LVVC: A Learned Versatile Video Coding Framework for Efficient Human-Machine Vision
CoRR(2023)
Abstract
Almost all digital videos are coded into compact representations before being transmitted. Such compact representations need to be decoded back to pixels before being displayed to human and - as usual - before being processed/analyzed by machine vision algorithms. For machine vision, it is more efficient at least conceptually, to process/analyze the coded representations directly without decoding them into pixels. Motivated by this concept, we propose a learned versatile video coding (LVVC) framework, which targets on learning compact representations to support both decoding and direct processing/analysis, thereby being versatile for both human and machine vision. Our LVVC framework has a feature-based compression loop, where one frame is encoded (resp. decoded) to intermediate features, and the intermediate features are referenced for encoding (resp. decoding) the following frames. Our proposed feature-based compression loop has two key technologies, one is feature-based temporal context mining, and the other is cross-domain motion encoder/decoder. With the LVVC framework, the intermediate features may be used to reconstruct videos, or be fed into different task networks. The LVVC framework is implemented and evaluated with video reconstruction, video processing, and video analysis tasks on the well-established benchmark datasets. The evaluation results demonstrate the compression efficiency of the proposed LVVC framework.
MoreTranslated text
AI Read Science
Must-Reading Tree
Example
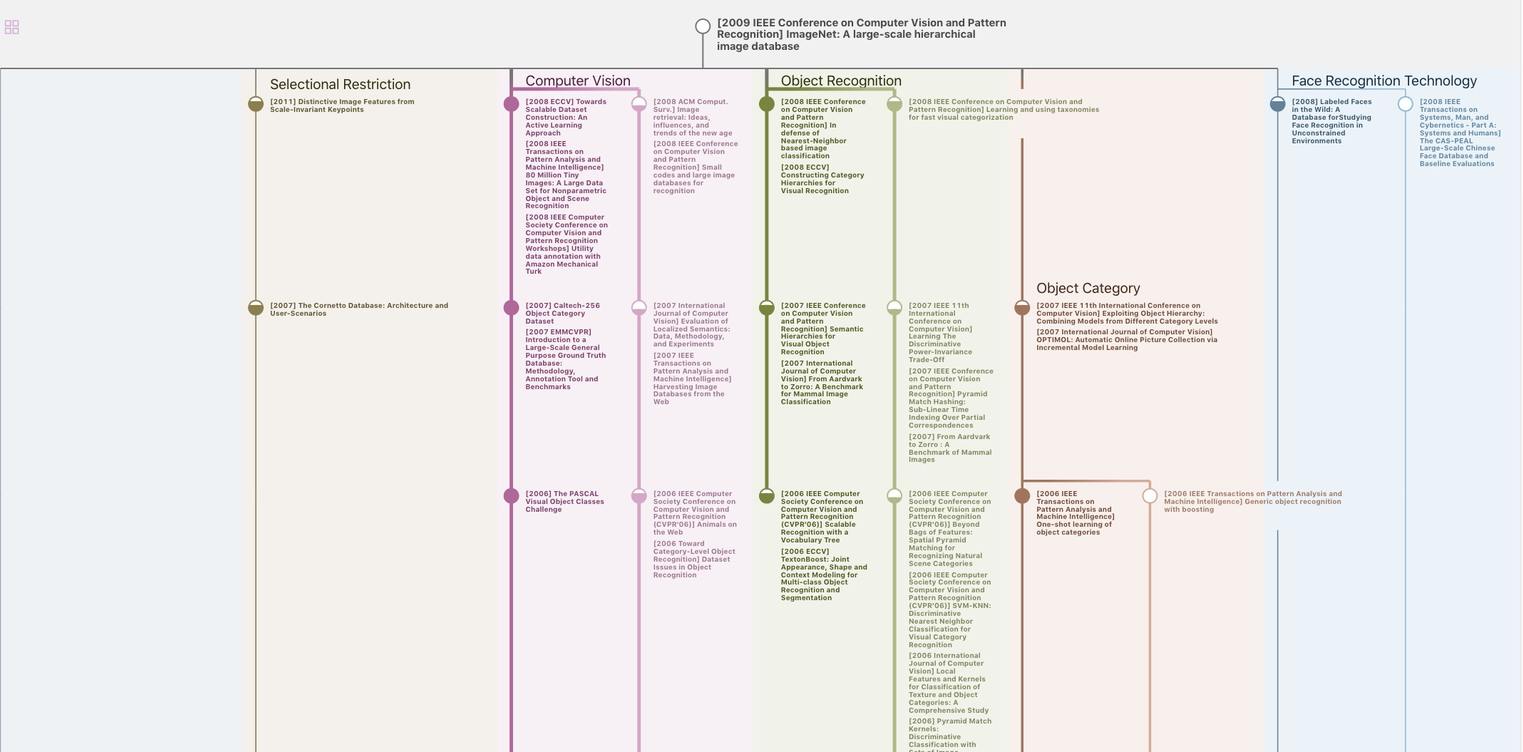
Generate MRT to find the research sequence of this paper
Chat Paper
Summary is being generated by the instructions you defined