Fisher Discriminative Embedding Low-Rank Sparse Representation for Music Genre Classification
Research Square (Research Square)(2022)
摘要
Abstract This work focuses on a music genre classification method based on a sparse low-rank representation. Sparse low-rank representation has been proposed as an effective way to learn the classifier, which aims to learn a row-sparse and low-rank representation matrix for correcting noise and identifying the subspace structure in the data contaminated by outliers. However, these related methods fail to utilize the discriminative information to mine the rich supervision information available in the training samples. To address this issue, a novel Fisher Discriminative Embedding Low-Rank Sparse Representation (FDLRSR) classification algorithm is proposed based on the Fisher criterion, which results in stronger intra-class similarity and inter-class separability representation coefficients. Meanwhile, its two special cases, i.e., the Fisher Discriminative Embedding Low-Rank Representation (FDLR) and Fisher Discriminative Embedding Sparse Representation (FDSR) are also presented in this work. Specifically, the proposed classification method employs the FDLRSR algorithm coupled with the feature combinations consisting of acoustic features and spectral features for music genre classification tasks by minimizing the residuals. Compared with the several state-of-the-art music genre classification methods, the proposed methods substantially improve the classification results on three widely used datasets, the GTZAN, ISMIR2004 and Homburg datasets, which verify its effectiveness and availability.
更多查看译文
关键词
music genre classification,low-rank
AI 理解论文
溯源树
样例
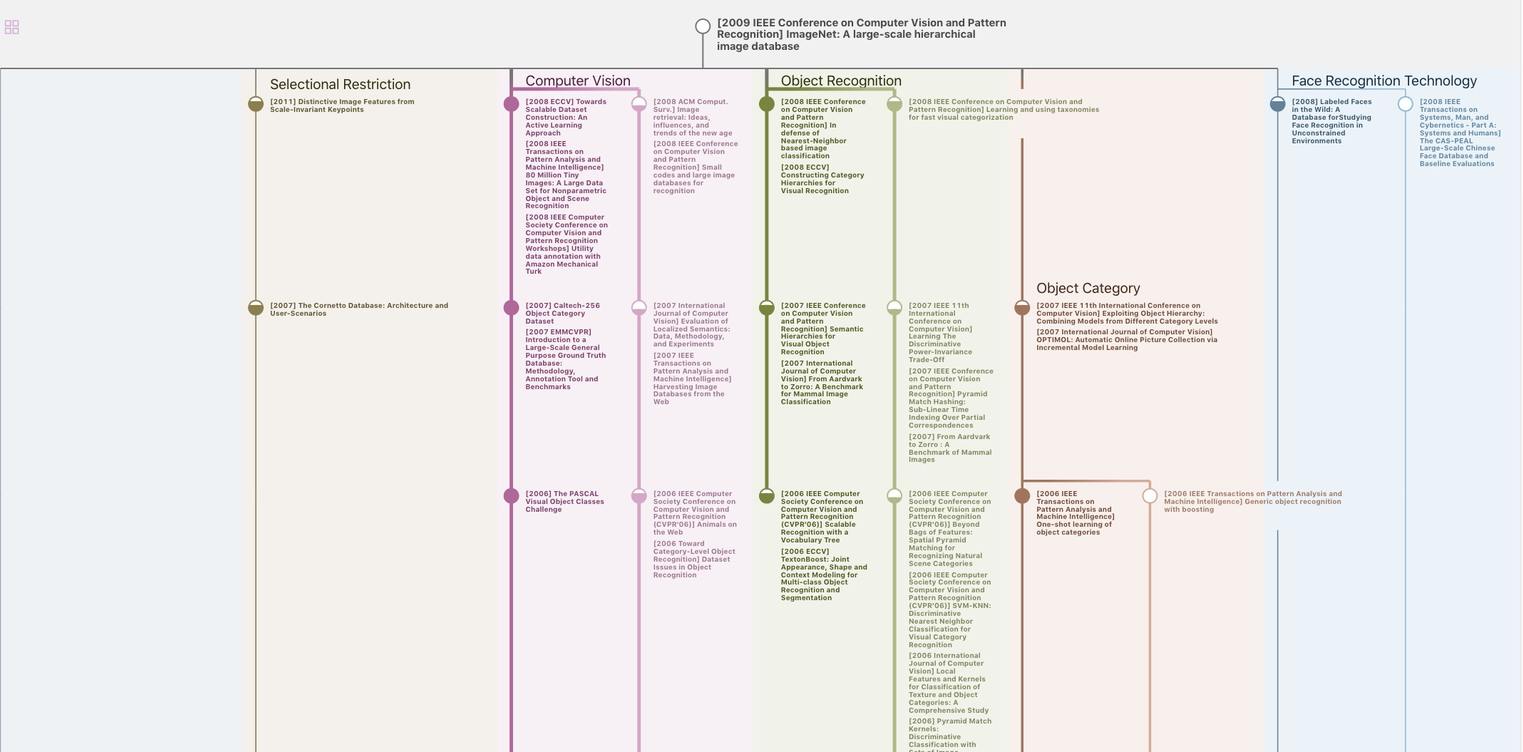
生成溯源树,研究论文发展脉络
Chat Paper
正在生成论文摘要