Procrustes Analysis on the Manifold of SPSD Matrices for Data Sets Alignment
IEEE Trans. Signal Process.(2023)
摘要
In contemporary high-dimensional data analysis, intrinsically similar and related data sets are often significantly different due to various undesired factors that could arise from different acquisition equipment, calibration, environmental conditions, and many other sources of batch effects. Therefore, the task of aligning such data sets has become ubiquitous. In this work, we present a method for the alignment of different, but related, sets of Symmetric Positive Semidefinite (SPSD) matrices, which constitute a commonly-used family of features, e.g., covariance and correlation matrices, various kernels, and prototypical graph and network representations. Our method does not require any a-priori correspondence, and it is based on non-Euclidean Procrustes Analysis (PA) using a particular Riemannian geometry of SPSD matrices. While the derivation is focused on the manifold of SPSD matrices, we show that our alignment method can be applied directly in the original high-dimensional data space, when considering SPSD features that are sample covariance matrices. We demonstrate the advantage of our approach over competing methods in simulations and in an application to Brain-Computer Interface (BCI) with electroencephalographic (EEG) recordings.
更多查看译文
关键词
Manifolds,Covariance matrices,Geometry,Recording,Symmetric matrices,Electroencephalography,Task analysis,Domain adaptation,symmetric positive semidefinite matrices,Riemannian geometry,BCI,EEG
AI 理解论文
溯源树
样例
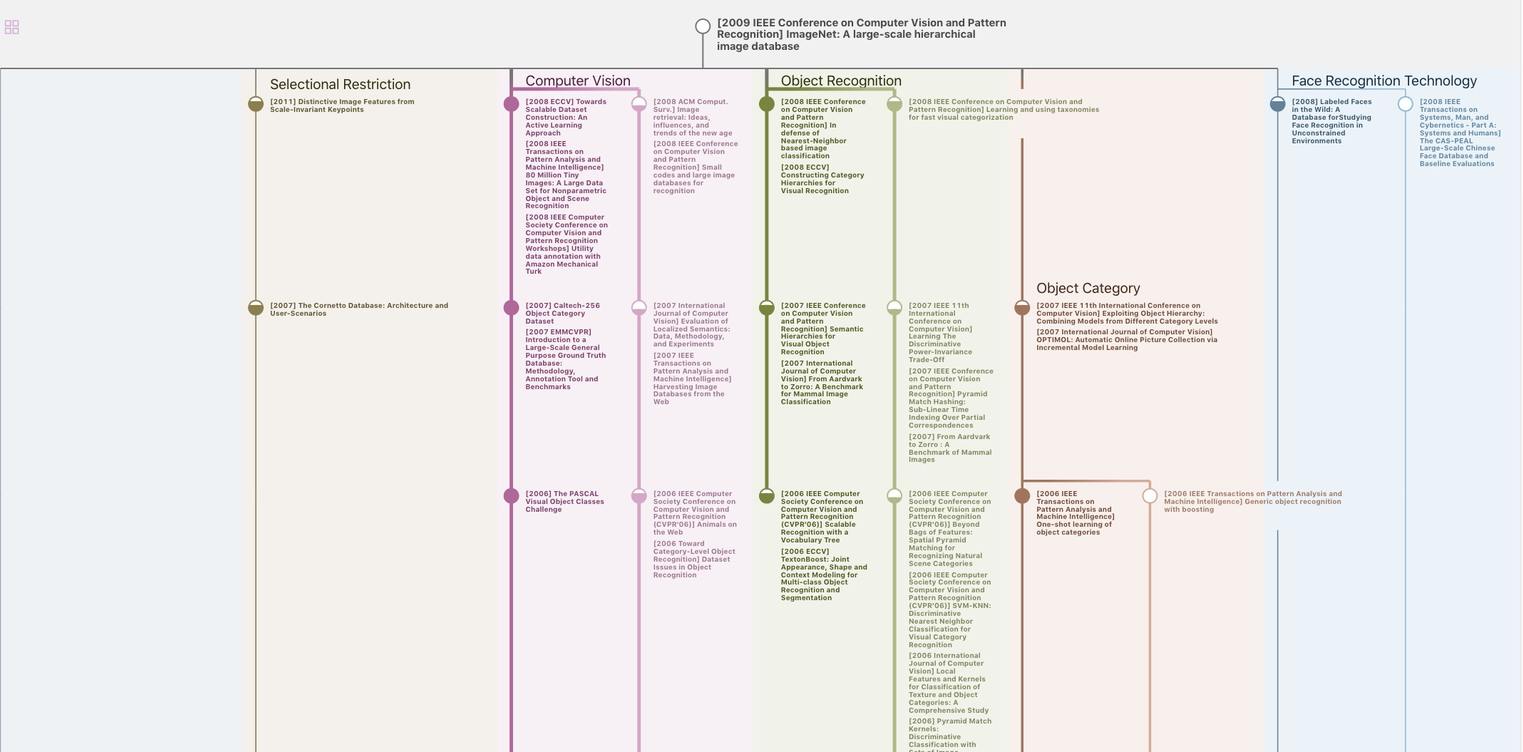
生成溯源树,研究论文发展脉络
Chat Paper
正在生成论文摘要