the same time, this study proposes a new evaluation metric named as comprehensive evaluation function (CEF) to assess overall performance of the STHNN on the entire dataset. To confirm the accuracy and validity of the method, experiments are conducted on the commercial modular aero-propulsion system simulation (C-MAPSS) dataset. The comparison with existing state-of-the-art methods on the same dataset demonstrates performance of the provided STHNN is significantly greater than the current methods.
更多查看译文
关键词
Remaining useful life prediction,Spatio-temporal hybrid neural network,Graph attention,Transformer encoder
AI 理解论文
溯源树
样例
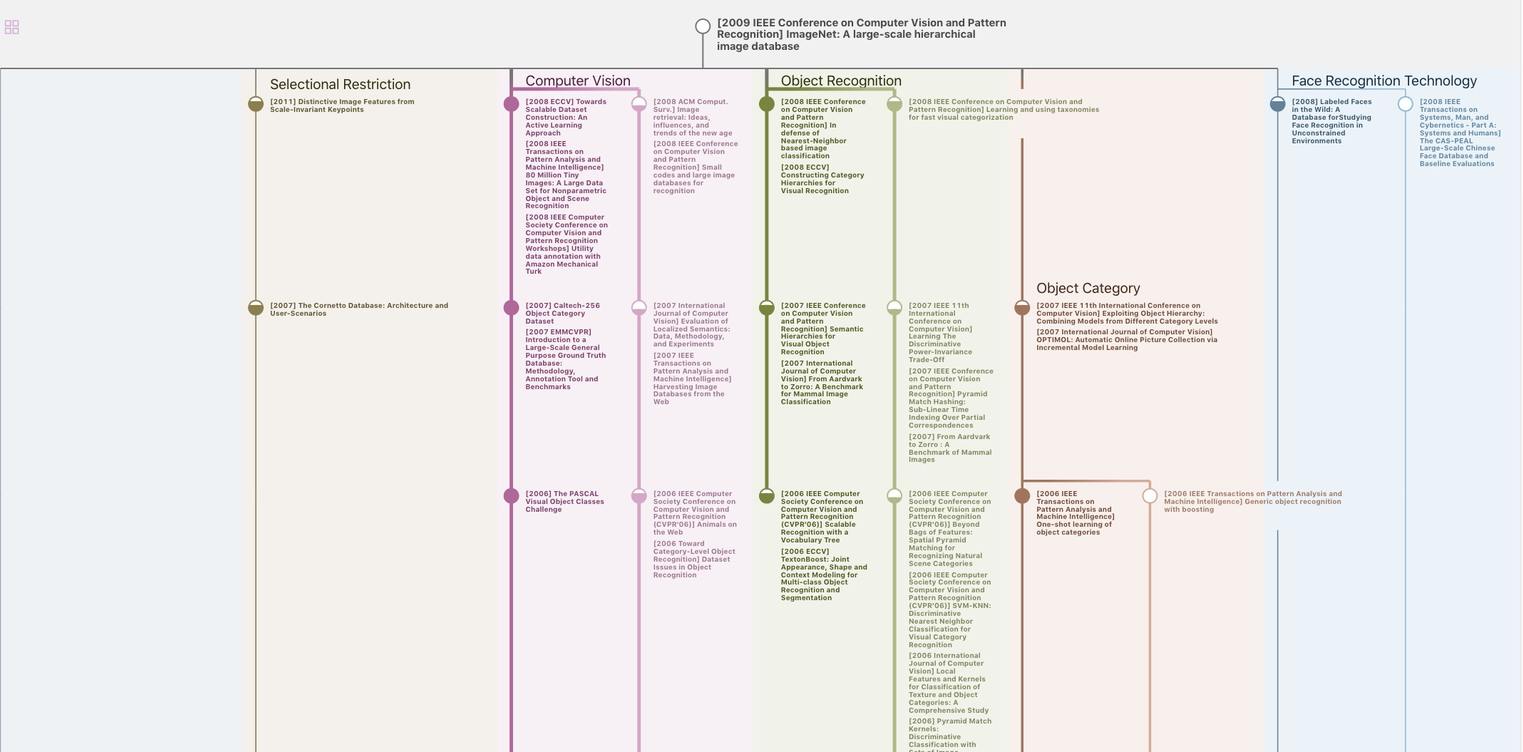
生成溯源树,研究论文发展脉络
Chat Paper
正在生成论文摘要