Safety AARL: Weight adjustment for reinforcement-learning-based safety dynamic asset allocation strategies
Expert Syst. Appl.(2023)
摘要
Dynamic asset allocation involves adjusting asset weights based on performance to reduce risks according to market conditions. This paper proposes a novel framework called safety asset allocation reinforcement learning (AARL), which combines multiple protective dynamic asset allocation strategies (PDAS) to minimize risks through reinforcement learning (RL). The framework integrates eight validated PDAS that maintain their unique characteristics. To implement the proposed framework, a novel RL environment and agent were developed to invest in PDAS using three high-probability actions. The agent receives state and reward information from the environment and is trained using the proximal policy optimization algorithm to output weights for investing. The actions are PDAS, which facilitates for diversified investments and the learning of various combinations that are suitable for market conditions. Three experiments were conducted to verify the effectiveness of the proposed Safety AARL framework. The first experiment demonstrates the validity of adjusting weights using the frame-work. The second experiment demonstrates that the framework outperforms existing techniques during global market decline. The third experiment demonstrates that risk is minimized, outperforming the latest methods for asset allocation. Overall, the results demonstrate that the Safety AARL framework outperforms existing tech-niques by minimizing risks and maintaining stable returns by combining the advantages of multiple PDAS.
更多查看译文
关键词
Reinforcement learning,Dynamic asset allocation,Proximal policy optimization,Weight adjustment,Stock trading
AI 理解论文
溯源树
样例
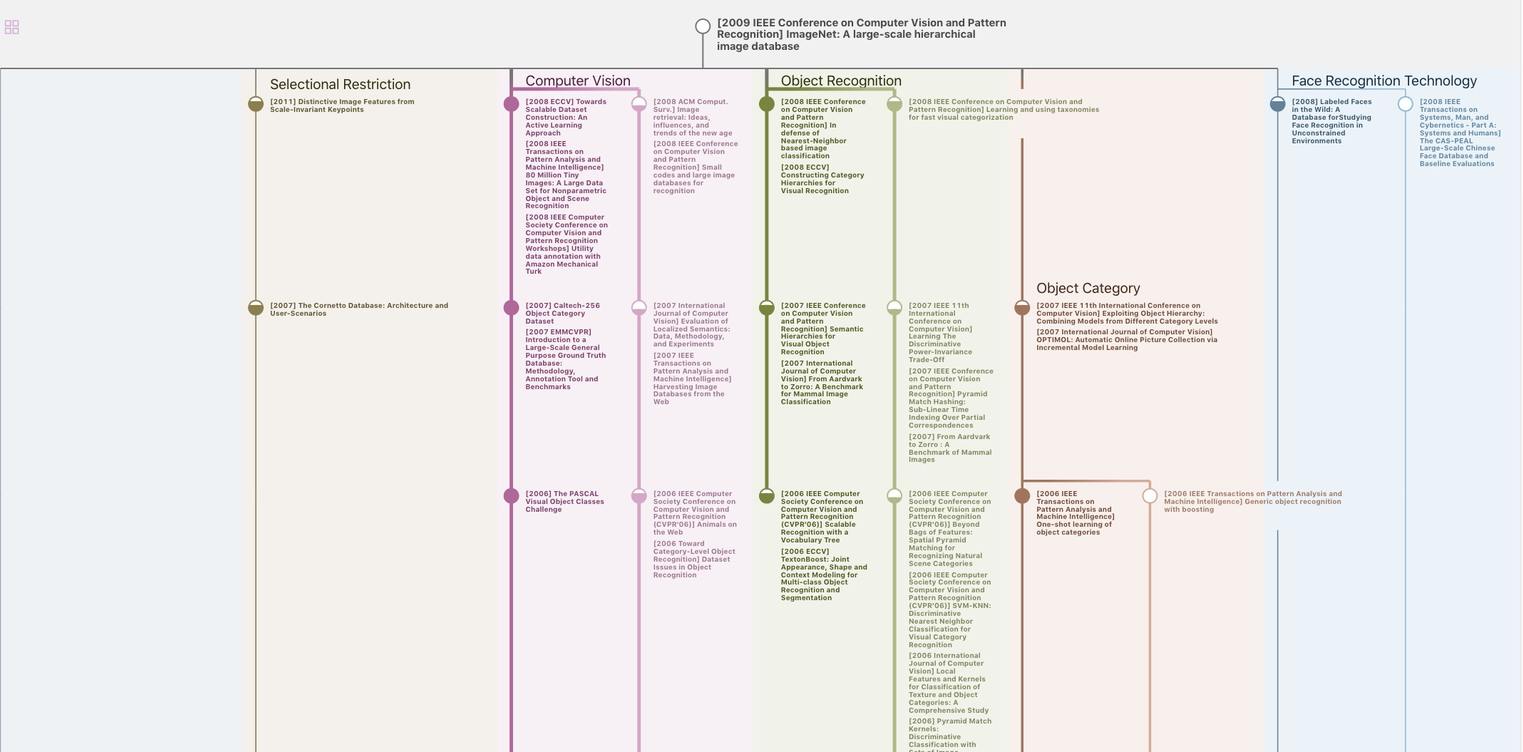
生成溯源树,研究论文发展脉络
Chat Paper
正在生成论文摘要