MS-SST: Single Image Reconstruction-Based Stain-Style Transfer for Multi-Domain Hematoxylin & Eosin Stained Pathology Images
IEEE Access(2023)
摘要
In digital pathology, pathological tissue images that are obtained using scanners are analyzed and diseases are diagnosed. One crucial aspect of this process is the staining of the tissue slides. However, differences appear in the staining color even when using the same staining protocol owing to various factors such as different facilities, hospitals, and scanning equipment. Many stain style normalization studies have been conducted to solve this problem. In this study, we propose a model named multi-domain single image reconstruction-based stain-style transfer. The proposed model is trained using a reconstruction-based learning framework, which can efficiently reduce the complexity and training time compared with that associated with the GAN objective. We randomly extracted stained tissue image patches from the CAMELYON17 and Mitos-Atypia-14 datasets and demonstrated an effective stain-style translation. Our study reveals that it is possible to perform translation among multiple domains using a single training image per domain. Furthermore, we experimentally demonstrated that translation among color temperature domains was possible in the natural image domain. Our code is publicly available at: https://github.com/jwkweon/MS-SST.
更多查看译文
关键词
Digital pathology,stain normalization,multi-domain translation,stain-style transfer,H&E stained histopathology image
AI 理解论文
溯源树
样例
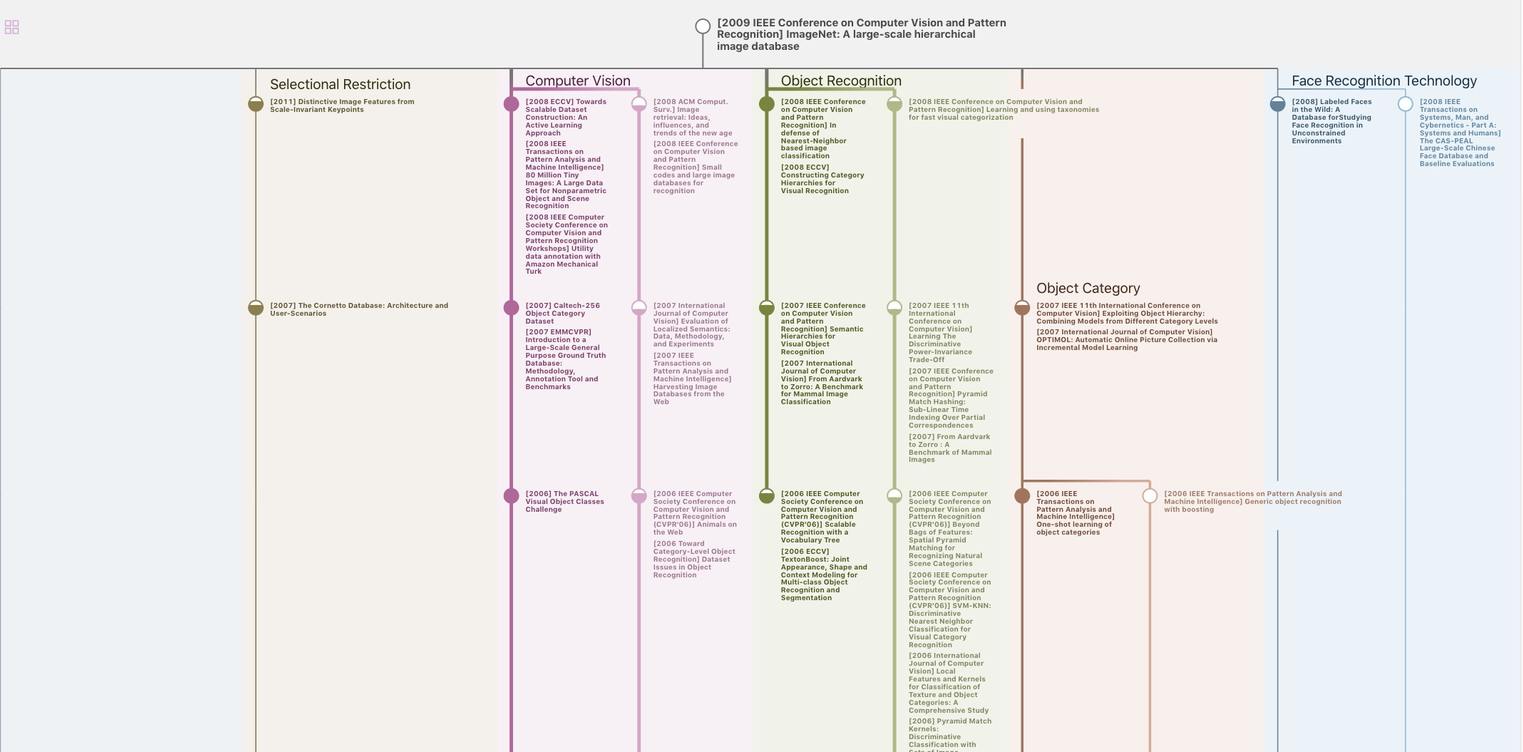
生成溯源树,研究论文发展脉络
Chat Paper
正在生成论文摘要