Reducing the Cost: Cross-Prompt Pre-finetuning for Short Answer Scoring.
AIED(2023)
摘要
Automated Short Answer Scoring (SAS) is the task of automatically scoring a given input to a prompt based on rubrics and reference answers. Although SAS is useful in real-world applications, both rubrics and reference answers differ between prompts, thus requiring a need to acquire new data and train a model for each new prompt. Such requirements are costly, especially for schools and online courses where resources are limited and only a few prompts are used. In this work, we attempt to reduce this cost through a two-phase approach: train a model on existing rubrics and answers with gold score signals and finetune it on a new prompt. Specifically, given that scoring rubrics and reference answers differ for each prompt, we utilize key phrases, or representative expressions that the answer should contain to increase scores, and train a SAS model to learn the relationship between key phrases and answers using already annotated prompts (i.e., cross-prompts). Our experimental results show that finetuning on existing cross-prompt data with key phrases significantly improves scoring accuracy, especially when the training data is limited. Finally, our extensive analysis shows that it is crucial to design the model so that it can learn the task’s general property. We publicly release our code and all of the experimental settings for reproducing our results ( https://github.com/hiro819/Reducing-the-cost-cross-prompt-prefinetuning-for-SAS ).
更多查看译文
关键词
short answer scoring,cross-prompt,pre-finetuning
AI 理解论文
溯源树
样例
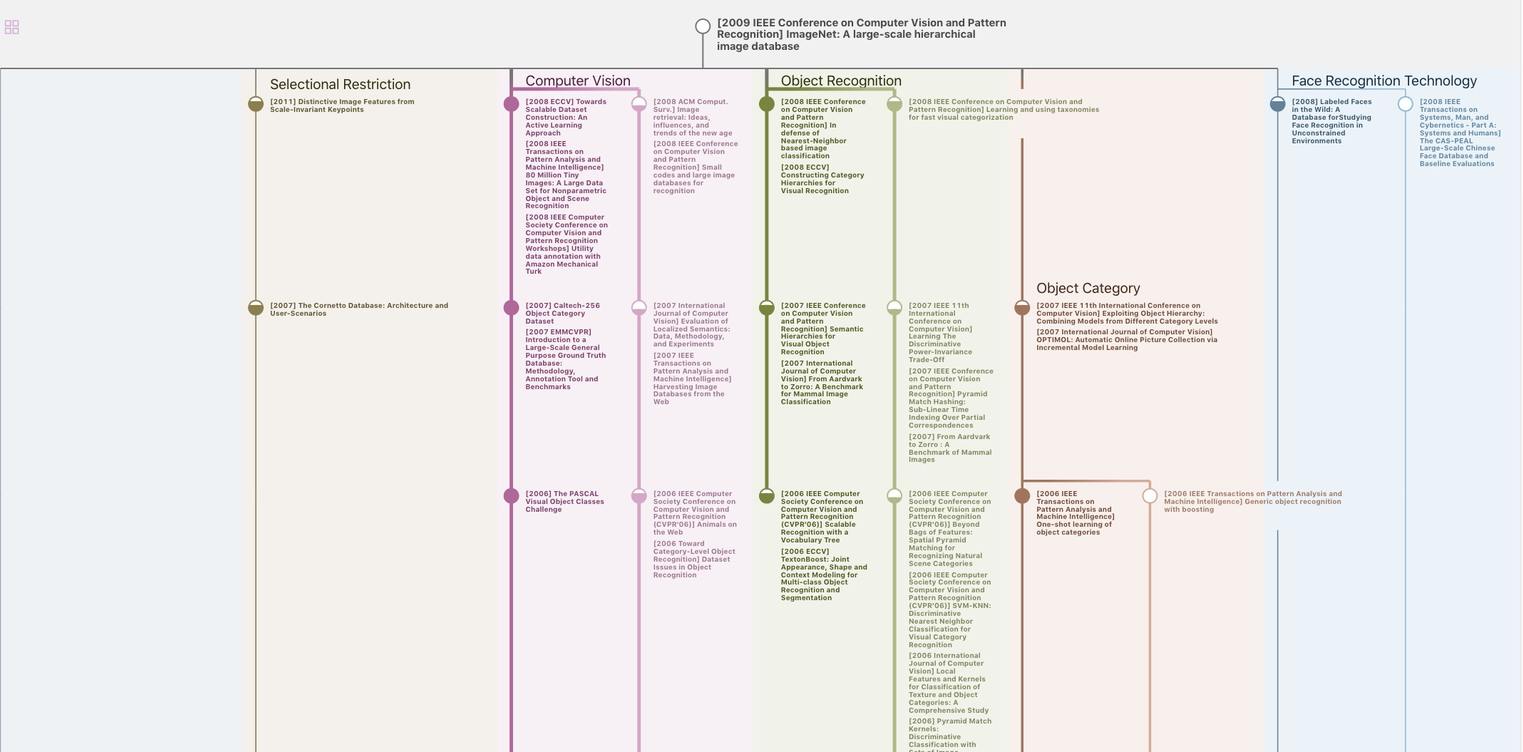
生成溯源树,研究论文发展脉络
Chat Paper
正在生成论文摘要