Label-noise robust classification with multi-view learning
Science China Technological Sciences(2023)
摘要
Label noise is often contained in the training data due to various human factors or measurement errors, which significantly causes a negative effect on classifiers. Despite many previous methods that have been proposed to learn robust classifiers, they are mainly based on the single-view feature. On the other hand, although existing multi-view classification methods benefit from the more comprehensive information, they rarely consider label noise. In this paper, we propose a novel label-noise robust classification model with multi-view learning to overcome these limitations. In the proposed model, not only the classifier learning but also the label-noise removal can benefit from the multi-view information. Specifically, we relax the label matrix of the basic multi-view least squares regression model, and develop a nonlinear transformation with a natural probabilistic approximation in the process of labels, which is conveniently optimized and beneficial to improve the discriminative ability of classifiers. Moreover, we preserve the intrinsic manifold structure of multi-view data on the relaxed label matrix, facilitating the process of label relaxation. For optimizing the proposed model with the nonlinear transformation, we derive a lemma about the partial derivation of the softmax related function, and develop an efficient alternating algorithm. Experimental evaluations on six real-world datasets confirm the advantages of the proposed method, compared to the related state-of-the-art methods.
更多查看译文
关键词
label noise,multi-view learning,classification,robust,least squares regression,label relaxation
AI 理解论文
溯源树
样例
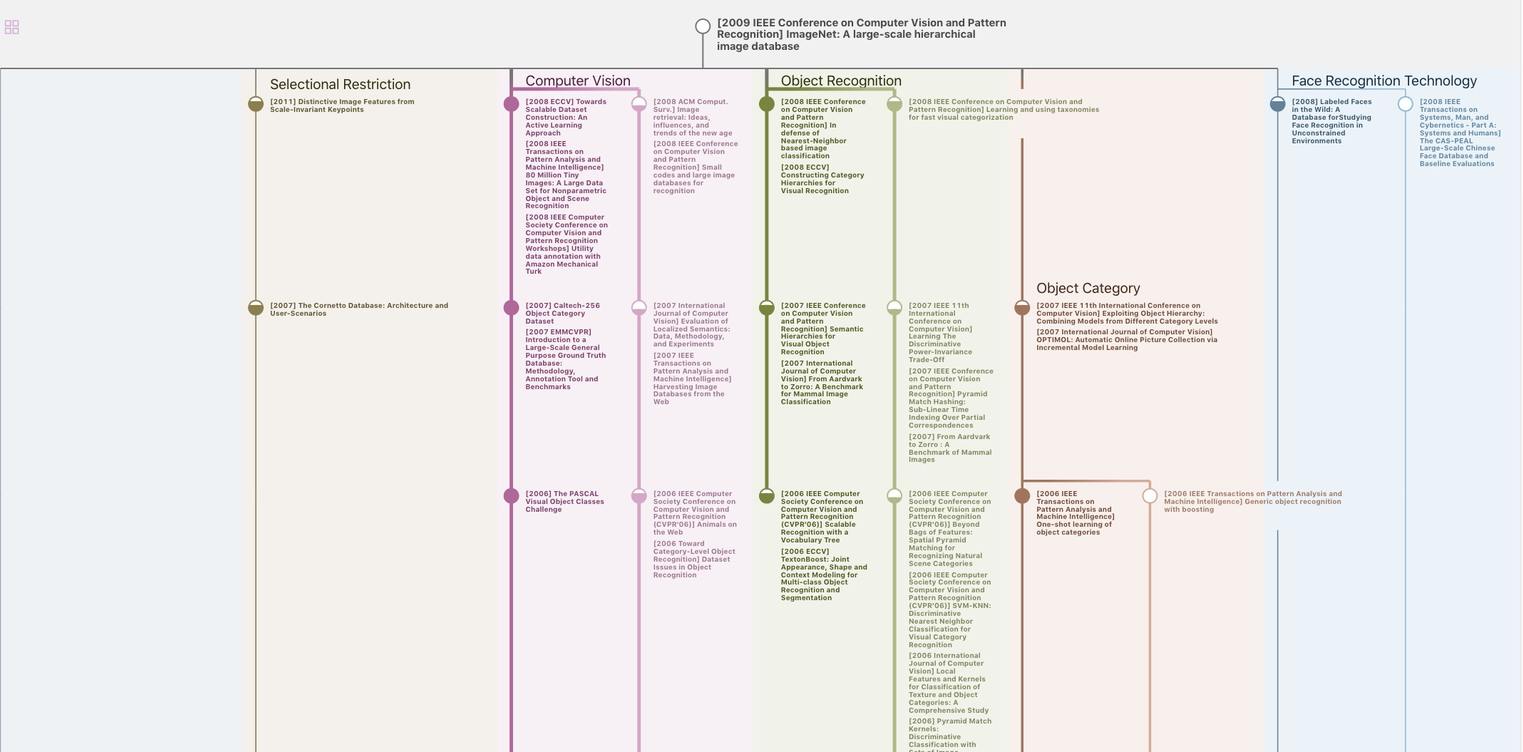
生成溯源树,研究论文发展脉络
Chat Paper
正在生成论文摘要