ESRTMDet: An End-to-End Super-Resolution Enhanced Real-Time Rotated Object Detector for Degraded Aerial Images
IEEE J. Sel. Top. Appl. Earth Obs. Remote. Sens.(2023)
摘要
degradation of image resolution reduces the detection performance in aerial imagery because it generates a large number of small objects, and accurately detecting these small objects remains a challenge. Existing methods mostly use a superresolution (SR) model to first obtain the SR image of the low-resolution degraded image (I-LR) and then use this image as the input of the object detection (OD) network to solve this problem. However, this architecture that involves executing a complex SR network before the detector is time-consuming and makes it hard to achieve real-time model inference. To address this challenge, we propose a simple and effective rotated small OD method, named end-to-end superresolution enhanced real-time rotated object detector (ESRTMDet). First, we design a lightweight embedded feature map superresolution module (ESRM) embedded in the detection model to enhance and amplify the backbone output features, making the detection heads detect small objects more easily. Furthermore, we train a parallel SR network branch (PSRB) simultaneously that uses the backbone feature to restore a high-resolution image. Through our proposed feature alignment loss and feature affinity layer, our PSRB effectively guides the feature map enhancement of ESRM. Finally, through end-to-end joint optimization of the detector and PSRB, the detection performance on I-LR is significantly improved. Extensive experiments over DOTA and UCAS-AOD demonstrate that our method can achieve state-of-the-art results. In addition, we discard our PSRB and use I-LR as the input during inference, reducing the inference time-consuming of our model. Therefore, our ESRTMDet-X not only achieves 77.11% mean of average precision on the degraded DOTA dataset, but also achieves an amazing inference speed of 337 FPS, thus obtaining the best speed-accuracy tradeoff.
更多查看译文
关键词
Object detection,Feature extraction,Superresolution,Detectors,Training,Remote sensing,Real-time systems,Aerial image,deep learning,remote sensing,rotated object detection (ROD),small object detection (SOD),superresolution (SR)
AI 理解论文
溯源树
样例
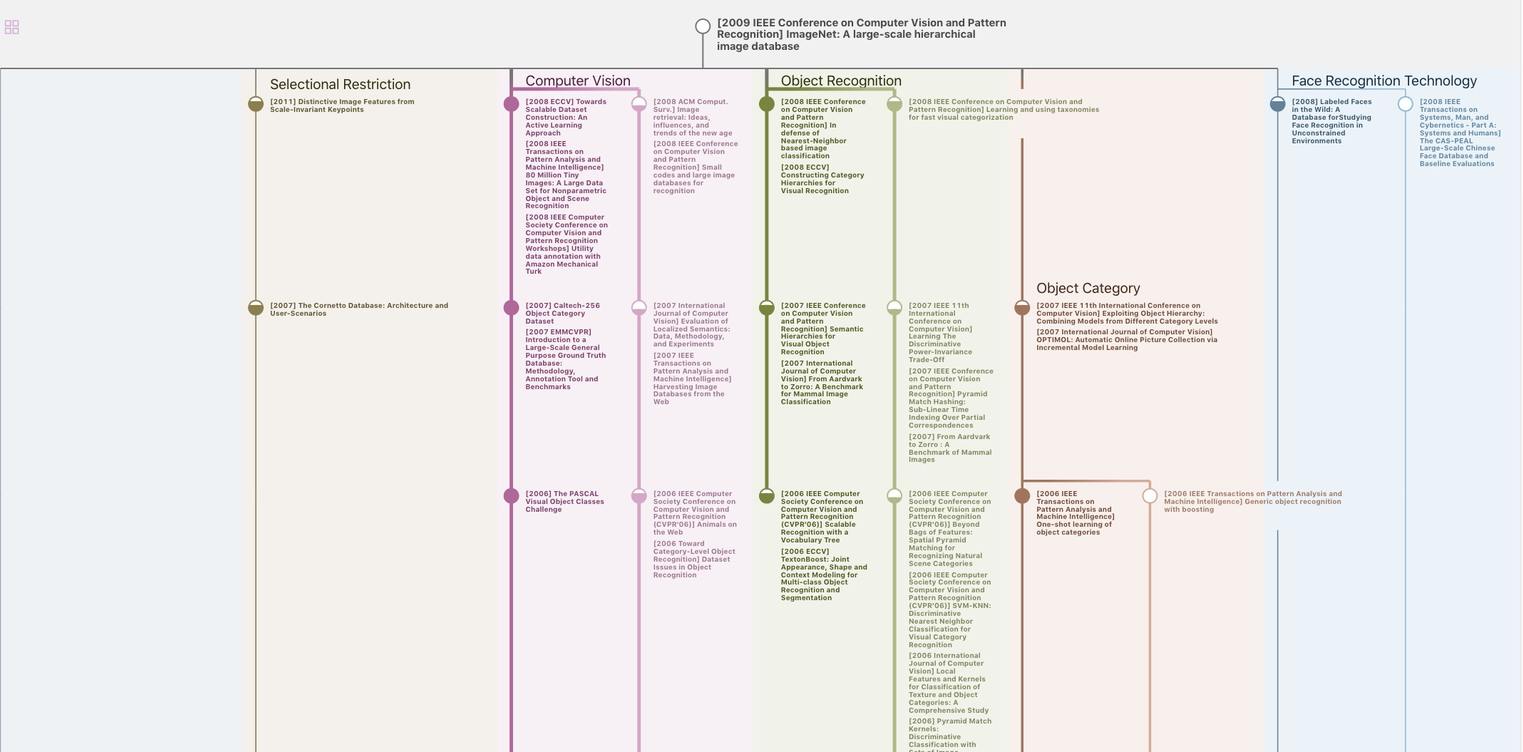
生成溯源树,研究论文发展脉络
Chat Paper
正在生成论文摘要