Smartformer: An Intelligent Model Compression Framework for Transformer
Research Square (Research Square)(2022)
摘要
Abstract Transformer, as one of the latest popular deep neural networks (DNNs), has achieved outstanding performance in various areas. However, this model usually requires massive amounts of parameters to fit. Over-parameterization not only brings storage challenges in a resource-limited setting but also inevitably results in the model over-fitting. Even though literature works introduced several ways to reduce the parameter size of Transformers, none of them have focused on addressing the over-parameterization issue by concurrently considering the following three objectives: preserving the model architecture, maintaining the model performance, and reducing the model complexity (number of parameters) to improve its convergence property. In this study, we propose an intelligent model compression framework, Smartformer, by incorporating reinforcement learning and CP-decomposition techniques. This framework has two advantages: 1) from the perspective of convenience, researchers can directly exploit this framework to train their designed Transformer models as usual without fine-tuning the original model training hyperparameters; 2) from the perspective of effectiveness, researchers can simultaneously fulfill the aforementioned three objectives by using this framework. We conduct two basic experiments and three practical experiments to demonstrate the proposed framework using various datasets and existing Transformer models. The results demonstrate that the Smartformer is able to improve the existing Transformers regarding the model performance and model complexity by intelligently incorporating the model decomposition and compression in the training process.
更多查看译文
关键词
intelligent model compression framework,transformer
AI 理解论文
溯源树
样例
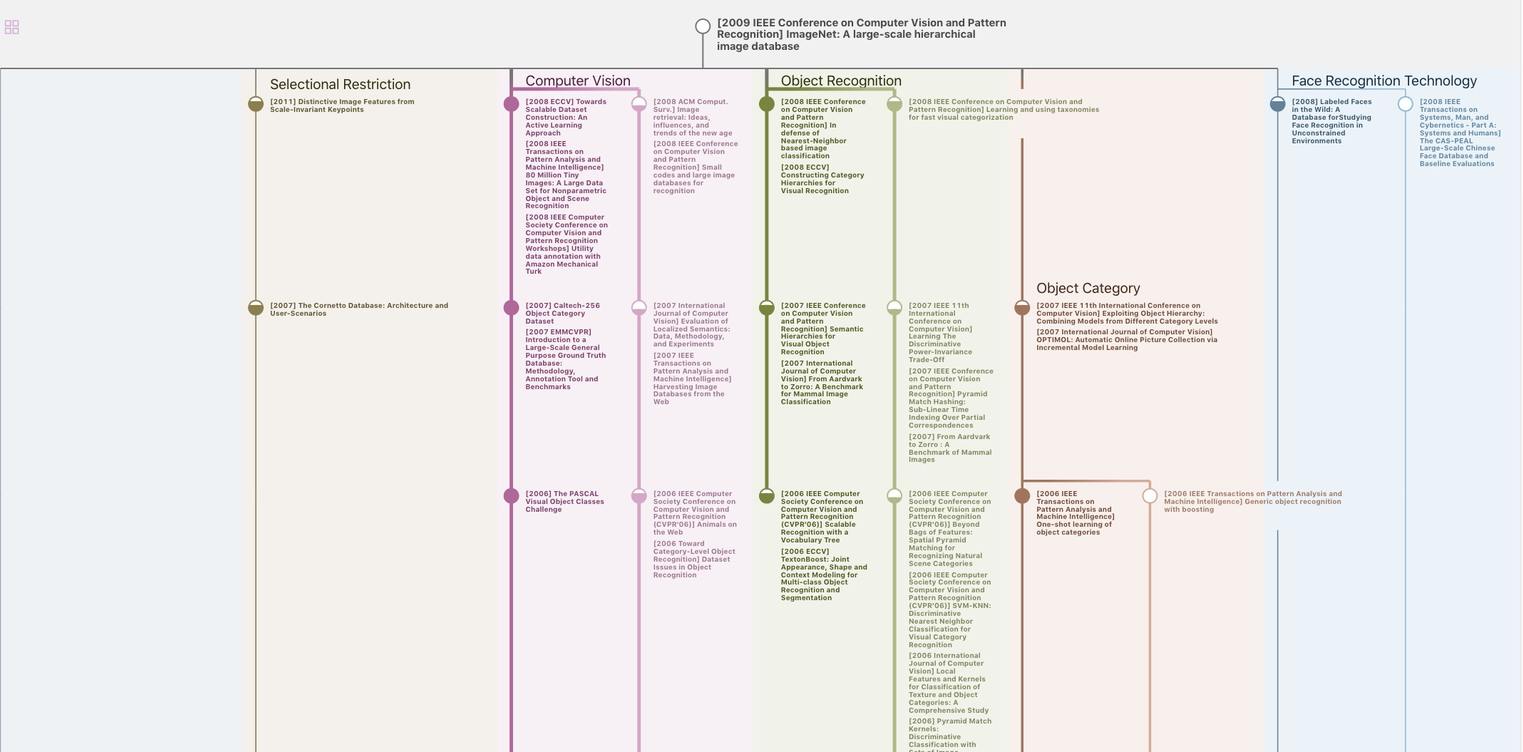
生成溯源树,研究论文发展脉络
Chat Paper
正在生成论文摘要