A Two-Stage Bayesian Small Area Estimation Method for Proportions
arXiv (Cornell University)(2023)
摘要
With the rise in popularity of digital Atlases to communicate spatial variation, there is an increasing need for robust small-area estimates. However, current small-area estimation methods suffer from various modelling problems when data are very sparse or when estimates are required for areas with very small populations. These issues are particularly heightened when modelling proportions. Additionally, recent work has shown significant benefits in modelling at both the individual and area levels. We propose a two-stage Bayesian hierarchical small area estimation model for proportions that can: account for survey design; use both individual-level survey-only covariates and area-level census covariates; reduce direct estimate instability; and generate prevalence estimates for small areas with no survey data. Using a simulation study we show that, compared with existing Bayesian small area estimation methods, our model can provide optimal predictive performance (Bayesian mean relative root mean squared error, mean absolute relative bias and coverage) of proportions under a variety of data conditions, including very sparse and unstable data. To assess the model in practice, we compare modeled estimates of current smoking prevalence for 1,630 small areas in Australia using the 2017-2018 National Health Survey data combined with 2016 census data.
更多查看译文
关键词
area,estimation,small,two-stage
AI 理解论文
溯源树
样例
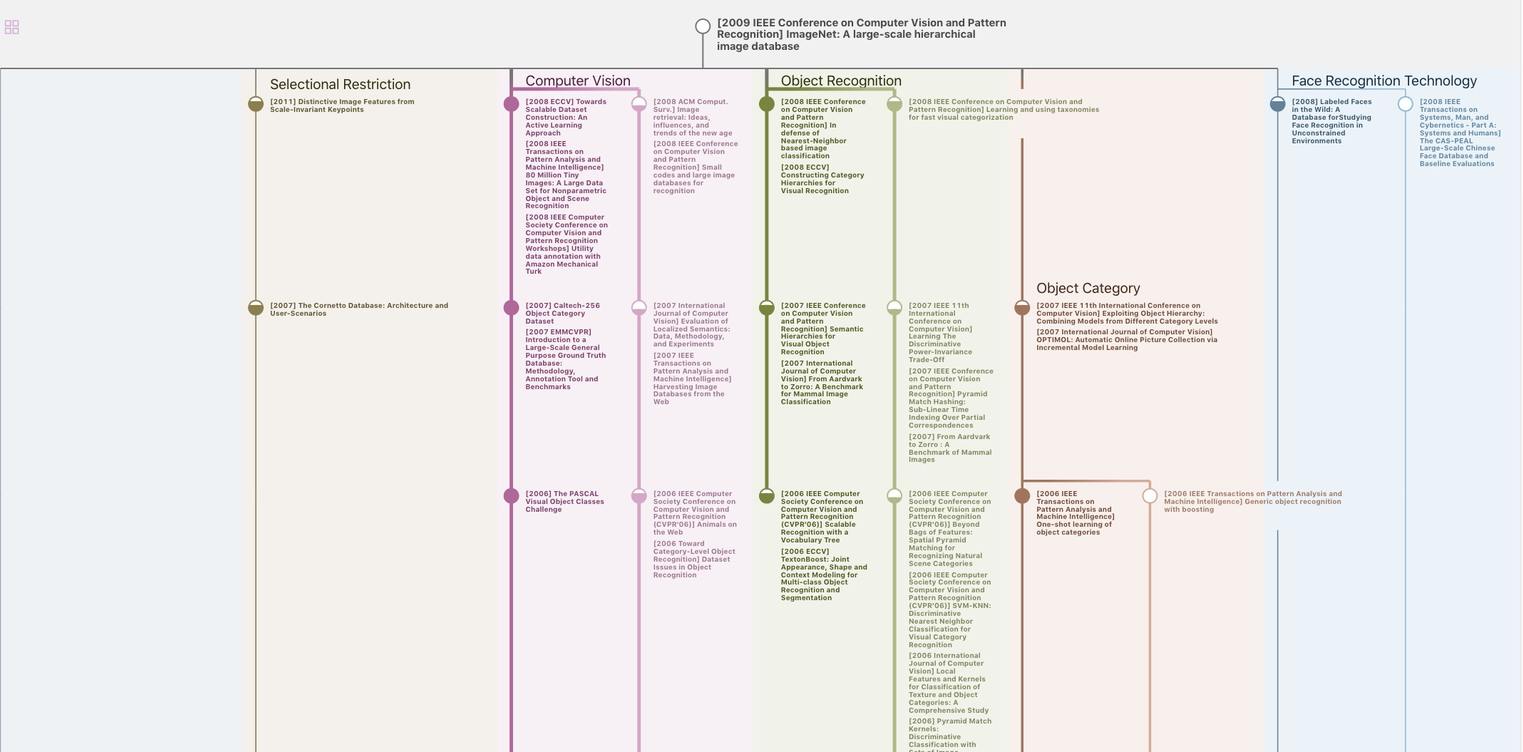
生成溯源树,研究论文发展脉络
Chat Paper
正在生成论文摘要