Classifying Synoptic Patterns and Identifying Sensitive Areas for Targeted Observations to Improve Air Quality Prediction over South Korea
crossref(2022)
摘要
<p>In air quality prediction, initial conditions, based on both atmospheric and aerosol/chemistry observations, are essentially required for a coupled atmosphere-chemistry model. In general, we have more accurate model results with a higher amount and quality of observations; however, forecast errors in a region of interest may grow from an initial error in a specific upstream region, primarily due to the lack of observations therein. Therefore, finding the upstream areas from which a small initial error can grow into significant forecast errors in the region of interest is essential for the strategic enhancement of observations to improve numerical air quality prediction. The conditional nonlinear optimal perturbation for initial conditions (CNOP-I) represents the initial error that can lead to the most significant error at the forecast time, which enables us to identify the sensitive regions as a considerable initial error value. Thus, CNOP-I is a suitable tool for targeted (adaptive) observations. The goal of this study is twofold: 1) to classify the synoptic patterns for sand dust storm cases by using the principal component analysis (PCA), and 2) to identify the sensitive areas in targeted observations for air quality prediction via CNOP-I. We focus on the Asian dust storm events that occurred over South Korea during the last 32 years (1990—2021). We expect to improve air quality forecasts by classifying the synoptic situations that bring about severe dust storm outbreaks in South Korea and by identifying the upstream areas for targeted observations to which we can potentially enhance observations through international collaborations.</p>
更多查看译文
AI 理解论文
溯源树
样例
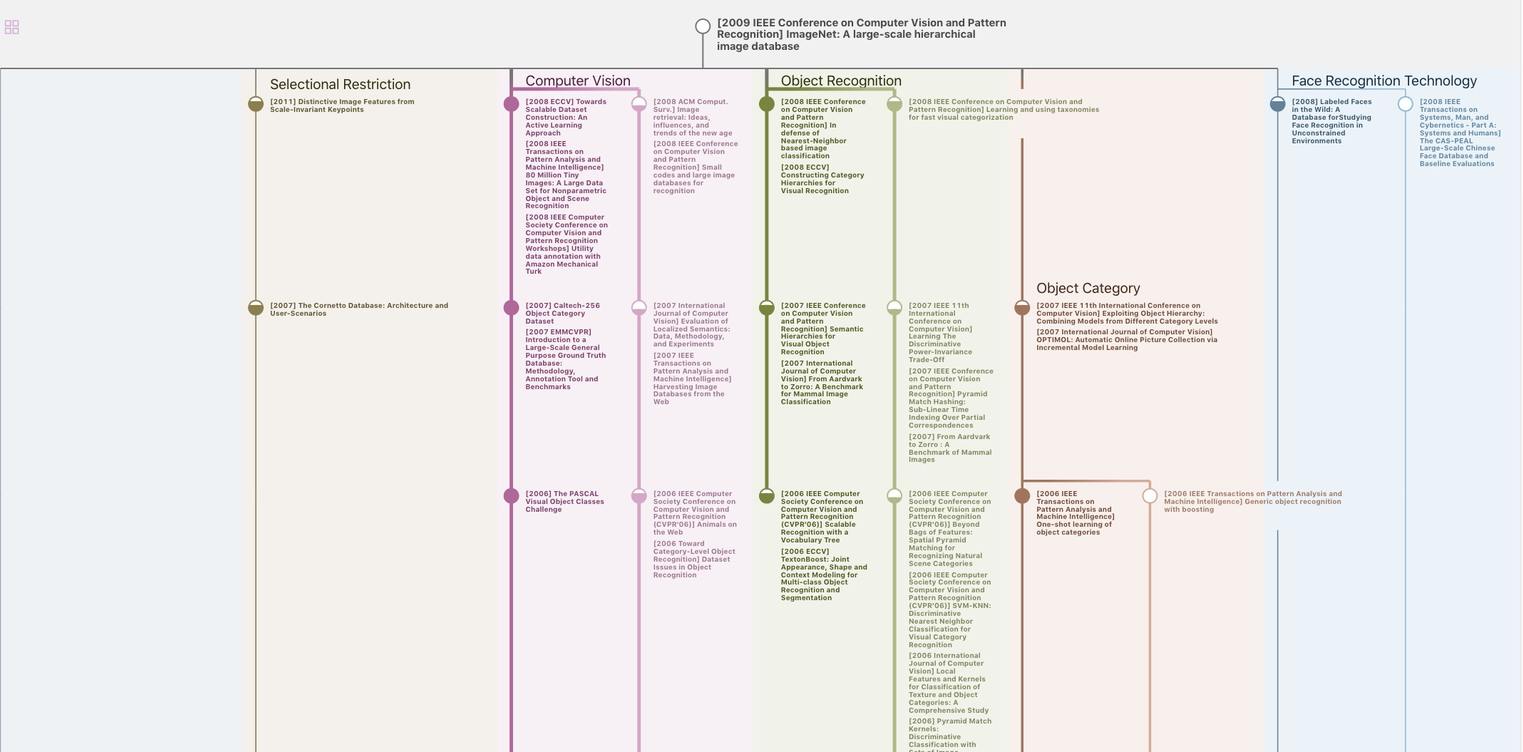
生成溯源树,研究论文发展脉络
Chat Paper
正在生成论文摘要