Cloud occurrence on the Antarctic plateau: ground-based detection and satellite products 
crossref(2022)
摘要
<div> <p><span>Downwelling </span><span>radiance </span><span>spectra in the 100-1500 cm</span><span>-1</span><span> interval, measured by the Radiation Explorer in the Far Infrared-Prototype for Applications and Development (REFIR-PAD) spectroradiometer on the Antarctic plateau since 2012, are ingested by an automatic machine learning algorithm, named cloud identification and classification (CIC), to detect and classify </span><span>the </span><span>Antarctic clouds.</span><span> </span></p> </div><div> <p><span>The CIC algorithm is a modified version of the one chained in the End-2-End Simulator (EES) of the Far-infrared Outgoing Radiation Understanding and Monitoring (FORUM) mission, the next ESA 9th Earth Explorer. CIC sits at the center of the decision tree of the FORUM EES for retrieving clear-sky atmospheric products or cloud properties.</span><span> </span></p> </div><div> <p><span>Co-located lidar measurements are exploited to define training sets composed of radiance spectra in presence of clear sky, ice clouds or mixed-phase clouds. The CIC is initially tested on a controlled verification subset for optimization. It is demonstrated that the information content in the far infrared (FIR) part of the spectrum is critical for the improvement of the performances of the algorithm to identify thin clouds and for cloud phase classification. A test set of 1726 spectra is then used to estimate the classification error. Unprecedented cloud occurrence statistics concerning more than 4 years of data are provided for multiple time scales and related to meteorological parameters such as surface air temperature and wind direction.</span><span> </span></p> </div><div> <p><span>The results indicate a clear sky mean annual occurrence of 72.3%, while ice and mixed-phase clouds are observed in 24.9% and 2.7% respectively, with an inter-annual variability of a few percent. The seasonal occurrence of clear sky shows a minimum in winter (66.8%) and maxima (75-76%) during intermediate seasons. In austral winter the mean surface temperature is about 9°C colder in clear conditions than when ice clouds are present. Mixed-phase clouds are observed only in the warm season (November-March). In austral summer they amount to more than one third of total observed clouds. Their occurrence is correlated with warmer surface temperatures. In the summer, the mean surface air temperature is about 5°C warmer when clouds are present than in clear sky conditions. </span><span> </span></p> </div><div> <p><span>A comparison of the CIC classification with available satellite Level-2 (L2) and Level-3 (L3) products, is provided. Passive (Infrared Atmospheric Sounding Interferometer - IASI, and Moderate Resolution Imaging Spectroradiometer - MODIS), and active (Cloud-Aerosol LiDAR with Orthogonal Polarization - CALIOP, and the Cloud Profiling Radar - CPR) sensors are considered.  </span><span> </span></p> </div><div> <p><span>For selected case studies, a direct comparison between co-located L2 satellite products and parameters retrieved from ground-based observations (REFIR-PAD, lidar) is performed. In case of cloudy scenes, retrieved cloud parameter are used to simulate FORUM like observations and to evaluate the impact of the additional FIR part of spectrum in satellite cloud retrievals from infrared passive measurements.</span><span> </span></p> </div><div> <p><span>For L3 satellite products, the monthly gridded data are used for comparison. The differences observed among the considered products and the CIC results are analysed in terms of footprint sizes and sensors' sensitivities. The comparison highlights the ability of the CIC/REFIR-PAD to identify multiple cloud conditions from high spectral resolution radiances.</span><span> </span></p> </div>
更多查看译文
AI 理解论文
溯源树
样例
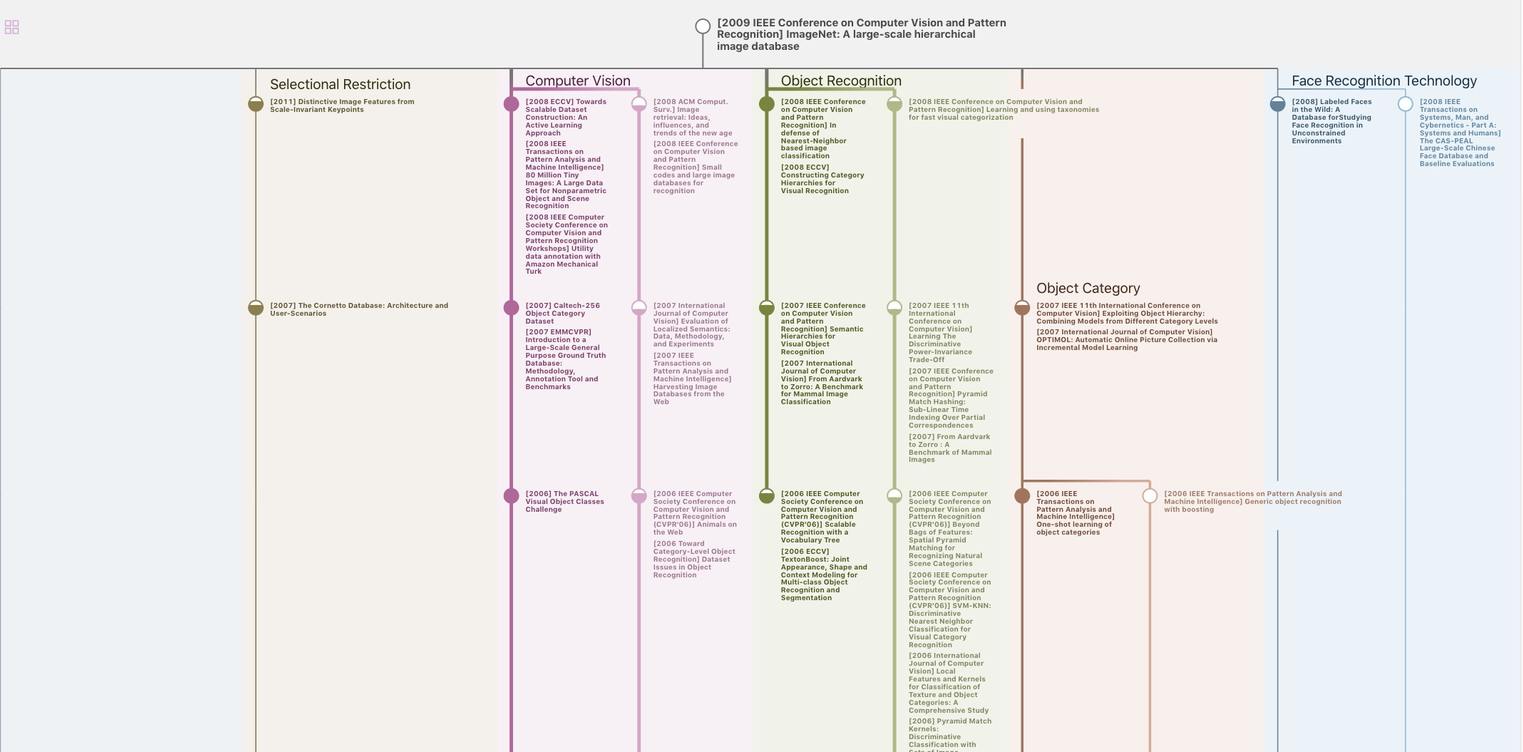
生成溯源树,研究论文发展脉络
Chat Paper
正在生成论文摘要