Gain Confidence, Reduce Disappointment: A New Approach to Cross-Validation for Sparse Regression
CoRR(2023)
摘要
Ridge regularized sparse regression involves selecting a subset of features that explains the relationship between a design matrix and an output vector in an interpretable manner. To select the sparsity and robustness of linear regressors, techniques like leave-one-out cross-validation are commonly used for hyperparameter tuning. However, cross-validation typically increases the cost of sparse regression by several orders of magnitude. Additionally, validation metrics are noisy estimators of the test-set error, with different hyperparameter combinations giving models with different amounts of noise. Therefore, optimizing over these metrics is vulnerable to out-of-sample disappointment, especially in underdetermined settings. To address this, we make two contributions. First, we leverage the generalization theory literature to propose confidence-adjusted variants of leave-one-out that display less propensity to out-of-sample disappointment. Second, we leverage ideas from the mixed-integer literature to obtain computationally tractable relaxations of confidence-adjusted leave-one-out, thereby minimizing it without solving as many MIOs. Our relaxations give rise to an efficient coordinate descent scheme which allows us to obtain significantly lower leave-one-out errors than via other methods in the literature. We validate our theory by demonstrating we obtain significantly sparser and comparably accurate solutions than via popular methods like GLMNet and suffer from less out-of-sample disappointment. On synthetic datasets, our confidence adjustment procedure generates significantly fewer false discoveries, and improves out-of-sample performance by 2-5% compared to cross-validating without confidence adjustment. Across a suite of 13 real datasets, a calibrated version of our procedure improves the test set error by an average of 4% compared to cross-validating without confidence adjustment.
更多查看译文
关键词
reduce disappointment,regression,confidence,cross-validation
AI 理解论文
溯源树
样例
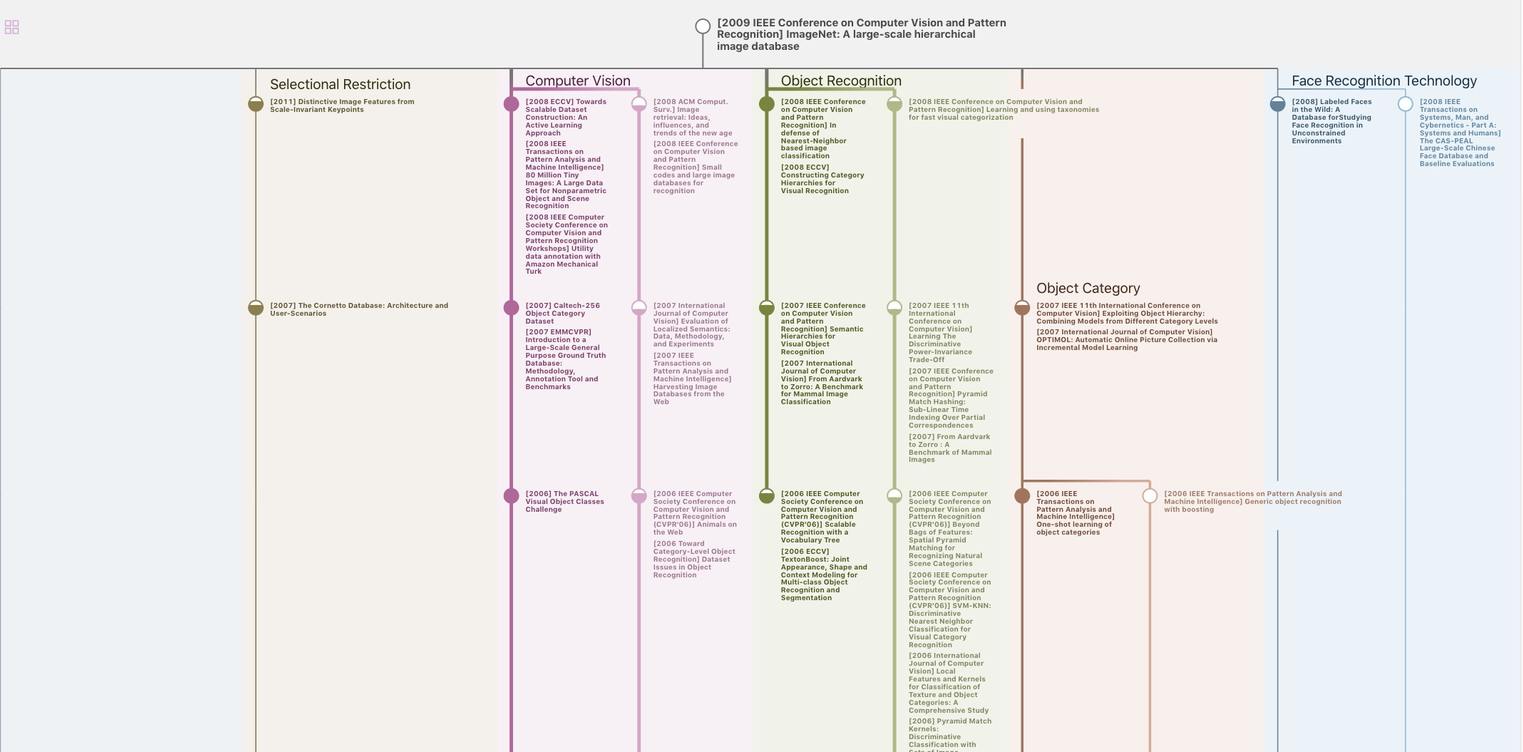
生成溯源树,研究论文发展脉络
Chat Paper
正在生成论文摘要