A Review of Federated Learning: Algorithms, Frameworks and Applications
Towards new e-Infrastructure and e-Services for Developing Countries(2023)
摘要
In today’s world, artificial intelligence (AI) and machine learning (ML) are being widely adopted at an exponential rate. A key requirement of AI and ML models is data, which often must be in proximity to these models. However, it is not always possible to “bring data to the model”, due to several reasons including legal jurisdictions, or ethical reasons, hence, a “taking the model to the data” might be a viable alternative. This process is called Federate Learning (FL), and it is a ML technique that allows devices or clients to collaboratively learn a shared model from the central server, while keeping the training data local and isolated. This ensures privacy and bandwidth preservation, especially in resource constrained environments. In this paper, a review of FL is done with a view of presenting the aggregation models, frameworks, and application areas, as well as identifying open challenges/gaps for potential research works.
更多查看译文
关键词
Federated Learning, Machine Learning, Federate Averaging
AI 理解论文
溯源树
样例
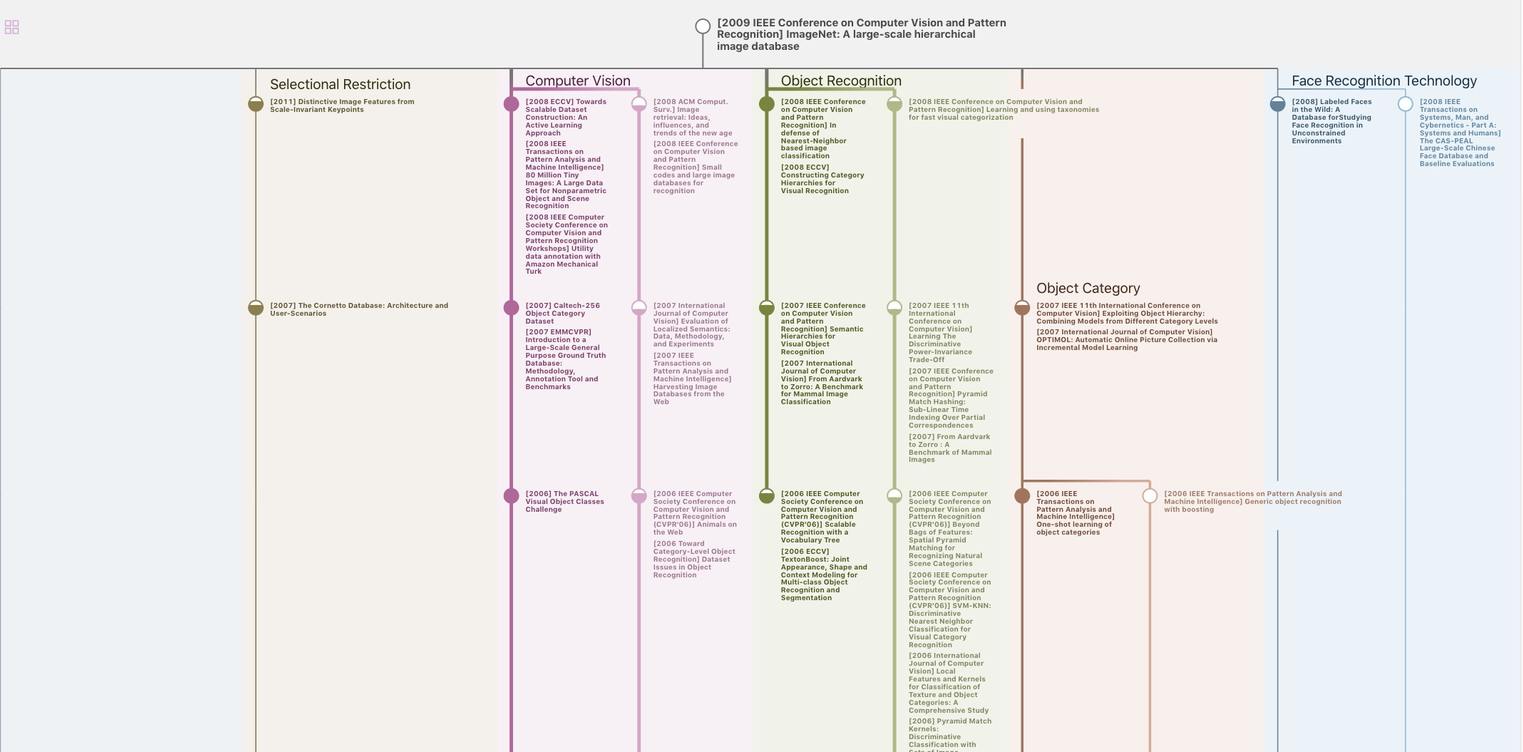
生成溯源树,研究论文发展脉络
Chat Paper
正在生成论文摘要