Comparing Sampling Strategies for the Classification of Bi-objective Problems by FLACCO Features.
CISIS(2023)
摘要
Problem understanding and the ability to assign problems to distinct classes can improve the usability of metaheuristics. A popular problem-independent method for the characterization of optimization problems is exploratory landscape analysis (ELA). It consists of a sequence of operations that describe the hypersurfaces formed by fitness and other characteristic properties of the problem solutions on the basis of a limited number of samples. Sampling is the initial step of ELA that selects a limited number of candidate solutions for which are the characteristic properties evaluated. The solutions and the computed properties serve as the main inputs for the rest of ELA. In this work, we study the impact of different sampling strategies on machine learning-based classification of bi-objective problems on the basis of FLACCO features. A series of computational experiments demonstrates that different sampling strategies affect the value of the resulting landscape features, their suitability for problem classification, and the overhead of the sampling process. An in-depth analysis of the results also shows the relationship between classification accuracy and the structure of the training data set.
更多查看译文
关键词
flacco features,classification,sampling strategies,bi-objective
AI 理解论文
溯源树
样例
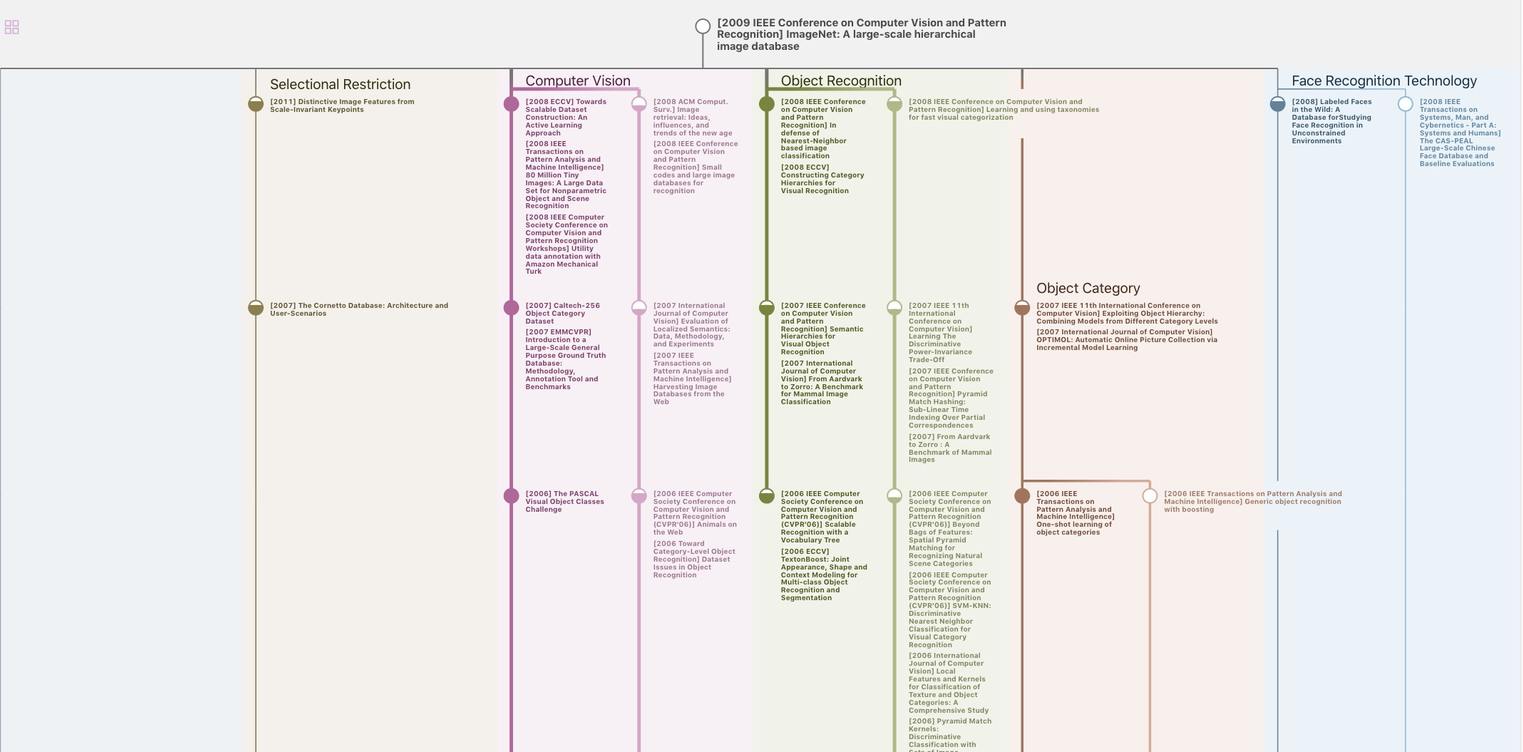
生成溯源树,研究论文发展脉络
Chat Paper
正在生成论文摘要