Machine Learning-assisted Single Vessel Analysis of Nanoparticle Permeability in Tumour Vasculatures
Research Square (Research Square)(2022)
摘要
Abstract The central dogma of nanoparticle delivery to tumours through enhanced leakiness of vasculatures has become a topic of debate in recent years. To address this problem, we created a single-vessel quantitative analysis method by taking advantage of protein-based nanoprobes and image segmentation-based machine learning technology (Nano-ISML). Using Nano-ISML, we quantified > 50,000 individual blood vessels from 32 tumour models, which revealed highly heterogenous vascular permeability of protein-based nanoparticles in different tumours and blood vessels. There was > 20-fold difference in the percentage of high-permeable vessels in different tumours and > 100-fold penetration ability in vessels with the highest permeability compared to vessels with the lowest permeability. We demonstrated that this phenomenon resulted from diversified vascular penetration mechanisms. Specifically, passive extravasation and trans-endothelial transport were dominant mechanisms for high-permeable and low-permeable tumour vessels, respectively. Furthermore, to exemplify Nano-ISML assisted rational design of desirable nanomedicines, we developed genetically tailored protein nanoparticles that improved trans-endothelial transport in low-permeable tumours. Our study delineates the heterogeneity of tumour vascular permeability and defines a direction for rational design of the next generation anti-cancer nanomedicines.
更多查看译文
关键词
nanoparticle permeability,single vessel analysis,tumour,learning-assisted
AI 理解论文
溯源树
样例
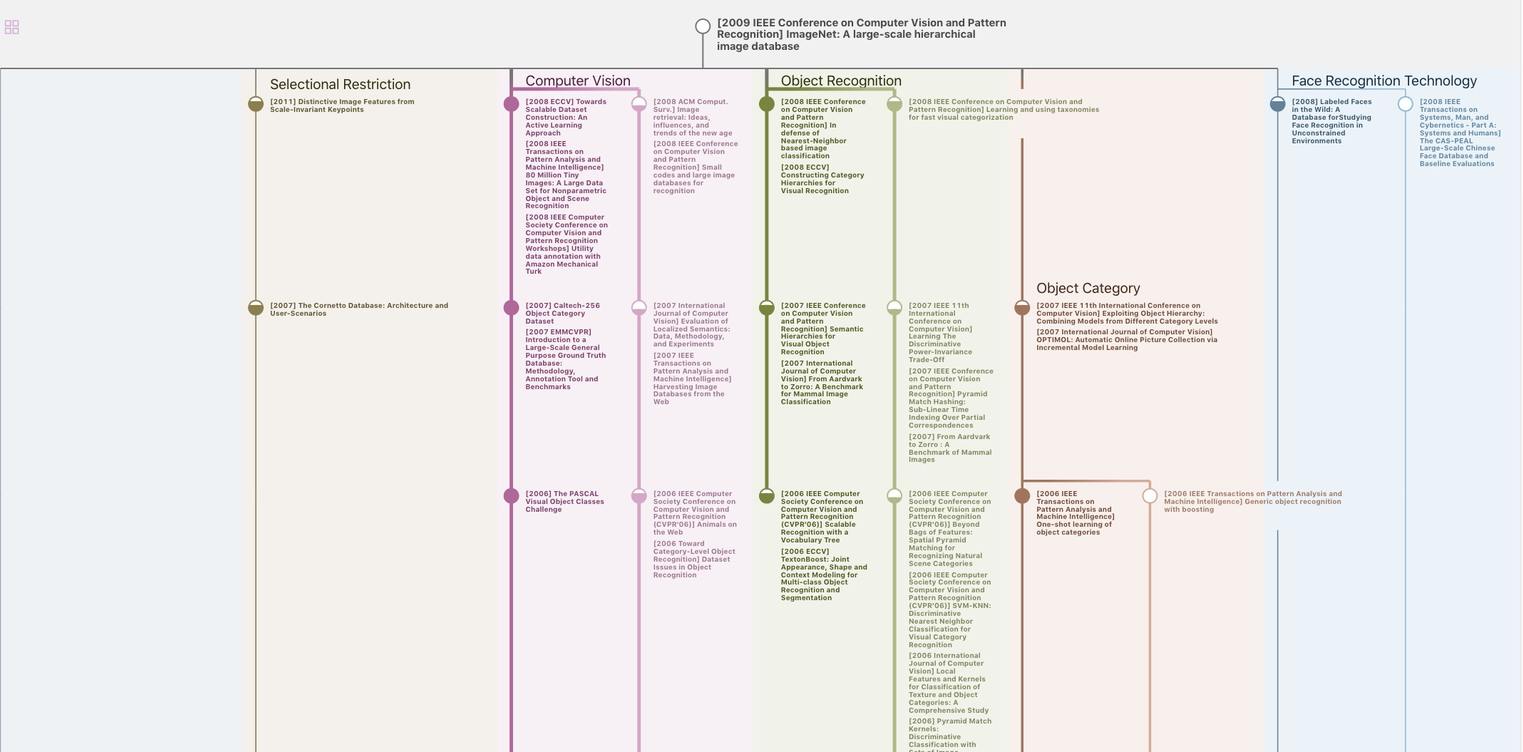
生成溯源树,研究论文发展脉络
Chat Paper
正在生成论文摘要