Threshold Attention Network for Semantic Segmentation of Remote Sensing Images.
IEEE Trans. Geosci. Remote. Sens.(2023)
摘要
Semantic segmentation of remote sensing images is essential for various applications, including vegetation monitoring, disaster management, and urban planning. Previous studies have demonstrated that the self-attention mechanism (SA) is an effective approach for designing segmentation networks that can capture long-range pixel dependencies. SA enables the network to model the global dependencies between the input features, resulting in improved segmentation outcomes. However, the high density of attentional feature maps used in this mechanism causes exponential increases in computational complexity. In addition, it introduces redundant information that negatively impacts the feature representation. Inspired by traditional threshold segmentation algorithms, we propose a novel threshold attention mechanism (TAM). This mechanism significantly reduces computational effort while also better modeling the correlation between different regions of the feature map. Based on TAM, we present a threshold attention network (TANet) for semantic segmentation. The TANet consists of an attentional feature enhancement module (AFEM) for global feature enhancement of shallow features and a threshold attention pyramid pooling (TAPP) module for acquiring feature information at different scales for deep features. We have conducted extensive experiments on the international society for photogrammetry and remote sensing (ISPRS) Vaihingen and Potsdam datasets. The results demonstrate the validity and superiority of our proposed TANet compared with most state-of-the-art models.
更多查看译文
关键词
remote sensing images,semantic segmentation,remote sensing,threshold,attention
AI 理解论文
溯源树
样例
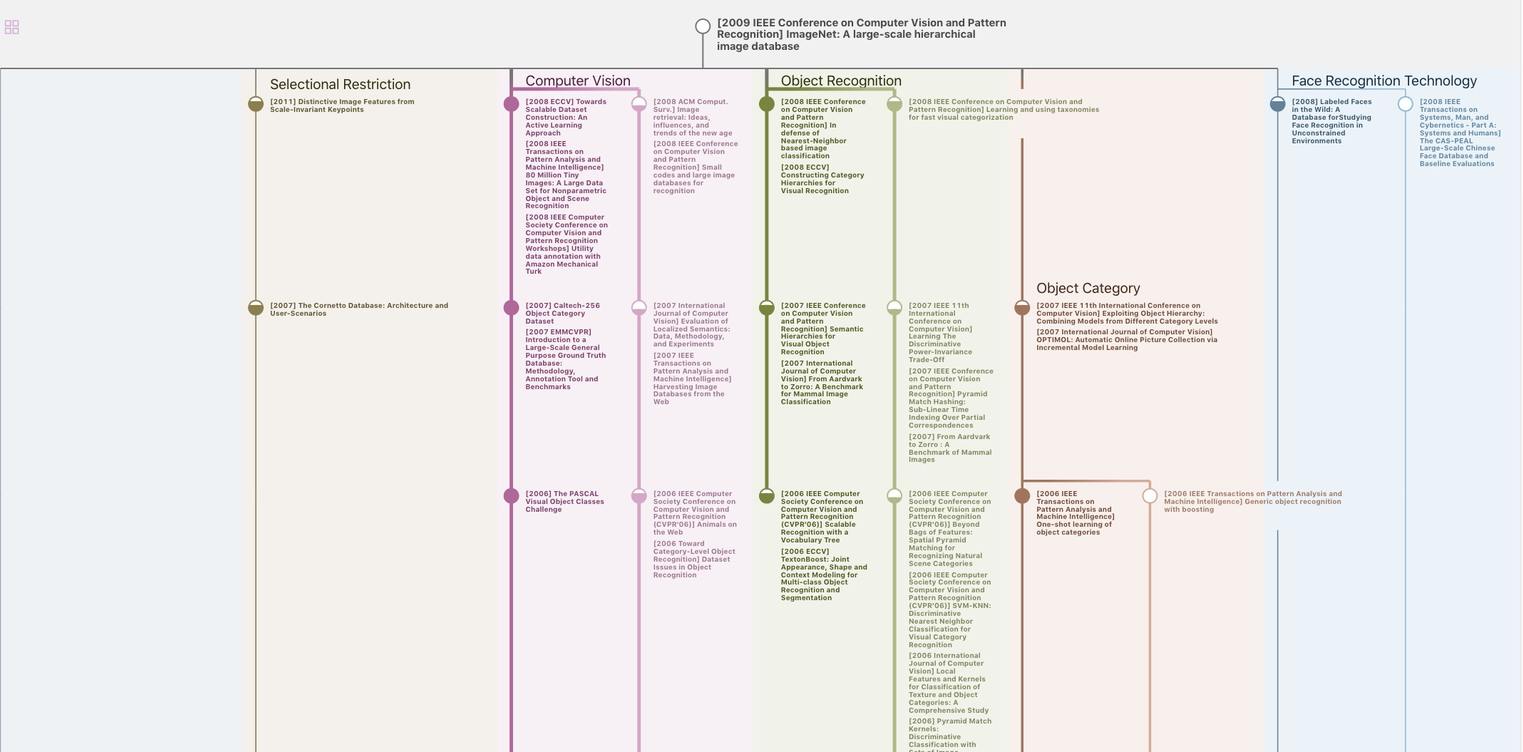
生成溯源树,研究论文发展脉络
Chat Paper
正在生成论文摘要