Balancing Federated Learning Trade-Offs for Heterogeneous Environments.
PerCom Workshops(2023)
摘要
Federated Learning (FL) is an enabling technology for supporting distributed machine learning across several de-vices on decentralized data. A critical challenge when FL in practice is the system resource heterogeneity of worker devices that train the ML model locally. FL workflows can be run across diverse computing devices, from sensors to High Performance Computing (HPC) clusters; however, these resource disparities may result in some devices being too burdened by the task of training and thus struggle to perform robust training when compared to more high-power devices (or clusters). Techniques can be applied to reduce the cost of training on low-power devices, such as reducing the number of epochs to perform during training. However, such techniques may also negatively harm the performance of the locally-trained model, introducing a resource-model performance trade-off. In this work, we perform robust experimentation with the aim of balancing this resource-model performance trade-off in FL. Our results provide intuition for how training hyper-parameters can be tuned to improve this trade-off in FL.
更多查看译文
关键词
Federated Learning,Heterogeneous Computing,Serverless Computing,Trade-offs
AI 理解论文
溯源树
样例
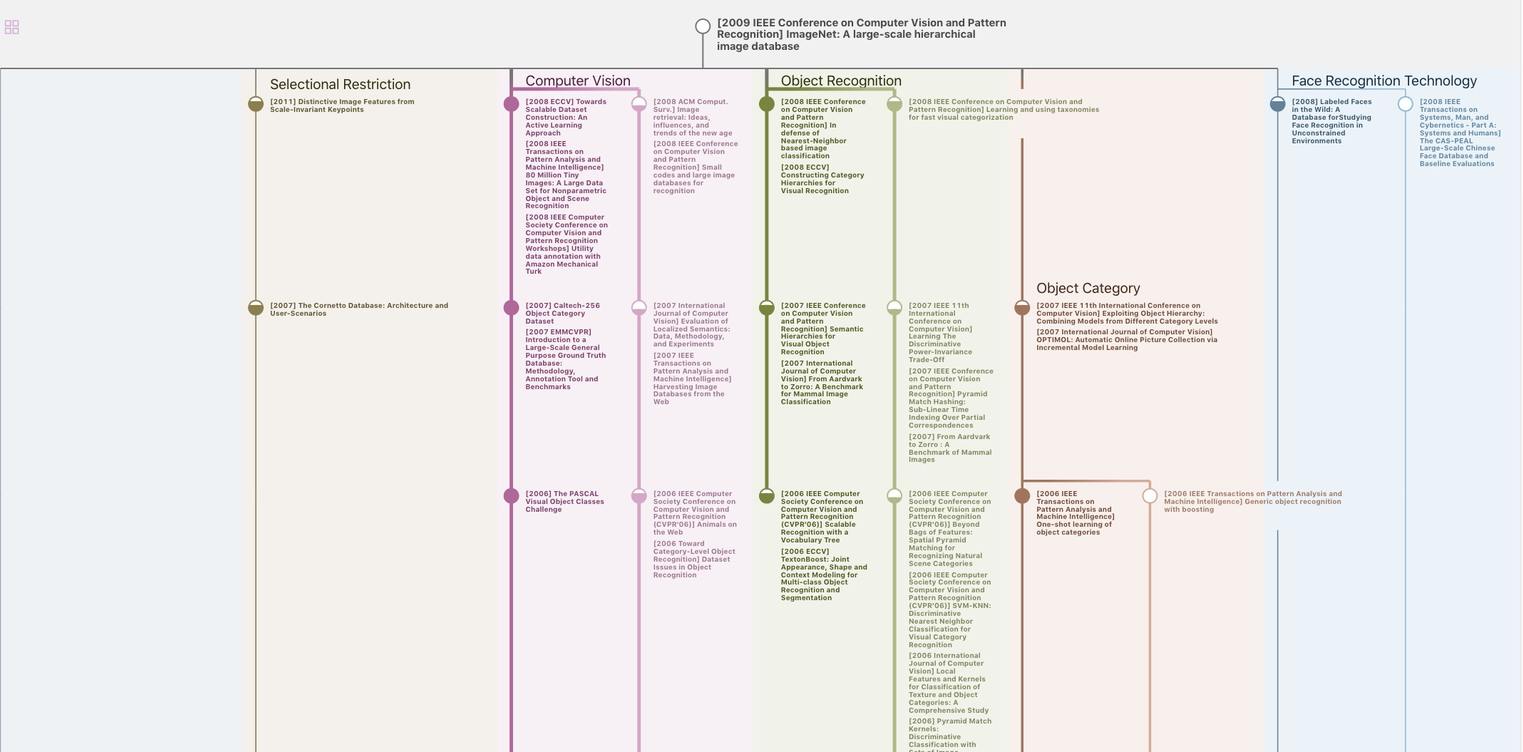
生成溯源树,研究论文发展脉络
Chat Paper
正在生成论文摘要