Assessing WHODAS 2.0 Scores from Behavioral Biomarkers: a Data-driven Approach (Preprint)
crossref(2022)
摘要
BACKGROUND Functional limitations are associated with poor clinical outcomes, higher mortality, and disability rates, especially in the elderly. Continuous assessment of patients’ functionality is important for clinical practice; however, traditional questionnaire-based assessment methods are very time-consuming and infrequently used. Mobile sensing offers a great range of sources that can assess function and disability daily. OBJECTIVE This work aims to prove the feasibility of an interpretable machine learning pipeline for predicting WHODAS 2.0 outcomes utilizing solely passively collected digital biomarkers. METHODS One-month long time-series data were summarized using statistical measures (minimum, maximum, mean, median, standard deviation, IQR), creating 64 features. We then applied a sequential feature selection on each WHODAS 2.0 domain (cognition, mobility, self-care, getting along, life activities, participation). Finally, we predicted the WHODAS 2.0 functional domain scores using linear regression using the best feature subsets. We reported the mean absolute errors and the mean absolute percentage errors as goodness-of-fit statistics to evaluate the model. RESULTS Our machine learning-based models for predicting patients’ WHODAS functionality scores per domain achieved a mean absolute percentage error varying between 14.86% and 27.21% among the domains with a set of interpretable features for each domain. CONCLUSIONS Our findings show the feasibility of using machine learning-based methods to assess functional health solely from passively sensed mobile data. The feature selection step provides a set of interpretable features for each domain, ensuring better explainability to the models’ decisions.
更多查看译文
AI 理解论文
溯源树
样例
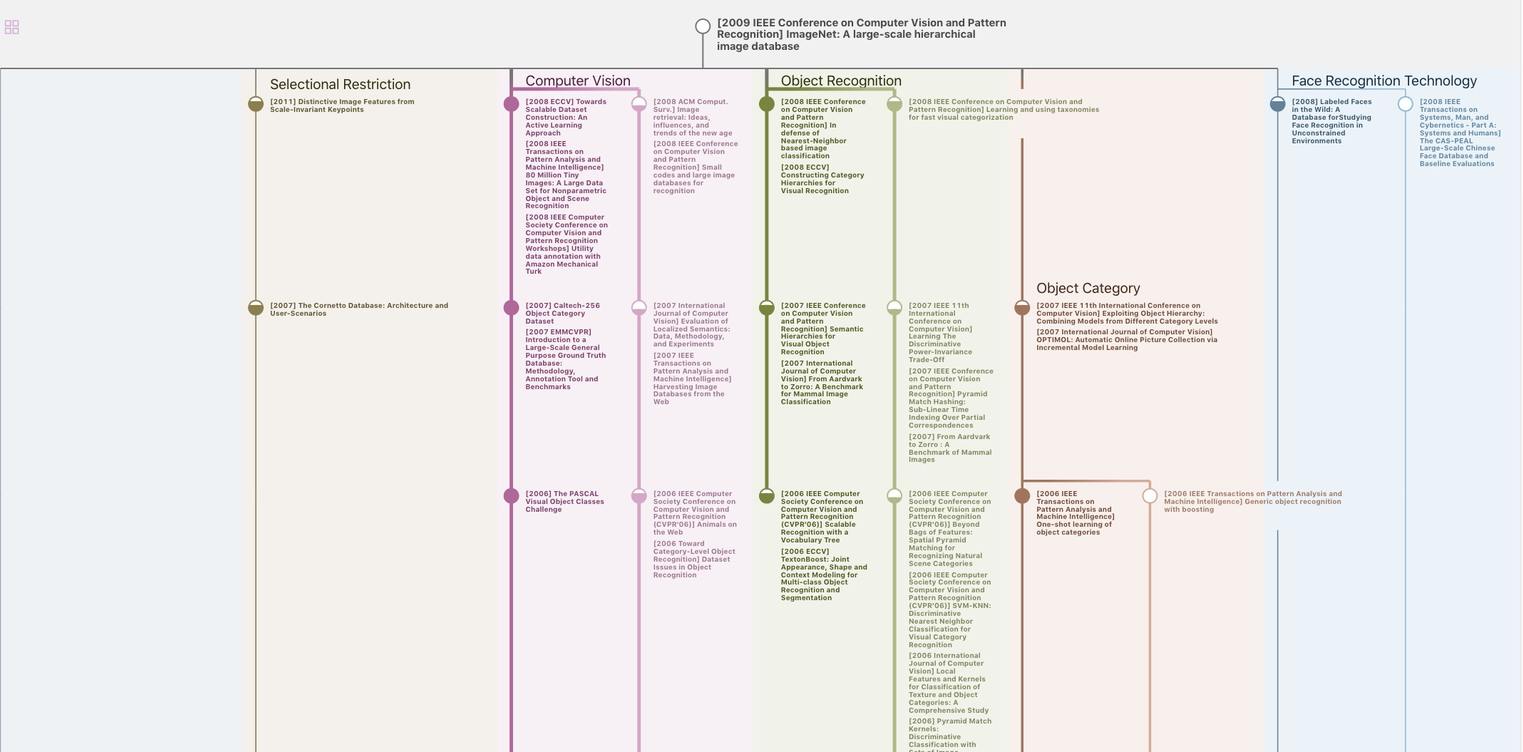
生成溯源树,研究论文发展脉络
Chat Paper
正在生成论文摘要