Cross-domain endoscopic image translation and landmark detection based on consistency regularization cycle generative adversarial network.
Expert Syst. Appl.(2023)
摘要
The mitral valve simulator provides an important training scenario for mitral valve suture repair surgery. However, since there are differences between simulator scenarios and intra-operative scenarios, trainers may not adapt well to real surgeries. To enhance the realism of simulator scenarios, generative adversarial network (GAN) based methods can transform simulator endoscopic images into intra-operative style. Nonetheless, due to the variable number of suture landmarks and their unclear structural relationship in endoscopic images, maintaining content consistency after transformation becomes a challenging task. To address this issue, we present an improved consistency regularization cycle generative adversarial method (RegCycleGAN) in this paper. RegCycleGAN jointly optimizes CycleGAN and equivalent perturbation landmark detectors, considering that the location of suture landmarks could provide quantitative information when analyzing the consistency of image content. RegCycleGAN is capable of generating pseudo-images with consistent content and detecting suture landmarks on endoscopic images more accurately. We use a student–teacher network with exponential moving average (EMA) to provide generators with more consistent suture landmarks information. Additionally, we have developed an equivalent perturbation transformation module to improve the training stability of landmark detectors. Our method has demonstrated superior performance in image generation and landmark detection on two test datasets from the AdaptOR Challenge. The final results demonstrate that our method increases the value of F1 from 42.828% to 47.020% and from 48.776% to 50.401% on the two test set respectively. It indicates that RegCycleGAN provides a competitive performance in comparison to the state-of-the-art image translation methods in endoscopic image-to-image translation and landmark detection tasks.
更多查看译文
关键词
landmark detection,generative adversarial network,consistency regularization cycle,cross-domain
AI 理解论文
溯源树
样例
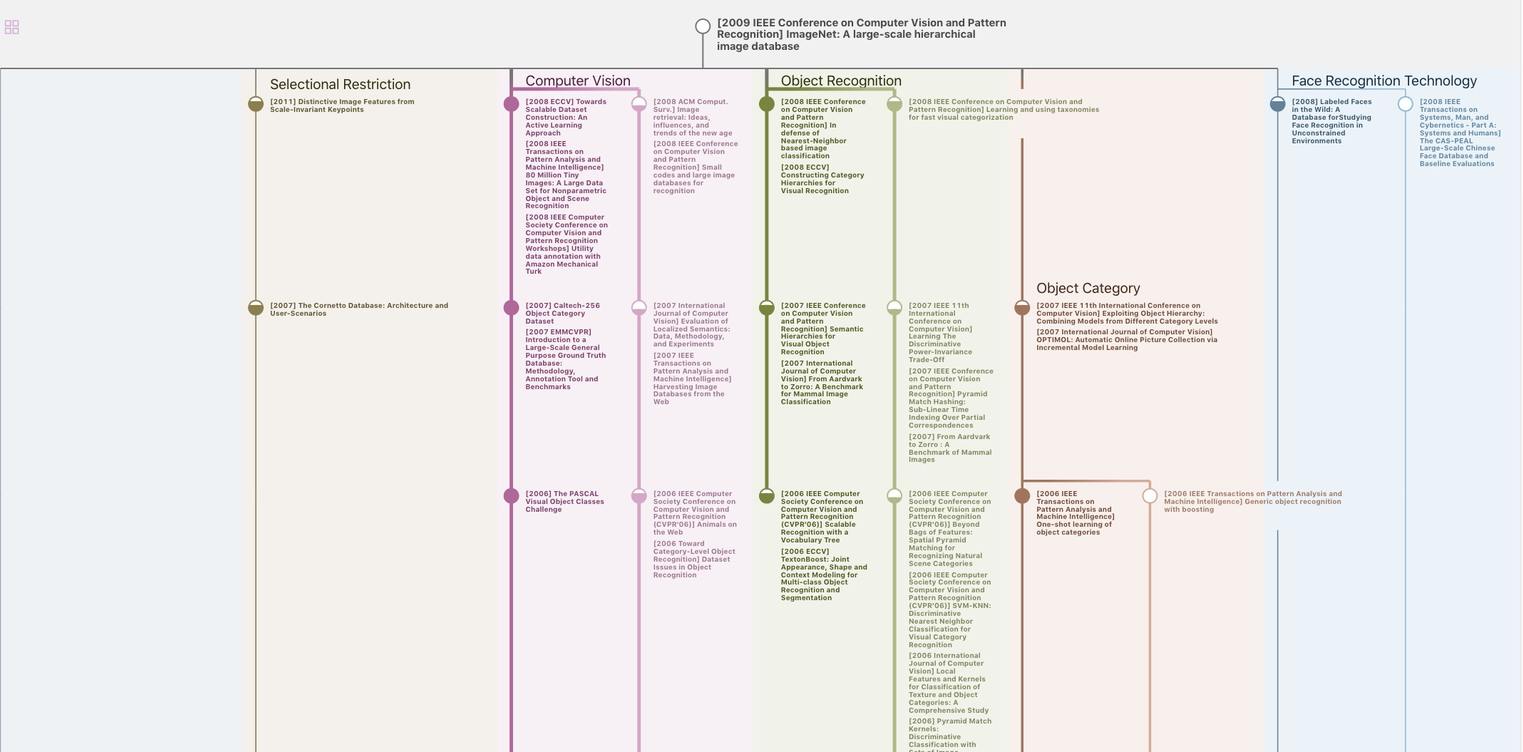
生成溯源树,研究论文发展脉络
Chat Paper
正在生成论文摘要