A Physiological Control System for Pulsatile Ventricular Assist Device Using an Energy-Efficient Deep Reinforcement Learning Method
IEEE Trans. Instrum. Meas.(2023)
摘要
Pulsatile ventricular assist device (PVAD) is a blood pump used to assist the circulation support of the native heart. Because of patients' complex physiological environment, PVAD's control system requires high adaptive ability and low computational energy. However, traditional PID controllers do not possess sufficient adaptive ability. Although neural network controllers are with high adaptive ability, their extensive energy cost limits the applications. In this study, a PVAD physiological control system based on deep reinforcement learning (DRL) is proposed, which significantly improves the system's adaptive ability. To further reduce its energy cost, a new energy-efficient DRL method, AddTD3, is developed, in which the fully connected network (FC) with high computation complexity is replaced by energy-efficient AdderNet. Experimental results show that the proposed AddTD3 controller is with higher adaptive ability than the traditional PID controller (cumulative absolute error: 237.1 versus 484.3 mmHg) and can be migrated to the mock circulation system (MCS) without fine-tuning. It can reduce the energy cost of the traditional DRL algorithm TD3 to 44.8% without reducing the performance (8773.2 versus 22420.4 pJ).
更多查看译文
关键词
Adder neural network,deep reinforcement learning (DRL),heart failure,physiological control,pulsatile ventricular assist device (PVAD)
AI 理解论文
溯源树
样例
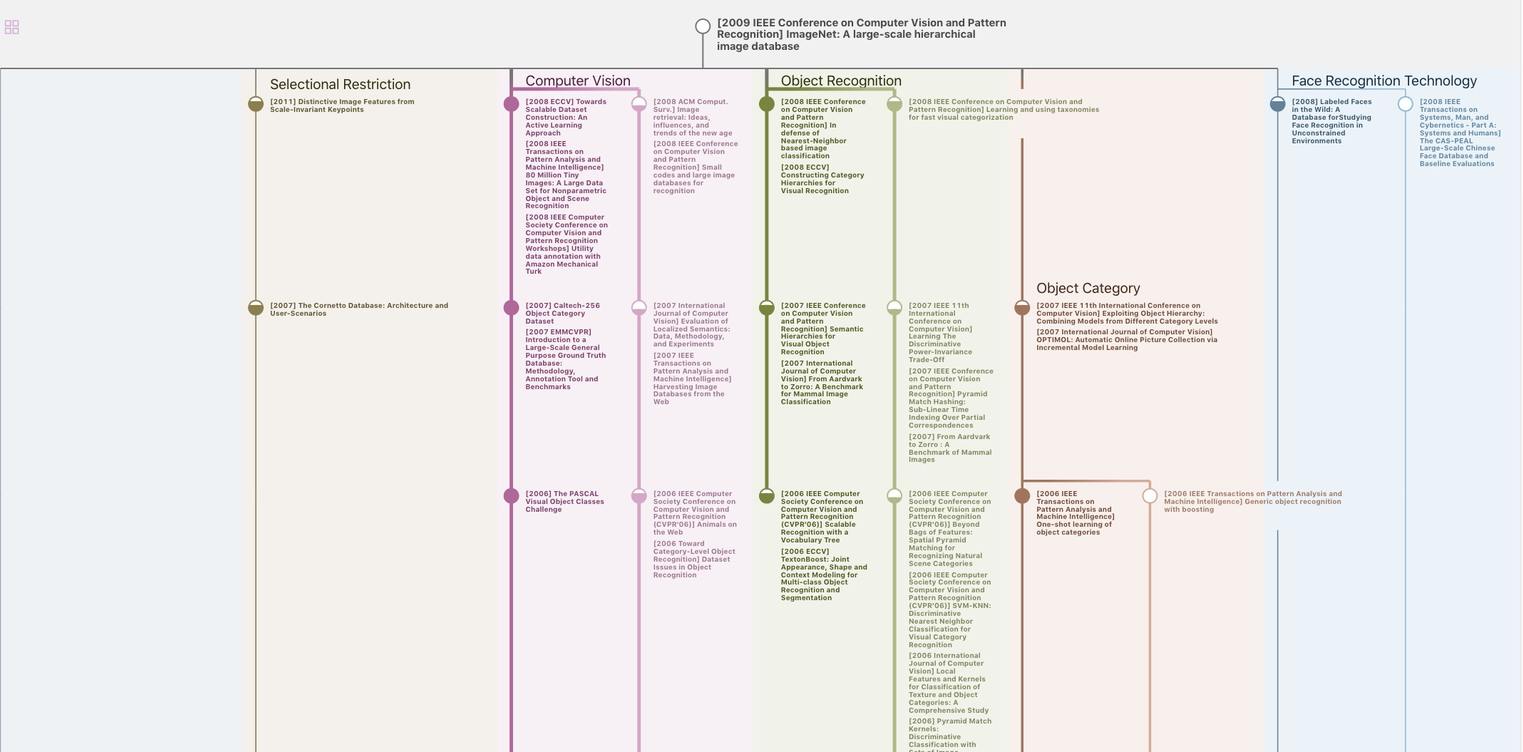
生成溯源树,研究论文发展脉络
Chat Paper
正在生成论文摘要