Data Compressive Dictionary Matching Based Optimization Approach for Non-Intrusive Load Monitoring
International Review of Electrical Engineering-iree(2022)
摘要
Non-Intrusive Load Monitoring (NILM) supports energy management by characterizing the energy usage patterns of individual appliances from aggregate demand measurements. Machine learning algorithms for NILM require bulk training data while optimization techniques involve high computation time. Addressing these issues, this paper presents an intelligent dictionary matching-based optimization approach for NILM of finite-state residential appliances. The operating states of the target appliances are determined by K-means clustering using limited training data. Employing these power states, a consumer-specific dictionary containing all the possible combinations of aggregate demand is constructed in sorted form. To reduce the computational burden, the number of aggregate demand samples is reduced by event-driven data compression. These compressed samples are then matched in the sorted dictionary to estimate the share of individual appliances in aggregate demand. Furthermore, a post-processing algorithm is proposed to eliminate the effect of sudden spikes/dips during state transitions of appliances. The proposed algorithm is evaluated using the low-frequency Reference Energy Disaggregation Dataset and its results are compared with recent approaches. The comparison results show that the proposed approach gives better results as compared to recent techniques with low computational effort. The proposed approach can support energy managers in managing demand side resources of residential microgrids.
更多查看译文
关键词
optimization,monitoring,non-intrusive
AI 理解论文
溯源树
样例
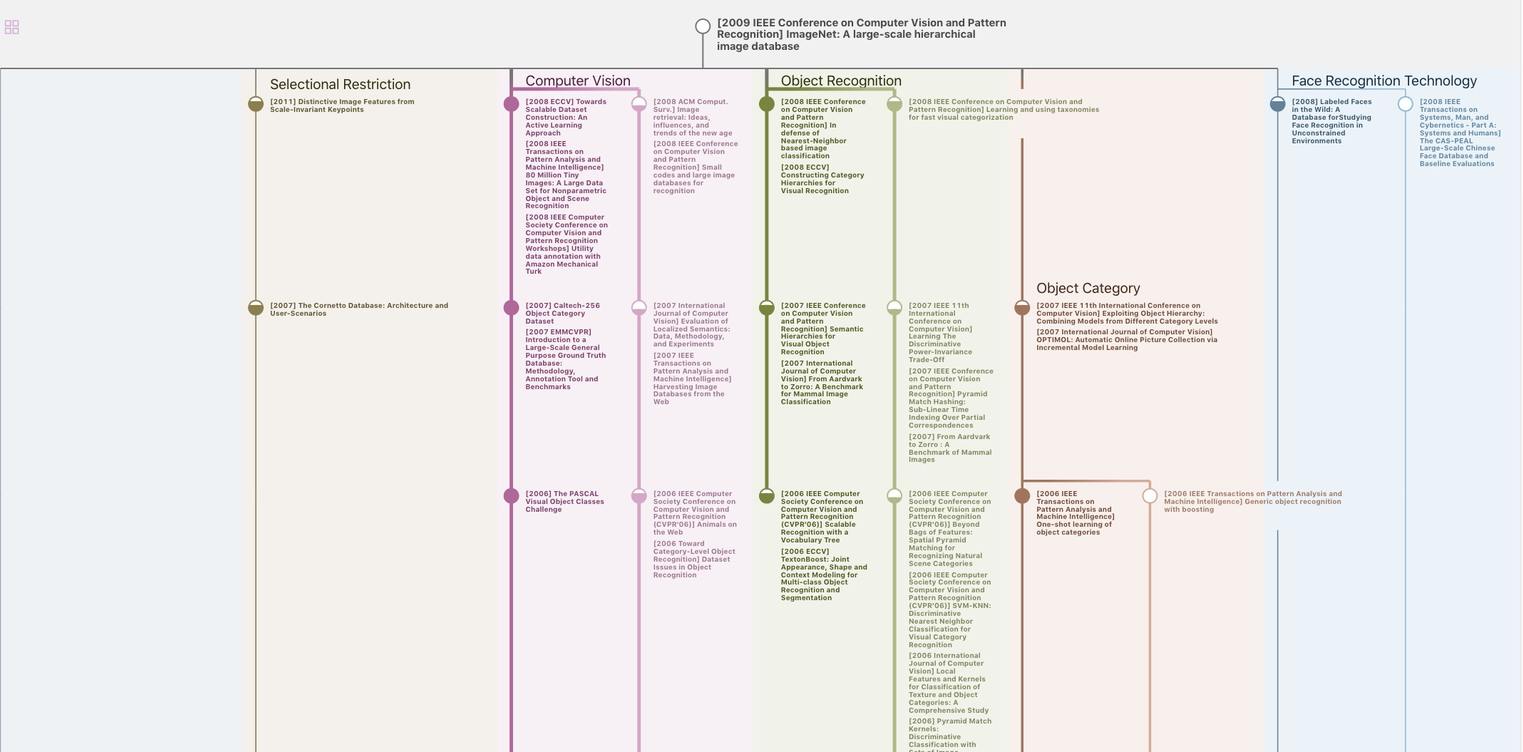
生成溯源树,研究论文发展脉络
Chat Paper
正在生成论文摘要