Deep Convolutional Neural Networks for the Classification and Detection of Human Vocal Exclamations of Panic in Subway Systems.
IEEE Access(2023)
摘要
The automated classification and detection of vocal exclamations of panic made by human beings in subway systems can enable more effective emergency response. Thus, in this study, we designed four multiscale deep convolutional neural networks (models 1-4) with one- and two-dimensional layers for detecting and classifying vocal exclamations of panic. First, we applied a decision-making framing-padding algorithm formulated to preprocess vocal exclamations of panic. Vocal sounds were then mixed with noise signals. Mel spectrogram, log-Mel spectrogram, and signal waveform data were used as learning data. The implementation of an ensemble technique in model 1 improved classification performance by 0.25% and 0.75% in terms of the F1 score at signal-to-noise ratios (SNRs) of 15 and -15, respectively. Models 4 and 2 exhibited the best classification performance and achieved F1 scores of 99.74% (under SNR = 15) and 80.56% (under SNR = -15), respectively. Model 2 performed the best in detecting screaming, quarrelling, and loud talking when SNR = 15 (F1 scores of 94.59%, 49.06%, and 64.94%, respectively). Model 2 also performed the best in distinguishing screaming and non-screaming. Our models outperformed their state-of-the-art counterparts in detection and classification at SNRs of 15 and 10.
更多查看译文
关键词
human vocal exclamations,deep convolutional neural networks,convolutional neural,panic,neural networks
AI 理解论文
溯源树
样例
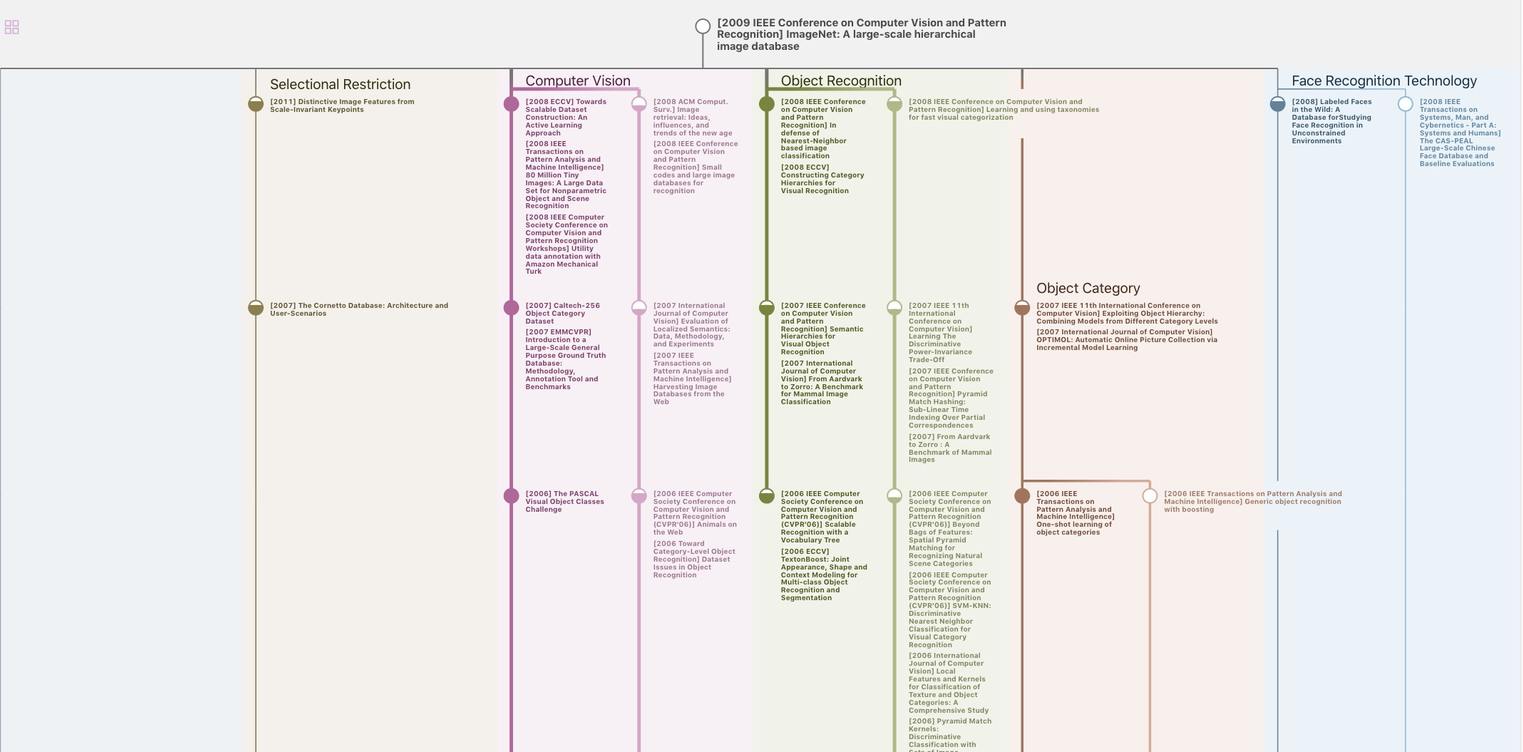
生成溯源树,研究论文发展脉络
Chat Paper
正在生成论文摘要