Protein Discovery with Discrete Walk-Jump Sampling
ICLR 2024(2023)
摘要
We resolve difficulties in training and sampling from a discrete generative model by learning a smoothed energy function, sampling from the smoothed data manifold with Langevin Markov chain Monte Carlo (MCMC), and projecting back to the true data manifold with one-step denoising. Our Discrete Walk-Jump Sampling formalism combines the maximum likelihood training of an energy-based model and improved sample quality of a score-based model, while simplifying training and sampling by requiring only a single noise level. We evaluate the robustness of our approach on generative modeling of antibody proteins and introduce the distributional conformity score to benchmark protein generative models. By optimizing and sampling from our models for the proposed distributional conformity score, 97-100% of generated samples are successfully expressed and purified and 35% of functional designs show equal or improved binding affinity compared to known functional antibodies on the first attempt in a single round of laboratory experiments. We also report the first demonstration of long-run fast-mixing MCMC chains where diverse antibody protein classes are visited in a single MCMC chain.
更多查看译文
关键词
generative modeling,langevin mcmc,energy-based models,score-based models,protein design,protein discovery
AI 理解论文
溯源树
样例
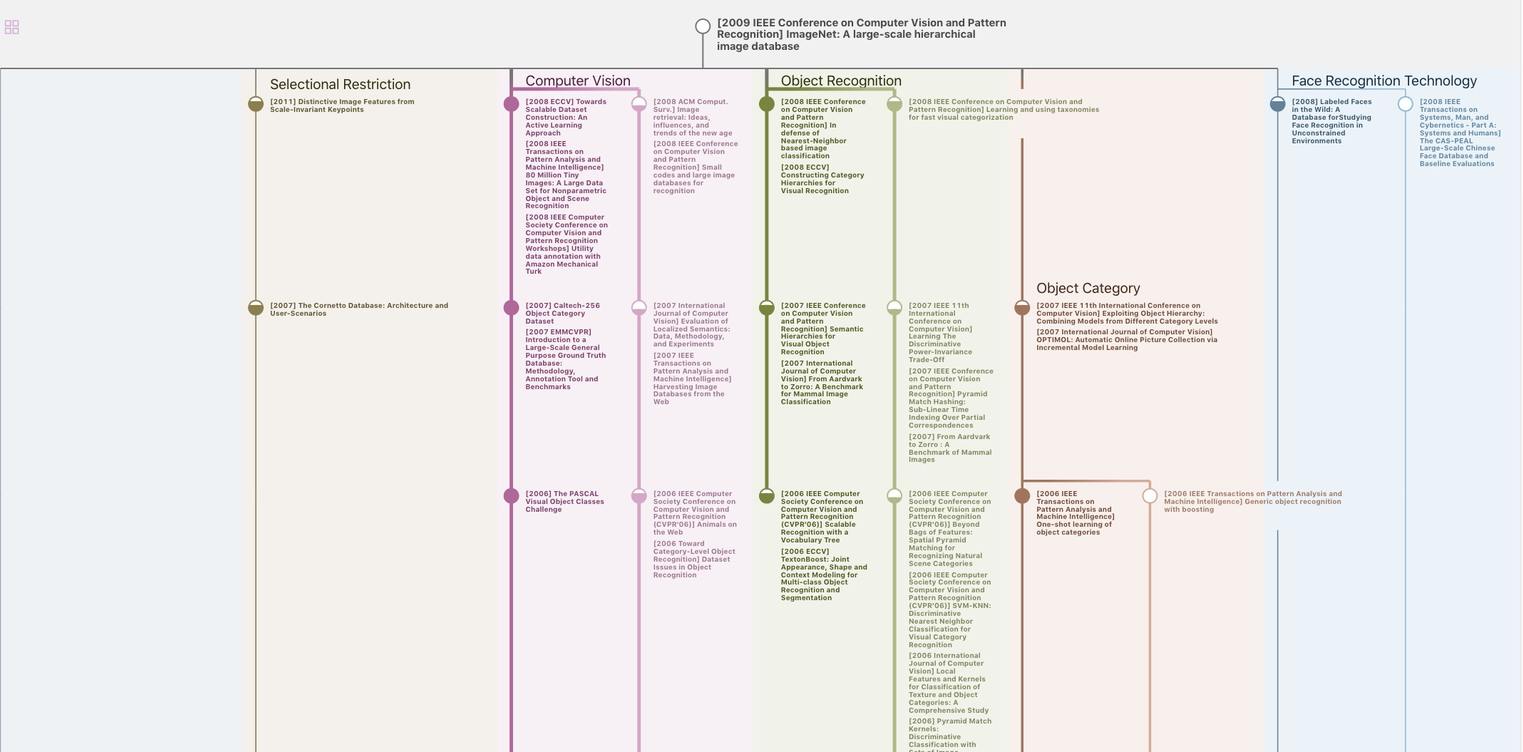
生成溯源树,研究论文发展脉络
Chat Paper
正在生成论文摘要