Incorporating multi-stage spatial visual cues and active localization offset for pancreas segmentation.
Pattern Recognit. Lett.(2023)
摘要
Accurately segmenting pancreas or pancreatic tumor from limited computed tomography (CT) scans plays an essential role in making a precise diagnosis and planning the surgical procedure for clinicians. Although deep convolutional neural networks (DCNNs) have greatly advanced in automatic organ segmentation, there are still many challenges in solving the pancreas segmentation problem with small region and complex background. Many researchers have developed a coarse-to-fine scheme, which employ prediction from the coarse stage as a smaller input region for the fine stage. Despite this scheme effectiveness, most existing approaches handle two stages individually, and fail to identify the reliability of coarse stage predictions. In this work, we present a novel coarse-to-fine framework based on spatial contextual cues and active localization offset. The novelty lies in carefully designed two modules: Spacial Visual Cues Fusion (SVCF) and Active Localization OffseT (ALOT). The SVCF combines the correlations between all pixels in an image to optimize the rough and uncertain pixel prediction at the coarse stage, while ALOT dynamically adjusts the localization as the coarse stage iteration. These two modules work together to optimize the coarse stage results and provide high-quality input for the fine stage, thereby achieving inspiring target segmentation. Empirical results on NIH pancreas segmentation and MSD pancreatic tumor segmentation dataset show that our framework yields state-of-the-art results. The code will make available at https://github.com/PinkGhost0812/SANet.
更多查看译文
关键词
pancreas,active localization,cues,multi-stage
AI 理解论文
溯源树
样例
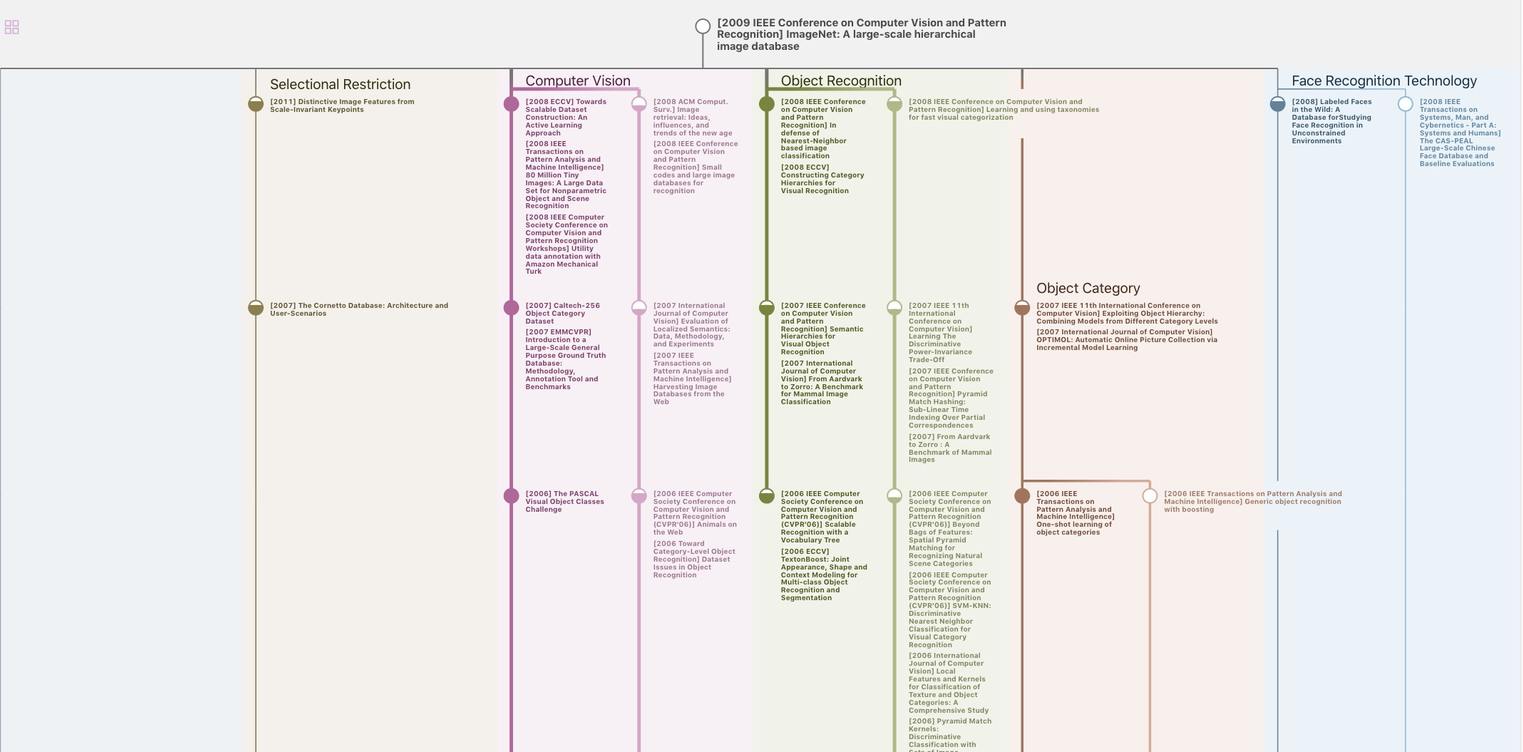
生成溯源树,研究论文发展脉络
Chat Paper
正在生成论文摘要