Drug-disease association prediction via geometric deep learning and negative sample selection
crossref(2022)
摘要
Abstract Background: Traditional drug development takes a good deal of time and effort, computational drug repositioning enables to discover the underlying mechanisms of drugs, thereby reducing the cost of drug discovery and development. However, present methods merely consider structure-based drug-related network for feature extraction of drugs and diseases in the biological network. Results: In this paper, we develop a novel model, namely DRGDL, based on geometric deep learning and negative sample selection to infer potential drug-disease associations (DDAs), which can more comprehensively make use of the biological features of drugs and diseases. Specifically, the lower-order and higher-order representations of drugs and diseases are captured by two different geometric deep learning strategies, and then negative samples are constructed by a negative selection strategy. A machine learning classifier is applied to complete the prediction task of DDAs by integrating two representations of drugs and diseases. Experiment results demonstrate that DRGDL achieves excellent performance under ten-fold cross-validation on the benchmark dataset.
更多查看译文
AI 理解论文
溯源树
样例
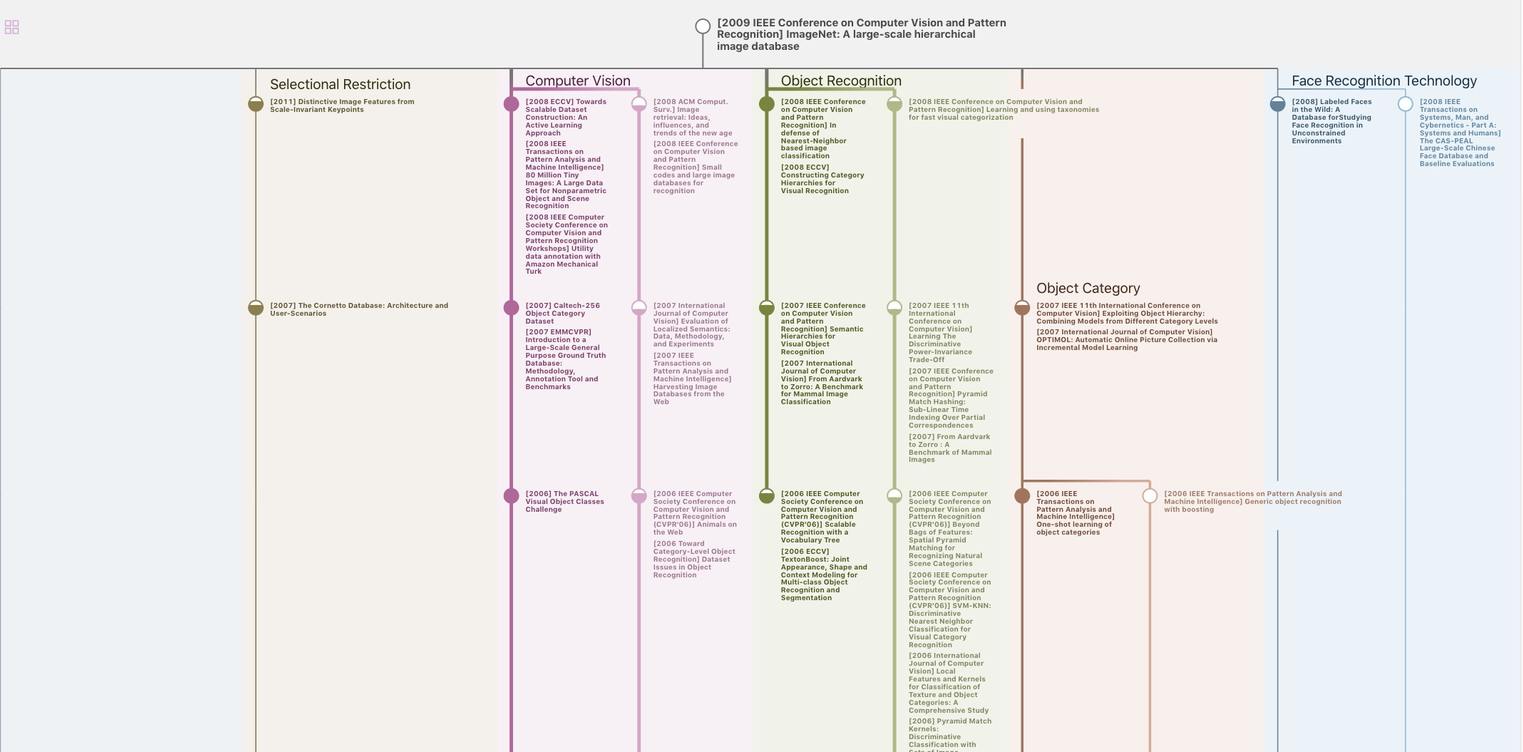
生成溯源树,研究论文发展脉络
Chat Paper
正在生成论文摘要