Efficient Periodicity Analysis for Real-Time Anomaly Detection
NOMS(2023)
摘要
The analysis of time series data is critical for the effective management of IT infrastructures, with real-time anomaly detection being of particular importance. A crucial component in any anomaly detection pipeline is the characterization of individual data streams so that the most appropriate algorithm can be deployed on a case-by-case basis. Periodicity is a characteristic that directly impacts the choice of algorithm; efficient identification of its presence, or absence, is essential. In the current paper, we develop a periodicity detector based on the Lomb-Scargle periodogram and analyze its performance when exposed to varying quantities of data. Our analysis uses the well-known F1-score to identify the lower limit on the data volume required for maximal detector performance. We then extend our periodicity detection method to include a statistical analysis based on the Wilson score to establish confidence in the detector result. We demonstrate that in the absence of ground-truth labels, our method can quantity the minimum amount of data and the minimum number of trials required to correctly, and definitively, identify periodicity within a data stream.
更多查看译文
关键词
case-by-case basis,data stream,individual data streams,IT infrastructure management,Lomb-Scargle periodogram,maximal detector performance,periodicity analysis efficiency,periodicity detector,real-time anomaly detection,statistical analysis,time series data analysis,Wilson score
AI 理解论文
溯源树
样例
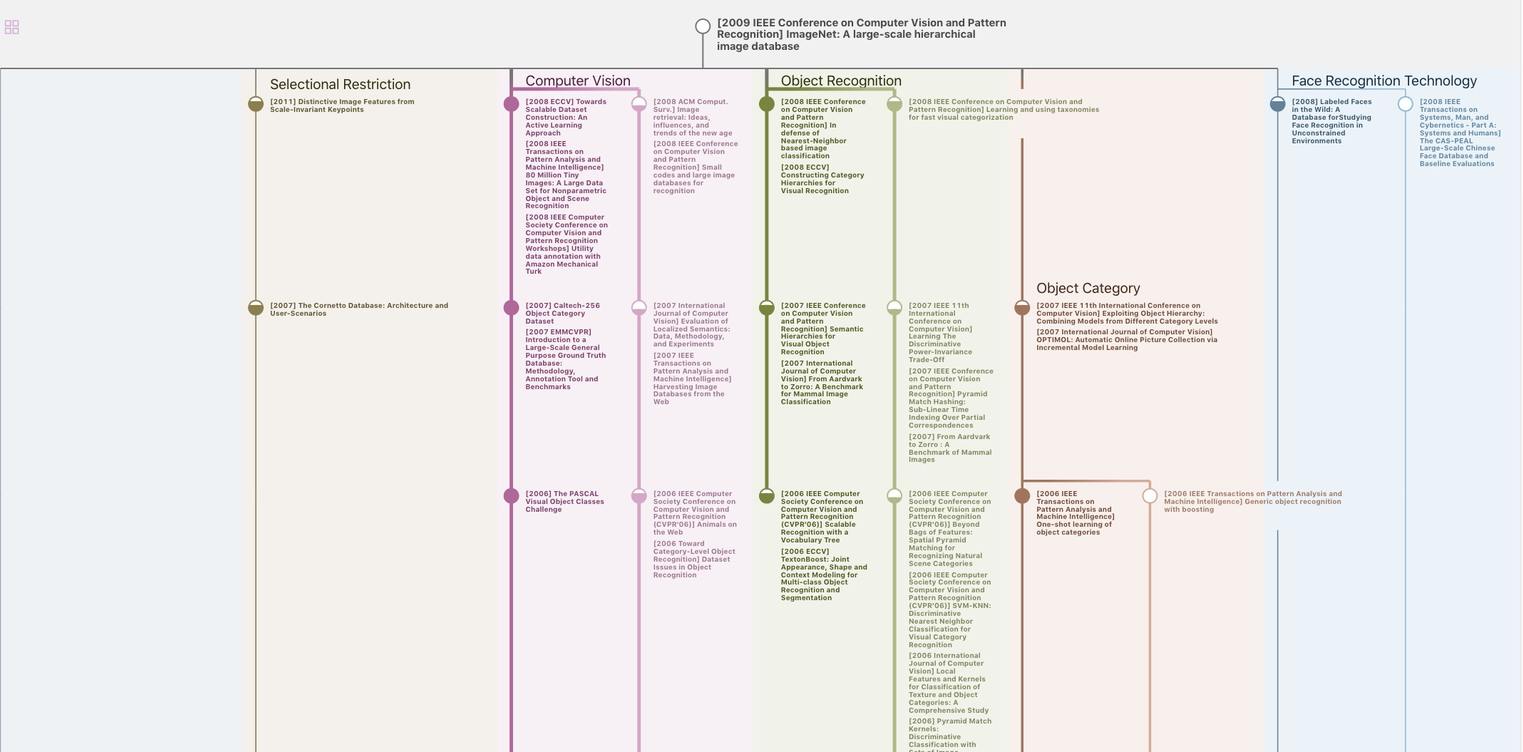
生成溯源树,研究论文发展脉络
Chat Paper
正在生成论文摘要