Long-Tailed Metrics and Object Detection in Camera Trap Datasets
APPLIED SCIENCES-BASEL(2023)
摘要
With their advantages in wildlife surveys and biodiversity monitoring, camera traps are widely used, and have been used to gather massive amounts of animal images and videos. The application of deep learning techniques has greatly promoted the analysis and utilization of camera trap data in biodiversity management and conservation. However, the long-tailed distribution of the camera trap dataset can degrade the deep learning performance. In this study, for the first time, we quantified the long-tailedness of class and object/box-level scale imbalance of camera trap datasets. In the camera trap dataset, the imbalance problem is prevalent and severe, in terms of class and object/box-level scale. The camera trap dataset has worse object/box-level scale imbalance, and too few samples of small objects, making deep learning more challenging. Furthermore, we used the BatchFormer module to exploit sample relationships, and improved the performance of the general object detection model, DINO, by up to 2.9% and up to 3.3% in terms of class imbalance and object/box-level scale imbalance. The experimental results showed that the sample relationship was simple and effective, improving detection performance in terms of class and object/box-level scale imbalance, but that it could not make up for the low number of small objects in the camera trap dataset.
更多查看译文
关键词
object detection,metrics,long-tailed
AI 理解论文
溯源树
样例
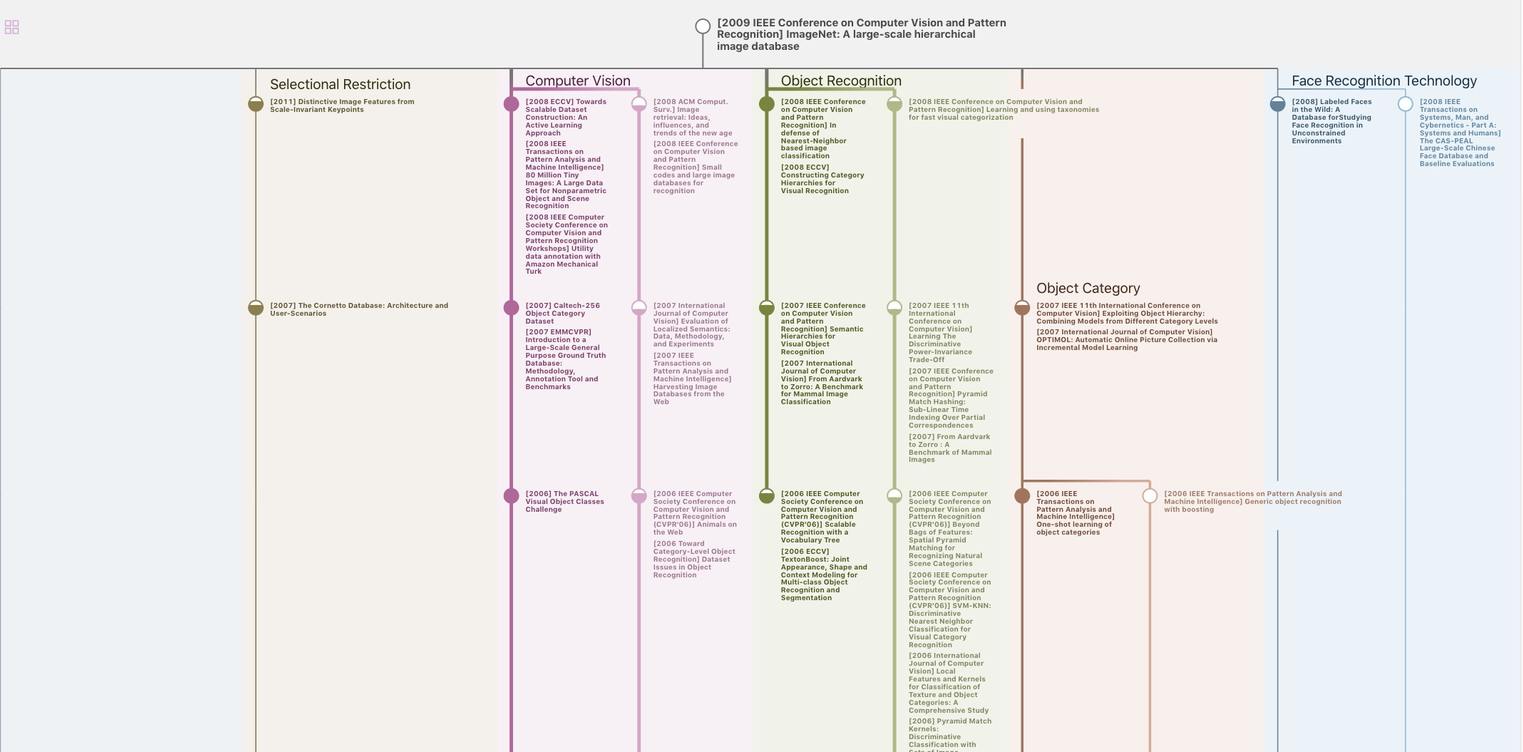
生成溯源树,研究论文发展脉络
Chat Paper
正在生成论文摘要