Sparse ECG denoising with generalized entropy penalty
Research Square (Research Square)(2022)
摘要
Abstract Background Electrocardiogram (ECG) monitoring can quickly obtain the human body's ECG signal, and it is convenient for researchers to analyze the variability of heart rate. Some noise is often introduced in the process of signal acquisition and transmission. The existence of these noises interferes with the authenticity of the signal, and it is not conducive to the signal analysis and processing. Method In this paper, for the purpose of suppressing these noises, a sparse ECG denoising framework combining low-pass filtering and sparsity recovery is investigated. We propose a sparse ECG denoising method based on generalized entropy penalty. We use a novel denoising penalty function for sparse recovery, and compared the proposed generalized entropy penalty (GEP) with \({\ell _{\text{1}}}\)-norm penalty. Result The proposed method is evaluated on ECG signals from the MIT-BIH Arrhythmia database. The results show that the proposed method overcomes the problem of underestimation of the high-frequency components amplitude of the ECG signal. At the same time, the GEP punishes the large values less severely than \({\ell _{\text{1}}}\)-norm, and can also be used to overcome the shortcomings of "\({\ell _{\text{1}}}\)-norm" underestimating the QRS wave in ECG signal. Conclusion The proposed GEP-based penalty can be used for ECG denoising. The result of signal denoising is to remove the noise without destroying the information of the signal itself. The method has a higher restoration degree of the original signal and has relatively excellent denoising effect.
更多查看译文
关键词
generalized entropy penalty,ecg,sparse
AI 理解论文
溯源树
样例
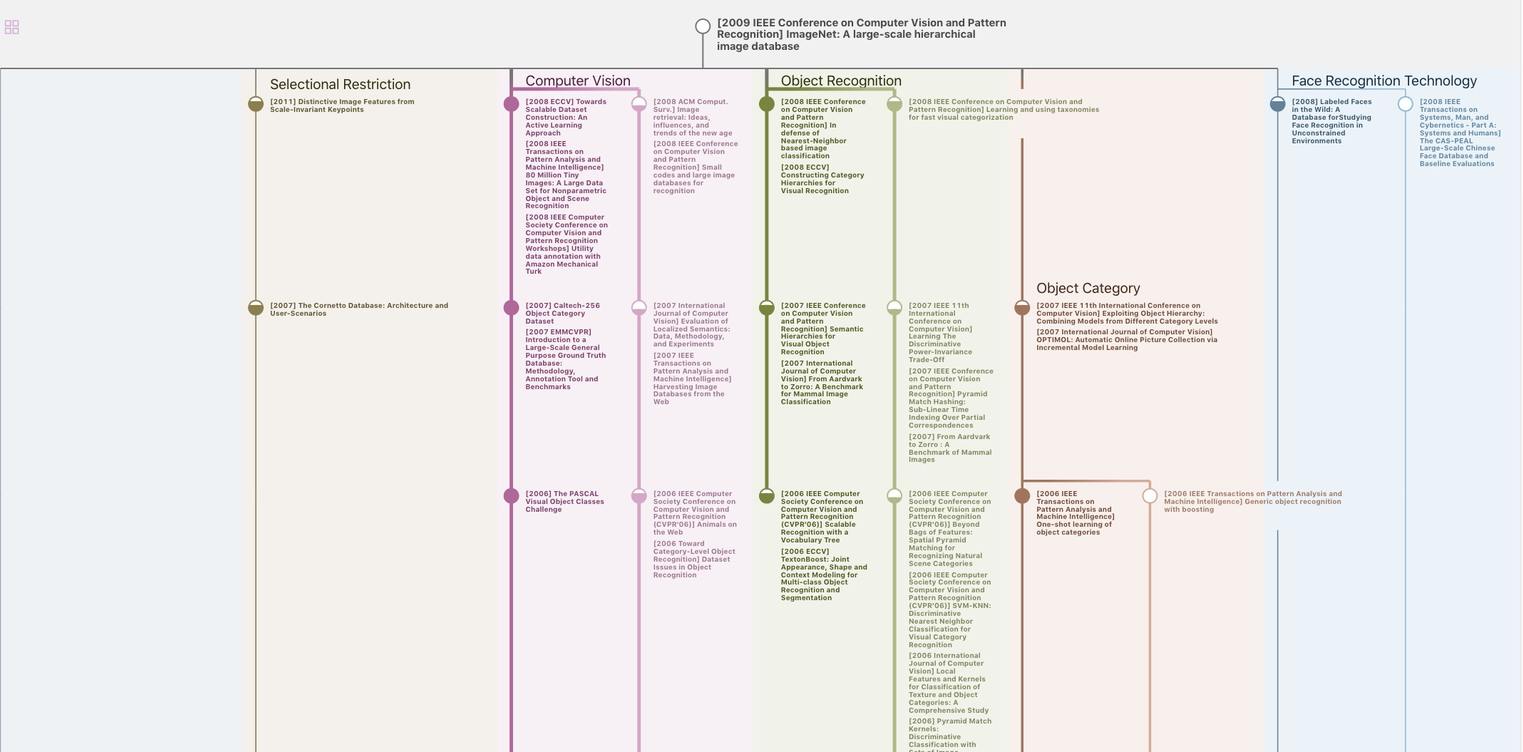
生成溯源树,研究论文发展脉络
Chat Paper
正在生成论文摘要