Deep Learning-Based RGB-D Fusion for Multimodal Condition Assessment of Civil Infrastructure.
J. Comput. Civ. Eng.(2023)
摘要
Recent advancements in the areas of computer vision and deep learning have broadened the scope of vision-based autonomous condition assessment of civil infrastructure. However, a review of available literature suggests that most of the existing vision-based inspection techniques rely only on color information, due to the immediate availability of inexpensive high-resolution cameras. Regular cameras translate a 3D scene to a 2D space, which leads to a loss of information vis-a-vis distance and scale. This imposes a barrier to the realization of the full potential of vision-based techniques. In this regard, the structural health monitoring community is yet to benefit from the new opportunities that commercially-available low-cost depth sensors offer. This study aims at filling this knowledge gap by incorporating depth fusion into an encoder-decoder-based semantic segmentation model. Advanced computer graphics approaches are exploited to generate a database of paired RGB and depth images representing various damage categories that are commonly observed in reinforced concrete (RC) buildings, namely, spalling, spalling with exposed rebars, and severely buckled rebars. A number of encoding techniques are explored for representing the depth data. Additionally, various schemes for the data-level, feature-level, and decision-level fusions of RGB and depth data are investigated to identify the best fusion strategy. Overall, it was observed that feature-level fusion is the most effective and can enhance the performance of deep learning-based damage segmentation algorithms by up to 25% without any appreciable increase in the computation time. Moreover, a novel volumetric damage quantification approach is introduced, which is robust against perspective distortion. This study is believed to advance the frontiers of infrastructure resilience and maintenance.
更多查看译文
关键词
multimodal condition assessment,civil infrastructure,fusion,learning-based
AI 理解论文
溯源树
样例
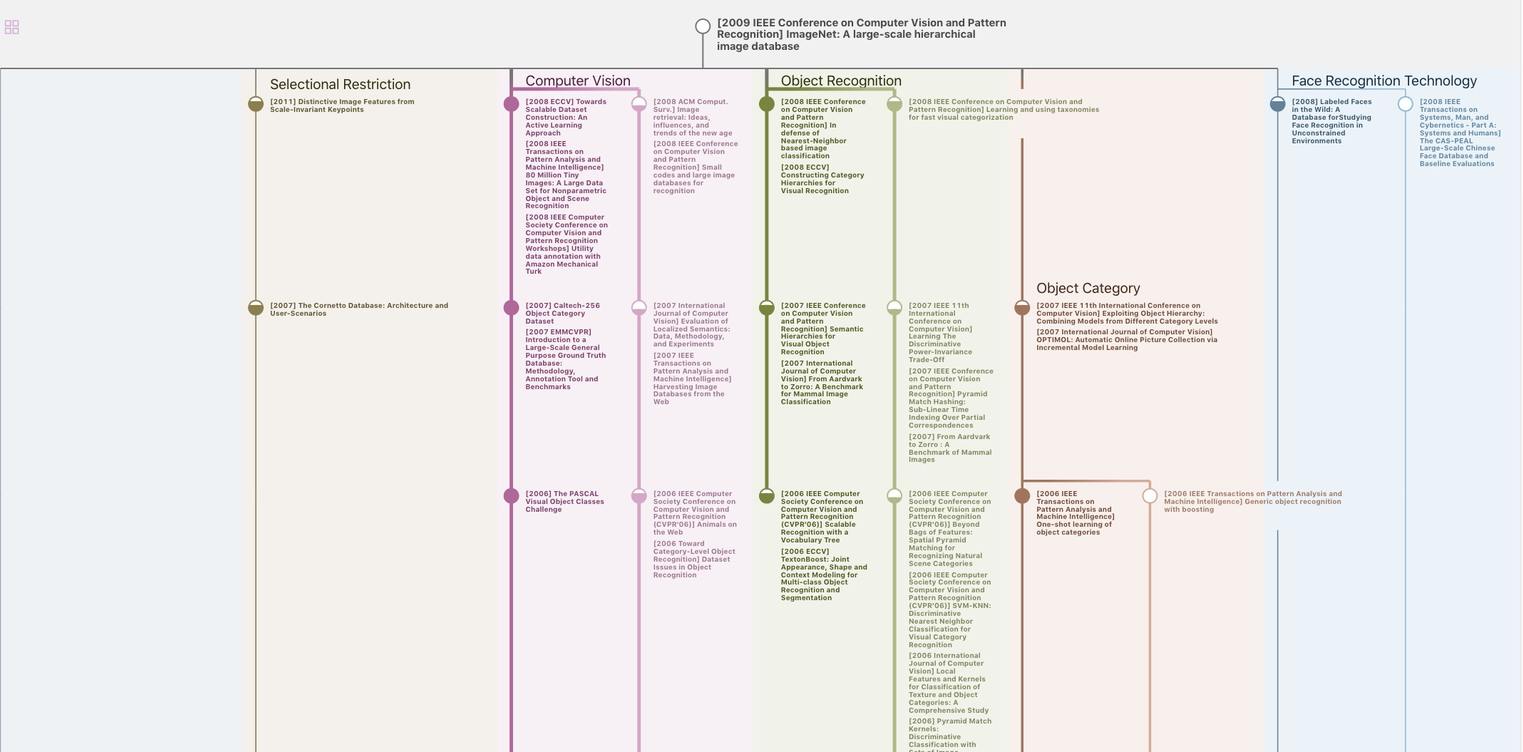
生成溯源树,研究论文发展脉络
Chat Paper
正在生成论文摘要