A Comprehensive Survey of RGB-Based and Skeleton-Based Human Action Recognition.
IEEE Access(2023)
摘要
With the advancement of computer vision, human action recognition (HAR) has shown its broad research worth and application prospects in a wide range of fields such as intelligent security, automatic driving and human-machine interaction. Based on the type of data captured by cameras and sensors, e.g., RGB, depth, skeleton, and infrared data, HAR methods can be classified into RGB-based and skeleton-based. RGB data is easy and inexpensive to obtain, but RGB-based methods need to cope with a large amount of irrelevant background information and are easily affected by factors such as lighting and shooting angle. The skeleton-based methods eliminate the impact of background variables and require little computational work due to their skeleton-focused features, but they lack the context data necessary for HAR. This paper gives a thorough survey of these two approaches, covering deep learning methods, handcrafted feature extraction methods, common datasets, challenges, and future research directions. The skeleton-based action recognition methods Section specifically presents the most well-liked 2D and 3D pose estimation algorithms. This survey aims to give researchers new to the area or engaged in a long-term study a selection of datasets and algorithms, as well as an overview of the present issues and expected future directions in the field.
更多查看译文
关键词
Feature extraction, Skeleton, Deep learning, Pose estimation, Convolution, X3D, Solid modeling, Action dataset, deep learning, pose estimation, RGB-based action recognition, skeleton-based action recognition, systematic survey
AI 理解论文
溯源树
样例
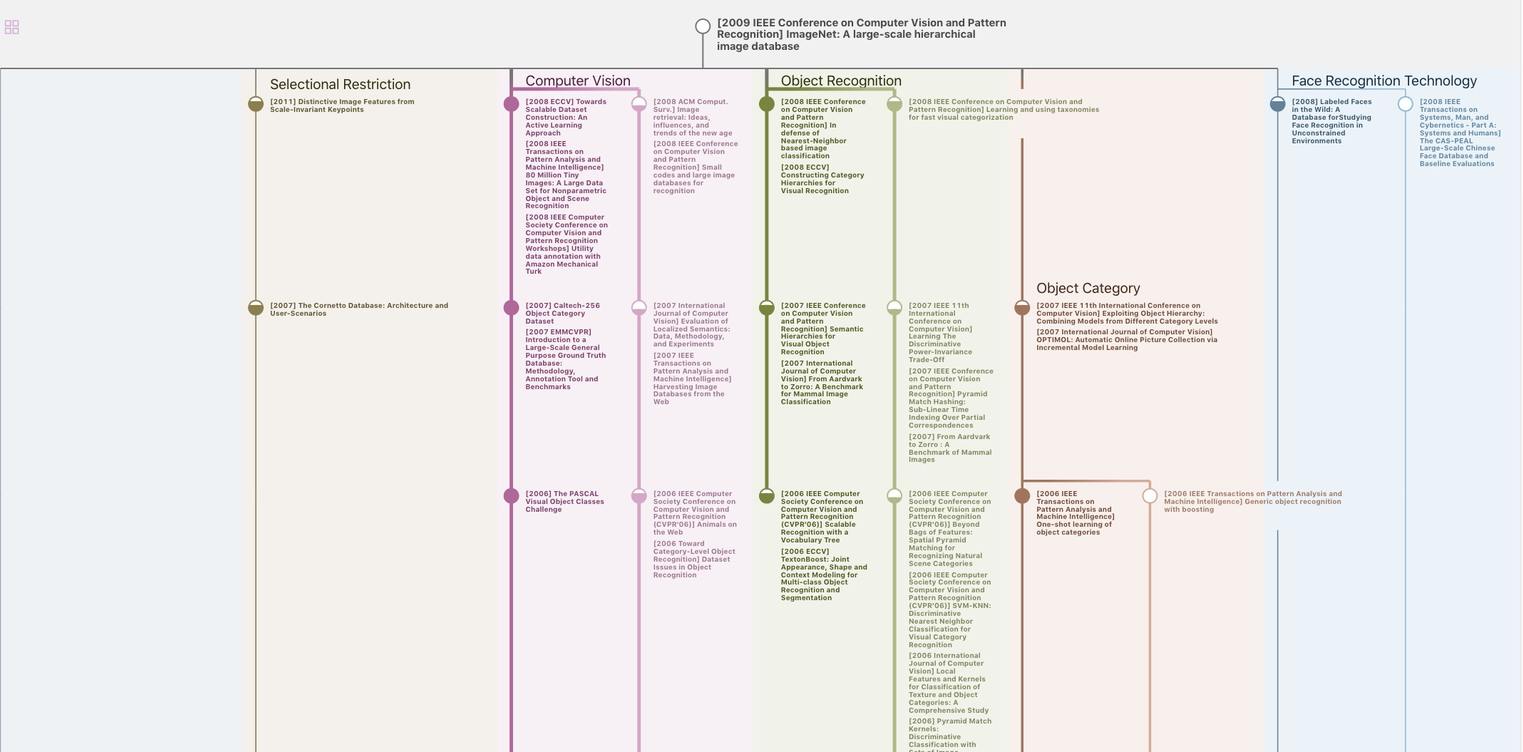
生成溯源树,研究论文发展脉络
Chat Paper
正在生成论文摘要