Method for Classifying Apple Leaf Diseases Based on Dual Attention and Multi-Scale Feature Extraction
AGRICULTURE-BASEL(2023)
摘要
Image datasets acquired from orchards are commonly characterized by intricate backgrounds and an imbalanced distribution of disease categories, resulting in suboptimal recognition outcomes when attempting to identify apple leaf diseases. In this regard, we propose a novel apple leaf disease recognition model, named RFCA ResNet, equipped with a dual attention mechanism and multi-scale feature extraction capacity, to more effectively tackle these issues. The dual attention mechanism incorporated into RFCA ResNet is a potent tool for mitigating the detrimental effects of complex backdrops on recognition outcomes. Additionally, by utilizing the class balance technique in conjunction with focal loss, the adverse effects of an unbalanced dataset on classification accuracy can be effectively minimized. The RFB module enables us to expand the receptive field and achieve multi-scale feature extraction, both of which are critical for the superior performance of RFCA ResNet. Experimental results demonstrate that RFCA ResNet significantly outperforms the standard CNN network model, exhibiting marked improvements of 89.61%, 56.66%, 72.76%, and 58.77% in terms of accuracy rate, precision rate, recall rate, and F1 score, respectively. It is better than other approaches, performs well in generalization, and has some theoretical relevance and practical value.
更多查看译文
关键词
apple leaf diseases,dual attention,multi-scale
AI 理解论文
溯源树
样例
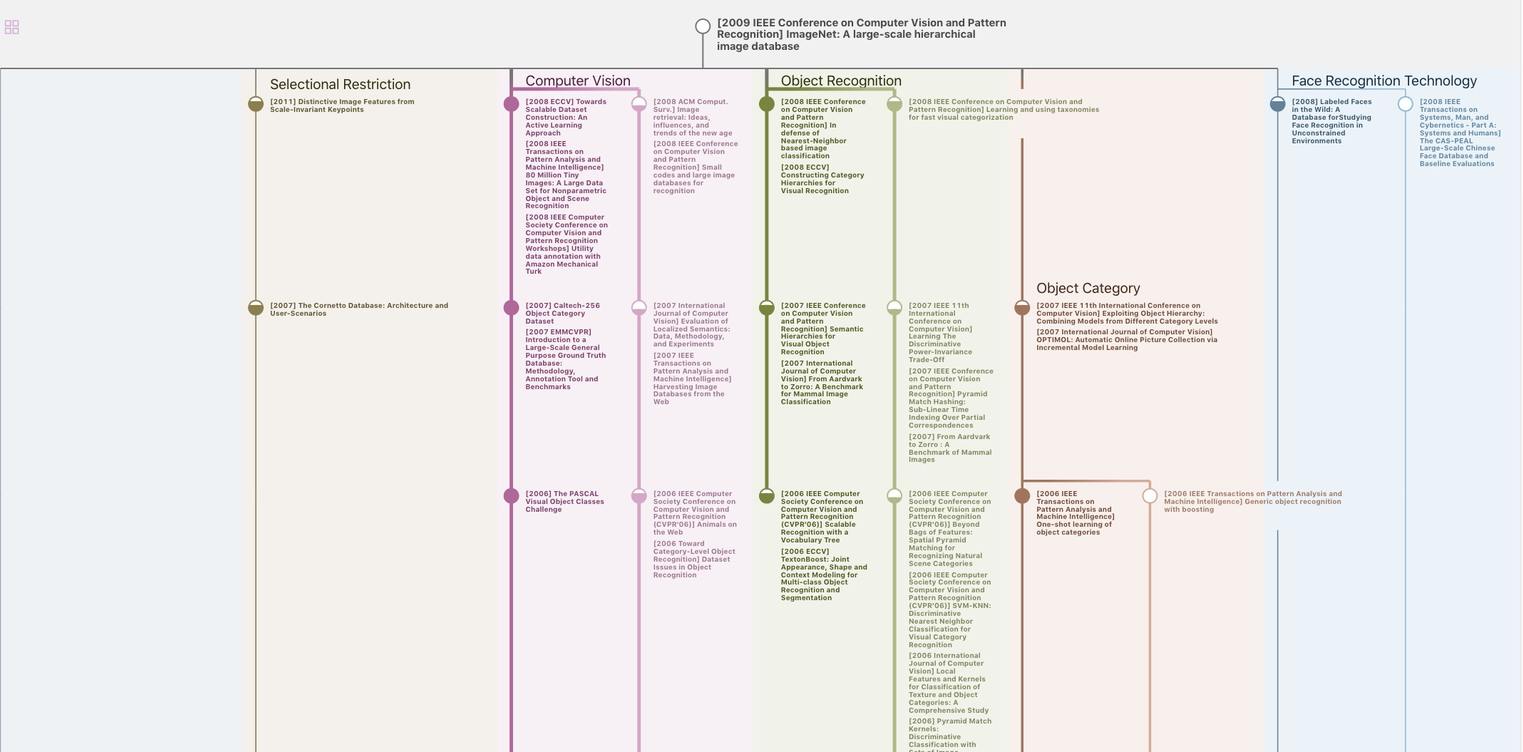
生成溯源树,研究论文发展脉络
Chat Paper
正在生成论文摘要