Predicting the status of human complex diseases with random forest and polygenic risk scores
crossref(2022)
摘要
Abstract In recent years, polygenic risk score (PRS) analysis has become one of the most practical ways to leverage genome wide association studies (GWAS) findings for disease prediction. This approach is useful for addressing the challenge to translate the vast knowledge of complex disease genetics into clinically usable information. As machine learning is being widely applied to life science and PRS analysis comes into wide use for disease prediction, we systematacially evaluated the performance of random forest and PRS in predicting the status of complex diseases. Simulation studies were conducted by the GWAsimulator software, considering various genetic effects, genetic models and sample sizes. Two target complex disease related diseases and two environmental exposure factors were also simulated to obtain the additional genetic information of target complex disease, which were generally ignored in previous PRS studies. We found that PRS-based disease prediction using random forest had moderate accuracies (~ 70%) under various scenarios simulated by this study. The genetic effects of simulated disease loci showed the most significant impact on the performance of PRS-based disease prediction. This novel approach can leverage pleiotropy and gene-environment interactions. Furthermore, it is an attempt combining publicly available summary statistics and individual-level genotype data. We hope that this study provides useful information for further approaches development and disease prediction.
更多查看译文
AI 理解论文
溯源树
样例
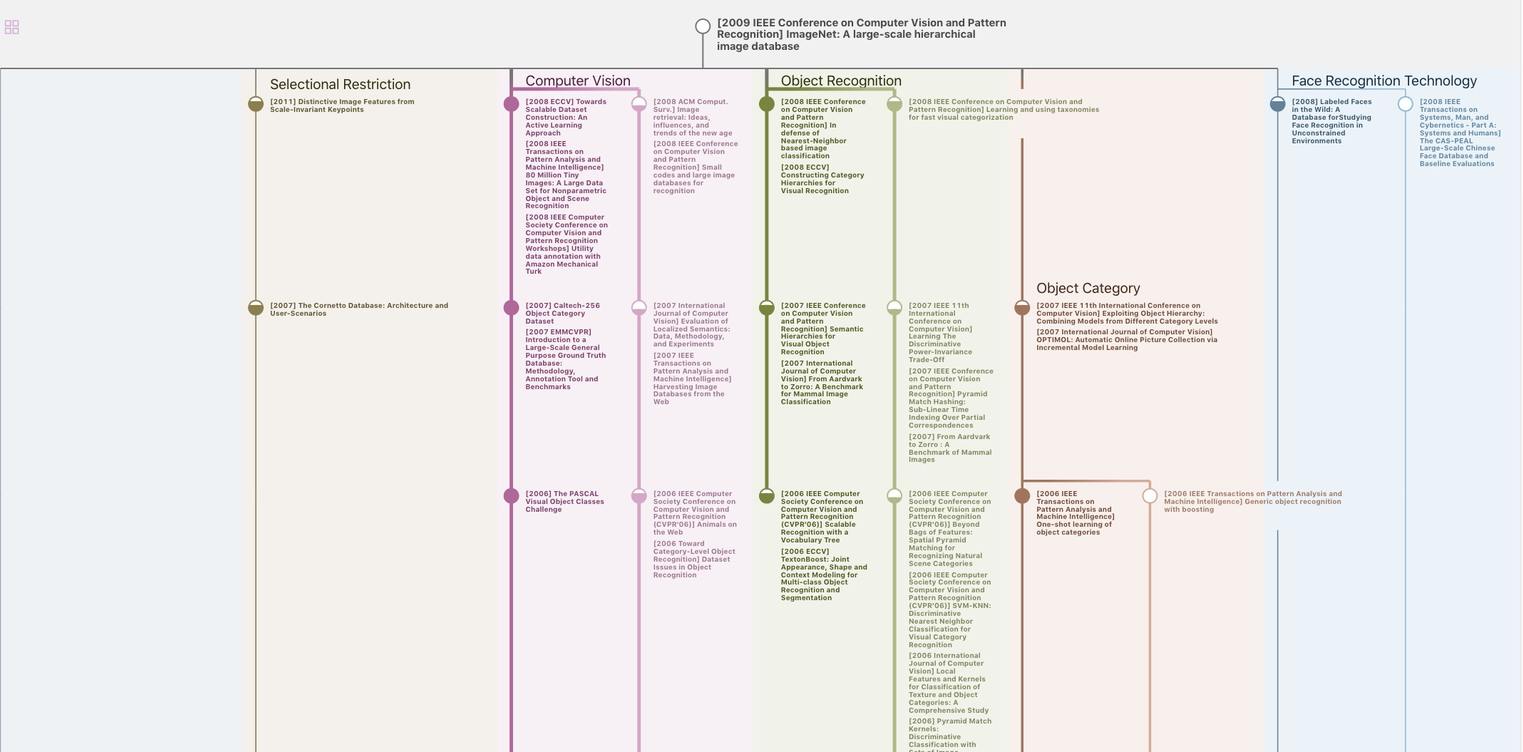
生成溯源树,研究论文发展脉络
Chat Paper
正在生成论文摘要