Automatic discrimination of species within theEnterobacter cloacaecomplex using MALDI-TOF Mass Spectrometry and supervised algorithms
bioRxiv (Cold Spring Harbor Laboratory)(2021)
摘要
TheEnterobacter cloacaecomplex (ECC) encompasses heterogeneous clusters of species that have been associated with nosocomial outbreaks. These species may host different acquired antimicrobial resistance and virulence mechanisms and their identification are challenging. This study aims to develop predictive models based on MALDI-TOF MS spectral profiles and machine learning for species-level identification.A total of 198 ECC and 116K. aerogenesclinical isolates from the University Hospital Ramón y Cajal (Spain) and the University Hospital Basel (Switzerland) were included. The capability of the proposed method to differentiate the most common ECC species (E. asburiae, E. kobei, E. hormaechei, E. roggenkampii, E. ludwigii, E. bugandensis) andK. aerogeneswas demonstrated by applying unsupervised hierarchical clustering with PCA pre-processing. We observed a distinctive clustering ofE. hormaecheiandK. aerogenesand a clear trend for the rest of the ECC species to be differentiated over the development dataset. Thus, we developed supervised, non-linear predictive models (Support Vector Machine with Radial Basis Function and Random Forest). The external validation of these models with protein spectra from the two participating hospitals yielded 100% correct species-level assignment forE. asburiae, E. kobei, andE. roggenkampiiand between 91.2% and 98.0% for the remaining ECC species. Similar results were obtained with the MSI database developed recently (https://msi.happy-dev.fr/) except in the case ofE. hormaechei, which was more accurately identified by Random Forest.In short, MALDI-TOF MS combined with machine learning demonstrated to be a rapid and accurate method for the differentiation of ECC species.
更多查看译文
关键词
mass spectrometry,species,supervised,maldi-tof
AI 理解论文
溯源树
样例
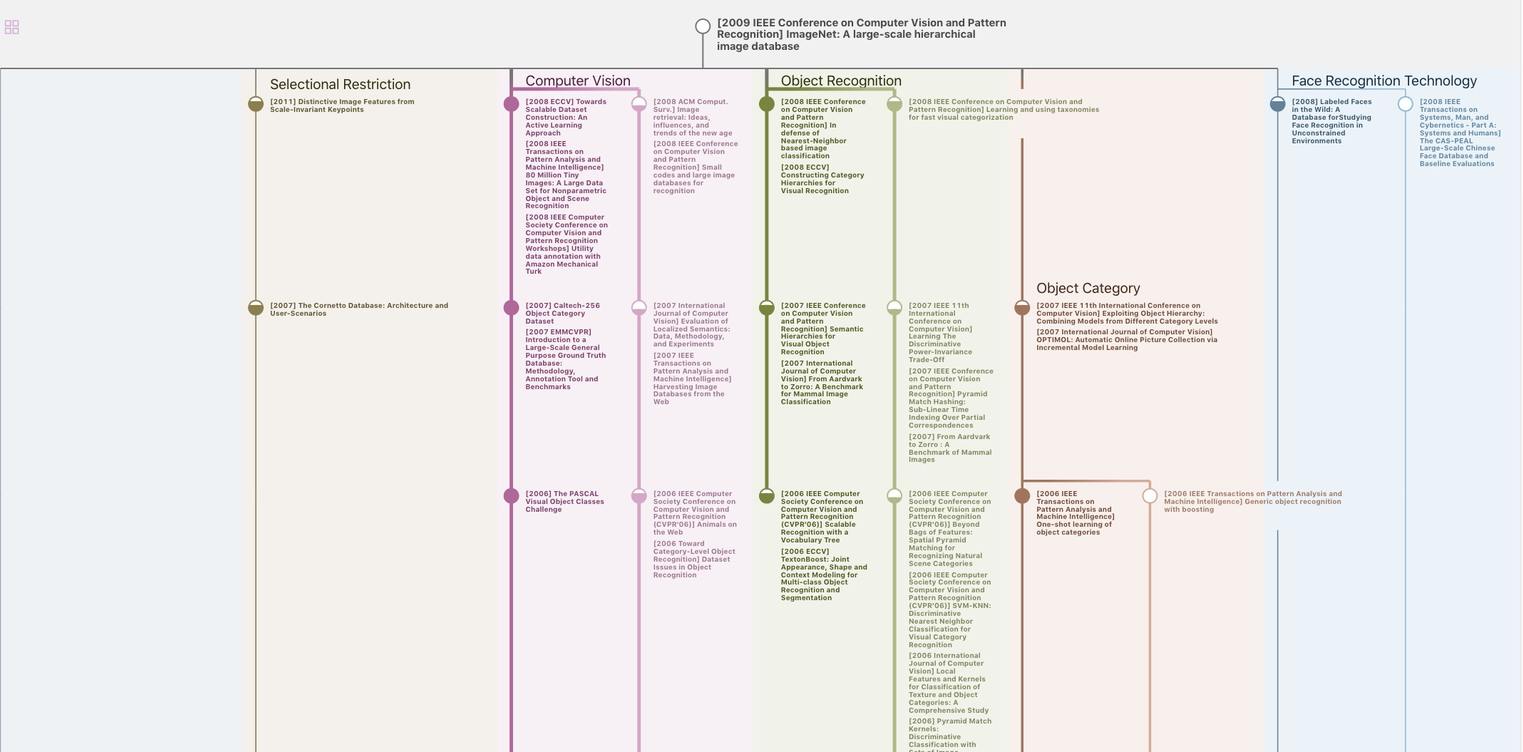
生成溯源树,研究论文发展脉络
Chat Paper
正在生成论文摘要