ENGA: Elastic Net-Based Genetic Algorithm for human action recognition.
Expert Syst. Appl.(2023)
摘要
Video surveillance and activity monitoring are the practical real-time applications of Human Action Recognition (HAR). A fusion of several Convolutional Neural Network (CNN) architectures has been widely used for effective HAR and achieved impressive results. Feature fusion of multiple pre-trained models also extracts redundant features due to the combinations of identical layers in all CNN architectures. In this study, network-level fusion is proposed, which reduces the possibility of having identical layers throughout the fusion process and helps extract unique features. Three pre-trained models, i.e., NASNetLarge, DenseNet201, and DarkNet53 are selected and analyzed to select the most efficient combinations of layers among these networks. Selected combinations of these networks are fused using five proposed strategies, i.e., sum, max, concatenation, convolutional and bilinear fusion. In the end, a proposed minimized CNN architecture is utilized to extract descriptors, which are optimized using the proposed Elastic Net-based Genetic Algorithm (ENGA) approach. A two-phase hybrid ENGA technique is suggested to pick features using both GA and EN. GA is used in the initial stage to reduce the dimensionality of retrieved features. To eliminate the unnecessary features, EN regularization is put into place in the second phase. The proposed ENGA model is evaluated on four publicly available datasets including UTKinect-Action, MSR-Action3D dataset, Florence3D-Action dataset, and Youtube-8 m, and achieved 99.63%, 99.69%. 98.63% and 91.46% accuracies, respectively.
更多查看译文
关键词
Network Level Fusion, Genetic Algorithm, Elastic Net, Feature Fusion, CNN, Transfer-learning
AI 理解论文
溯源树
样例
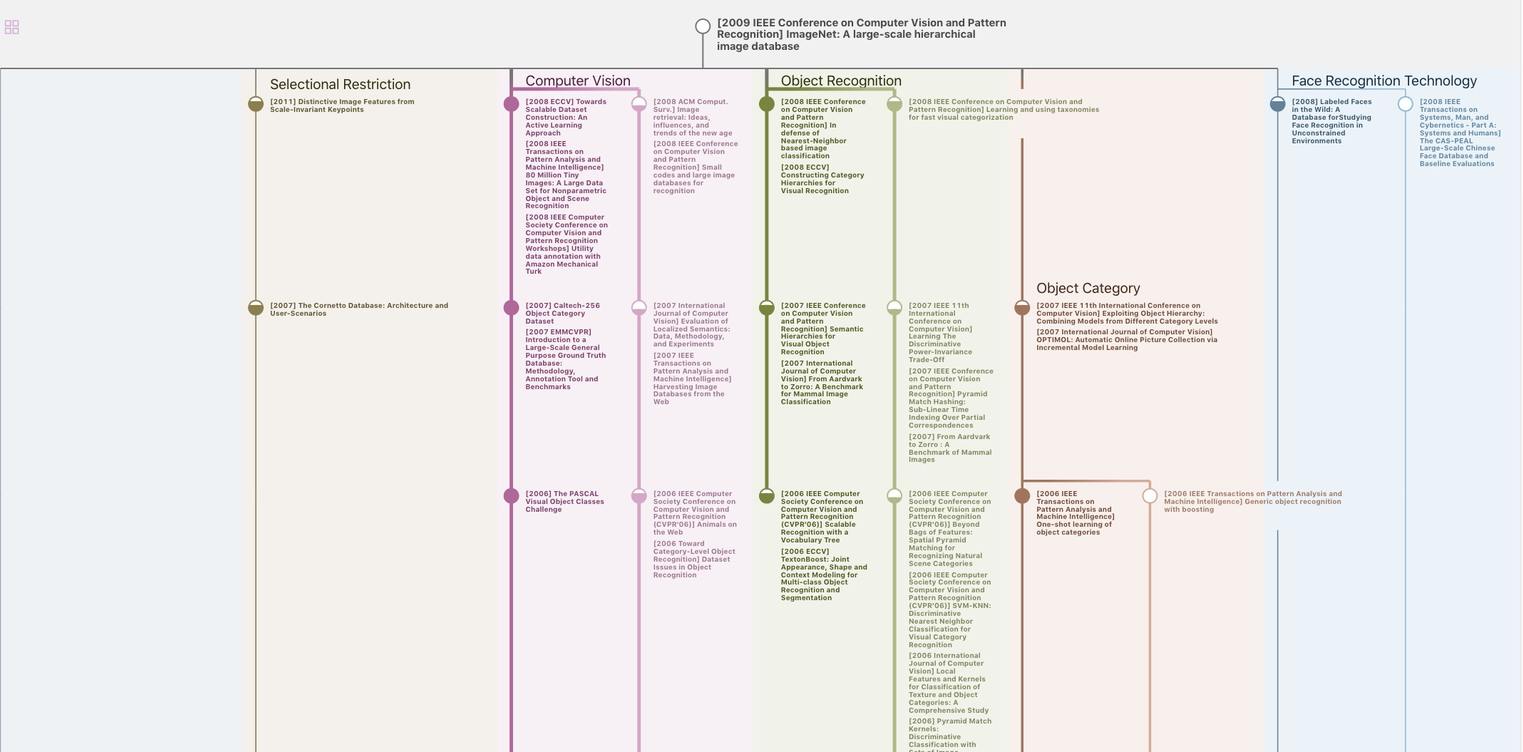
生成溯源树,研究论文发展脉络
Chat Paper
正在生成论文摘要