Z-number-valued rule-based decision trees.
Inf. Sci.(2023)
摘要
As a novel architecture of a fuzzy decision tree constructed on fuzzy rules, the fuzzy rule-based decision tree (FRDT) achieved better performance in terms of both classification accuracy and the size of the resulted decision tree than other classical decision trees such as C4.5, LADtree, BFtree, SimpleCart and NBTree. The concept of Z-number extends the classical fuzzy number to model both uncertain and partial reliable information. Z-numbers have significant potential in rule-based systems due to their strong representation capability. This paper designs a Z-number-valued rule-based decision tree (ZRDT) and provides the learning algorithm. Firstly, the information gain is used to replace the fuzzy confidence in FRDT to select features in each rule. Additionally, we use the negative samples to generate the second fuzzy numbers that adjust the first fuzzy numbers and improve the model's fit to the training data. The proposed ZRDT is compared with the FRDT with three different parameter values and two classical decision trees, PUBLIC and C4.5, and a decision tree ensemble method, AdaBoost.NC, in terms of classification effect and size of decision trees. Based on statistical tests, the proposed ZRDT has the highest classification performance with the smallest size for the produced decision tree.
更多查看译文
关键词
Z-numbers, Rule-based system, Decision trees, Z-number-valued rule, Classification, Information gain
AI 理解论文
溯源树
样例
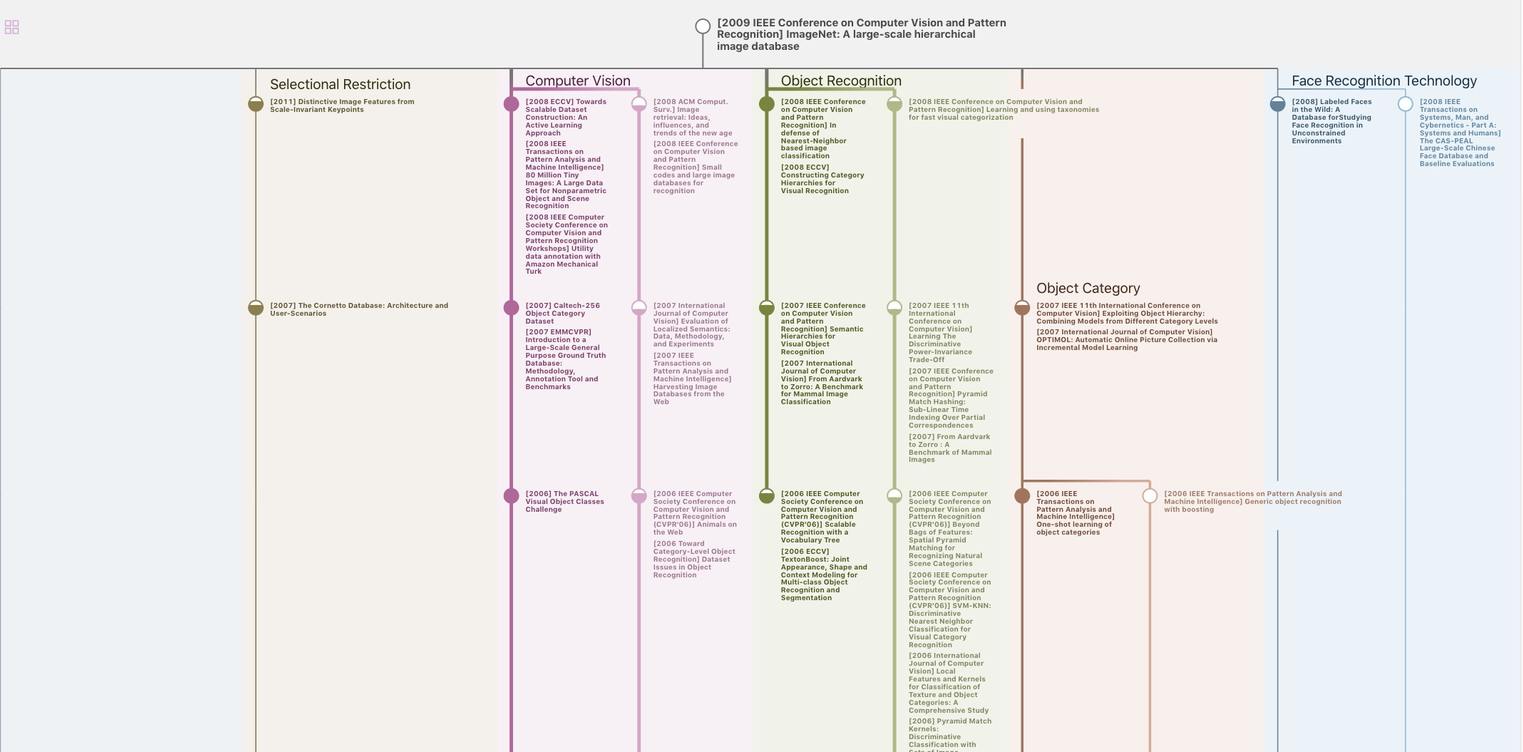
生成溯源树,研究论文发展脉络
Chat Paper
正在生成论文摘要