Element-wise representations with ECNet for material property prediction and applications in high-entropy alloys
Research Square (Research Square)(2022)
摘要
Abstract To develop machine learning models for accurate property prediction, the current graph networks are designed to give a sufficient representations of materials. However, the relationships between the atomic and structural inputs and many target properties are very complex, and even insensitive to the local environment. Here, we propose the elemental convolution (EC) operation to obtain a more general and global element-wise representations, and develop EC graph neural networks (ECNet) to accurately model material properties. We demonstrate that the ECNet models show better prediction in properties like band gaps, refractive index, and elastic moduli of crystals. The element-wise feature vectors are able to capture information of elemental types and crystal structures, and show a special characteristics related to its target properties. To explore its application on high entropy alloys, we focus on the properties modelling on CrFeNiCoMn/Pd high entropy alloys. Multi-task learning of ECNet models show its advantage in accuracy and convenience for multiple property predictions. Furthermore, we demonstrate that the transfer learning (TL) from the less-principal element alloys can enhance model performance in multiple-principal element alloys. Generally, the TL element-wise feature vectors extracted from parent model can be used as universal descriptors and retain good accuracy at small data limits when combined with simple multi-layer perceptrons. Finally, the TL framework is used to do interpolations in Fe-Co-Mn system to understand the underlying physics including the stability and magnetic moment.PACS numbers: 74.25.-q,71.15.-m,89.20.Ff
更多查看译文
关键词
material property prediction,alloys,ecnet,element-wise,high-entropy
AI 理解论文
溯源树
样例
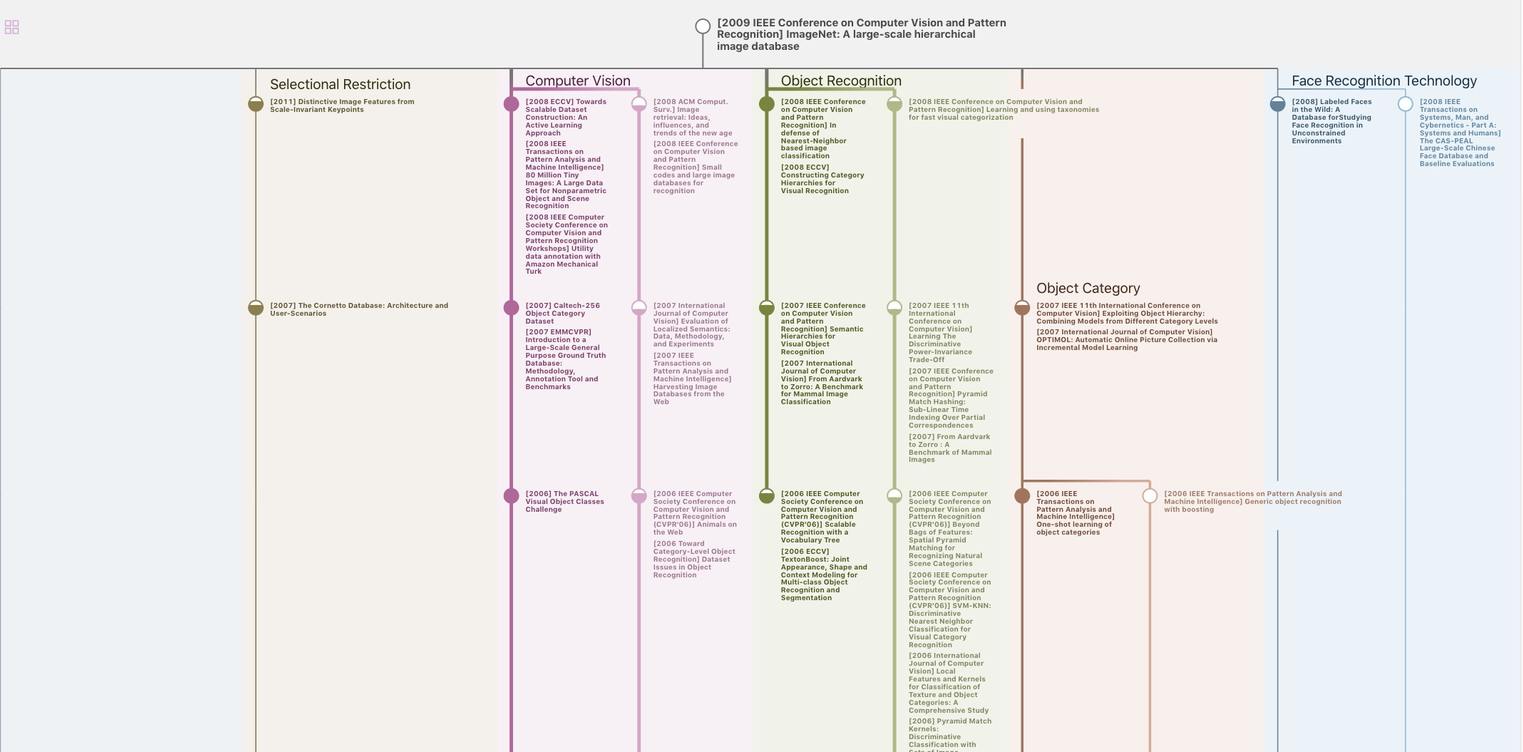
生成溯源树,研究论文发展脉络
Chat Paper
正在生成论文摘要