Improving the quality of chemical language model outcomes with atom-in-SMILES tokenization
J. Cheminformatics(2023)
摘要
Tokenization is an important preprocessing step in natural language processing that may have a significant influence on prediction quality. This research showed that the traditional SMILES tokenization has a certain limitation that results in tokens failing to reflect the true nature of molecules. To address this issue, we developed the atom-in-SMILES tokenization scheme that eliminates ambiguities in the generic nature of SMILES tokens. Our results in multiple chemical translation and molecular property prediction tasks demonstrate that proper tokenization has a significant impact on prediction quality. In terms of prediction accuracy and token degeneration, atom-in-SMILES is more effective method in generating higher-quality SMILES sequences from AI-based chemical models compared to other tokenization and representation schemes. We investigated the degrees of token degeneration of various schemes and analyzed their adverse effects on prediction quality. Additionally, token-level repetitions were quantified, and generated examples were incorporated for qualitative examination. We believe that the atom-in-SMILES tokenization has a great potential to be adopted by broad related scientific communities, as it provides chemically accurate, tailor-made tokens for molecular property prediction, chemical translation, and molecular generative models.
更多查看译文
关键词
Atom-in-SMILES,Tokenization,Repetition,Chemical language processing
AI 理解论文
溯源树
样例
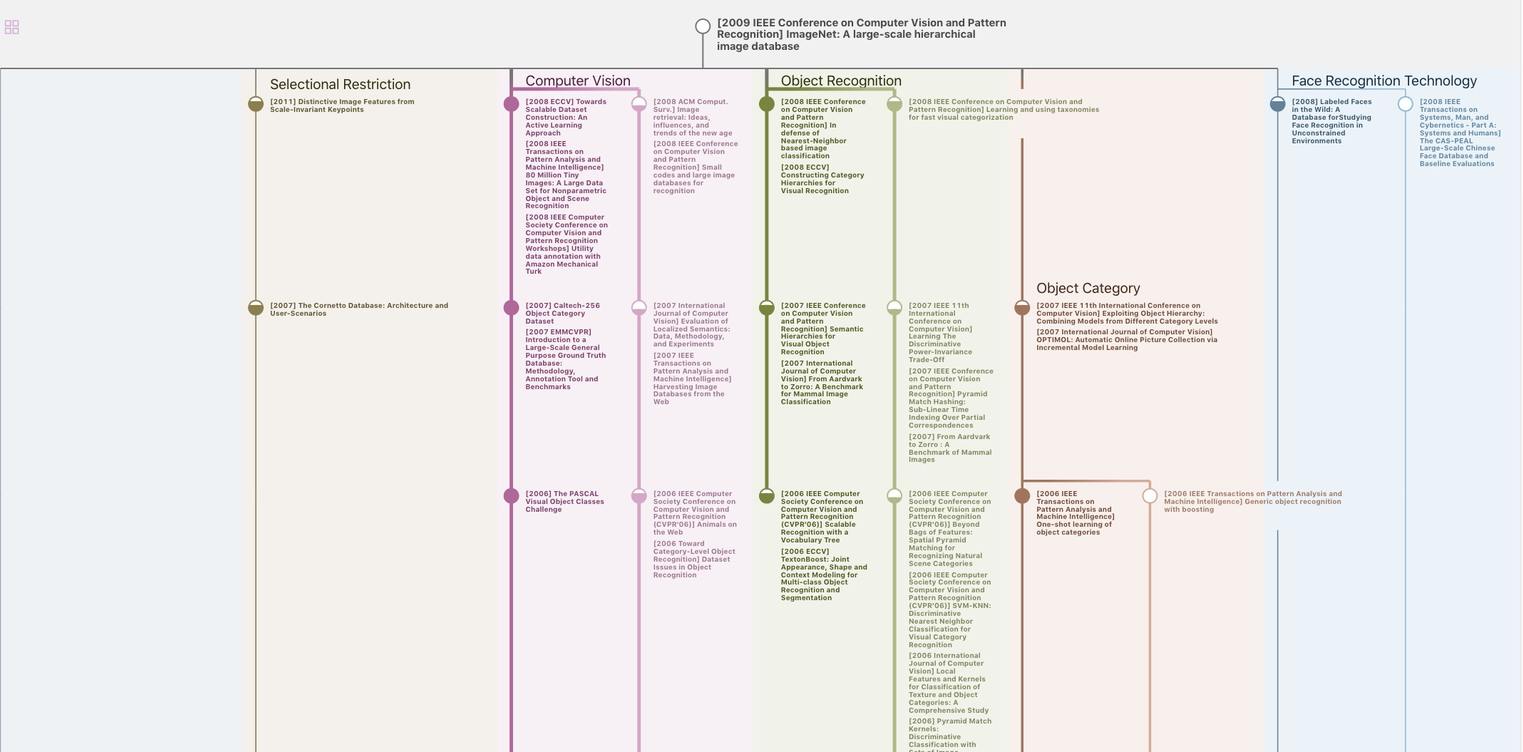
生成溯源树,研究论文发展脉络
Chat Paper
正在生成论文摘要