Local scale surface temperature estimation by downscaling satellite thermal infrared observations using neural networks.
JURSE(2023)
摘要
Land Surface Temperature (LST) is an important parameter for studying various phenomena in the urban environment, especially at a high spatial resolution. However, the sensors that provide high resolution (order of 100 m) LST do not frequently provide data (best case twice a month), and the ones that frequently provide data (daily) have a low spatial resolution (order of 1 km). Although, new thermal sensor satellite missions are planned with improved spatial and temporal resolution in the coming years, from space agencies around the world, exploiting existing and past missions for high spatio-temporal LST retrieval is important for many scientific fields, including urban climate. Resolving the diurnal variation of LST over cities remains a challenge and satellite data remain the most valuable source of information for large spatial coverage. In this paper a neural network was constructed to estimate high resolution LST by downscaling low resolution thermal infrared (TIR) imagery from MODIS. Besides TIR imagery, land cover fractions were given as input to the network, along with sky view factor and water vapor (also from MODIS), and the ASTER LST product was used to train it. The network was trained and evaluated on two different dates on London, and achieved a mean absolute error of 1.93 K.
更多查看译文
关键词
Land Surface Temperature (LST),Thermal Infrared,Downscaling,Sky View Factor,Neural Network
AI 理解论文
溯源树
样例
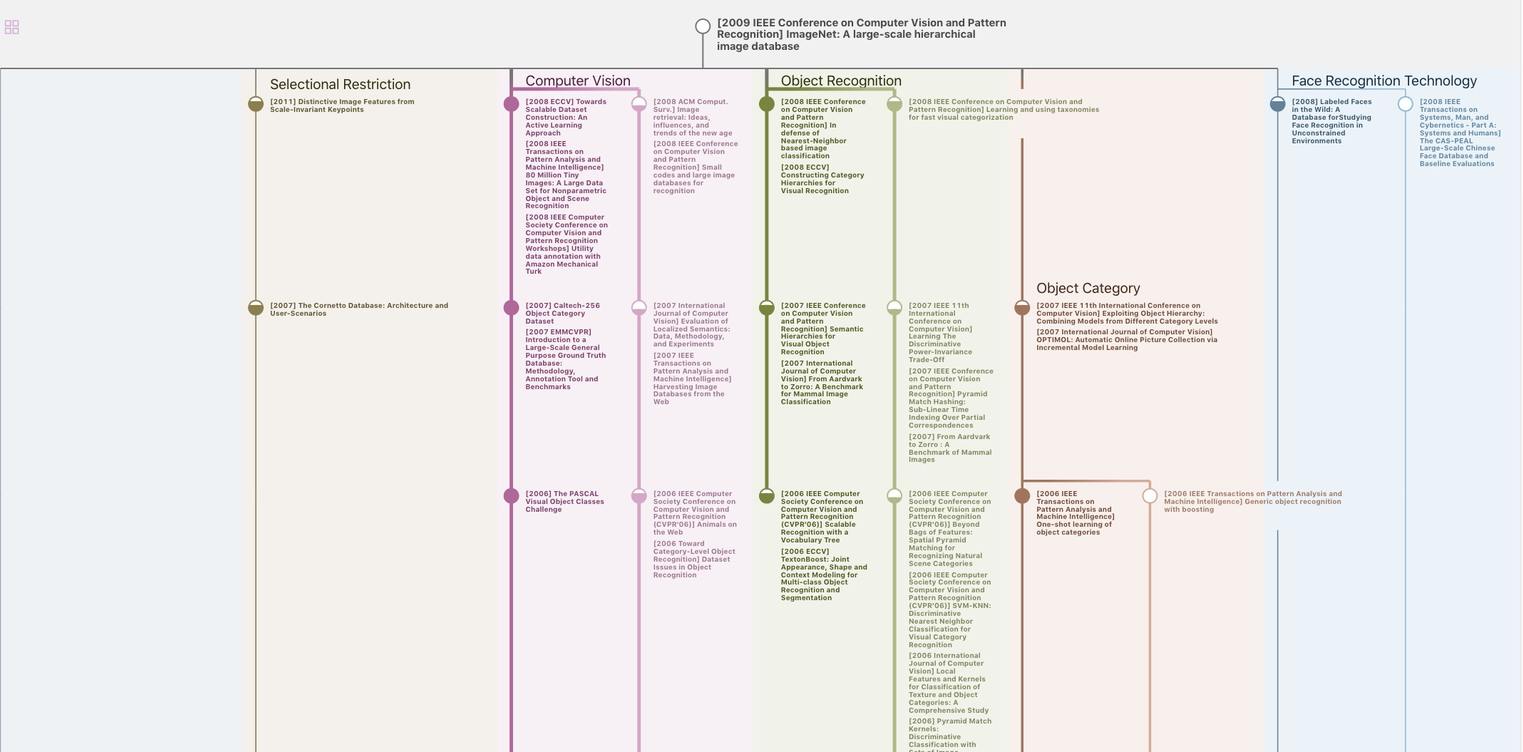
生成溯源树,研究论文发展脉络
Chat Paper
正在生成论文摘要