P159. Unsupervised machine learning driven stratification of spinal cord injury patients for prediction of long-term outcomes
The Spine Journal(2022)
摘要
BACKGROUND CONTEXT Several studies have attempted to predict outcomes of spinal cord injury (SCI) patients, emphasizing the American Spinal Association (ASA) Scale or Functional Independence Measure (FIM). However, no studies to date have attempted to create a unified metric to define patient outcomes that span multiple domains. In this study, we attempt to create a novel machine learning driven classification system of long-term outcomes and attempt to observe natural clusters in combinations of known outcomes. METHODS We utilized the multi-institutional SCI Model Systems Public Access dataset to identify patients. We selected 7 outcomes consistently reported at 1-year and performed dimensionality reduction using t-distributed stochastic network embedding (tSNE) and identified clusters using unsupervised k-mean clustering. We used patient features collected at admission and during the initial hospitalization to predict cluster membership. RESULTS A total of 4,830 patients were identified. Four natural clusters emerged from our analysis of outcomes. Cluster C was associated with improved outcomes in multiple outcome metrics. Cluster A and B were able to differentiate between independence and mobility. We sought to predict membership in Cluster C from data only collected during the initial hospitalization and rehabilitation of the patient. We tested six classification algorithms to predict membership into Cluster C with 5-fold cross-validation. Adaptive Boosted (AdaBoost) clustering proved to have the highest accuracy of 78.7%, and an AUC of 0.74. CONCLUSIONS We demonstrate the capacity of unsupervised machine learning models to identify new patterns to better prognosticate long-term outcomes for SCI patients. We identify membership to a unique cluster of SCI patients associated with significantly better outcomes at 1-year followup. FDA DEVICE/DRUG STATUS This abstract does not discuss or include any applicable devices or drugs. Several studies have attempted to predict outcomes of spinal cord injury (SCI) patients, emphasizing the American Spinal Association (ASA) Scale or Functional Independence Measure (FIM). However, no studies to date have attempted to create a unified metric to define patient outcomes that span multiple domains. In this study, we attempt to create a novel machine learning driven classification system of long-term outcomes and attempt to observe natural clusters in combinations of known outcomes. We utilized the multi-institutional SCI Model Systems Public Access dataset to identify patients. We selected 7 outcomes consistently reported at 1-year and performed dimensionality reduction using t-distributed stochastic network embedding (tSNE) and identified clusters using unsupervised k-mean clustering. We used patient features collected at admission and during the initial hospitalization to predict cluster membership. A total of 4,830 patients were identified. Four natural clusters emerged from our analysis of outcomes. Cluster C was associated with improved outcomes in multiple outcome metrics. Cluster A and B were able to differentiate between independence and mobility. We sought to predict membership in Cluster C from data only collected during the initial hospitalization and rehabilitation of the patient. We tested six classification algorithms to predict membership into Cluster C with 5-fold cross-validation. Adaptive Boosted (AdaBoost) clustering proved to have the highest accuracy of 78.7%, and an AUC of 0.74. We demonstrate the capacity of unsupervised machine learning models to identify new patterns to better prognosticate long-term outcomes for SCI patients. We identify membership to a unique cluster of SCI patients associated with significantly better outcomes at 1-year followup.
更多查看译文
关键词
spinal cord injury patients,spinal cord injury,spinal cord,stratification,machine learning,long-term
AI 理解论文
溯源树
样例
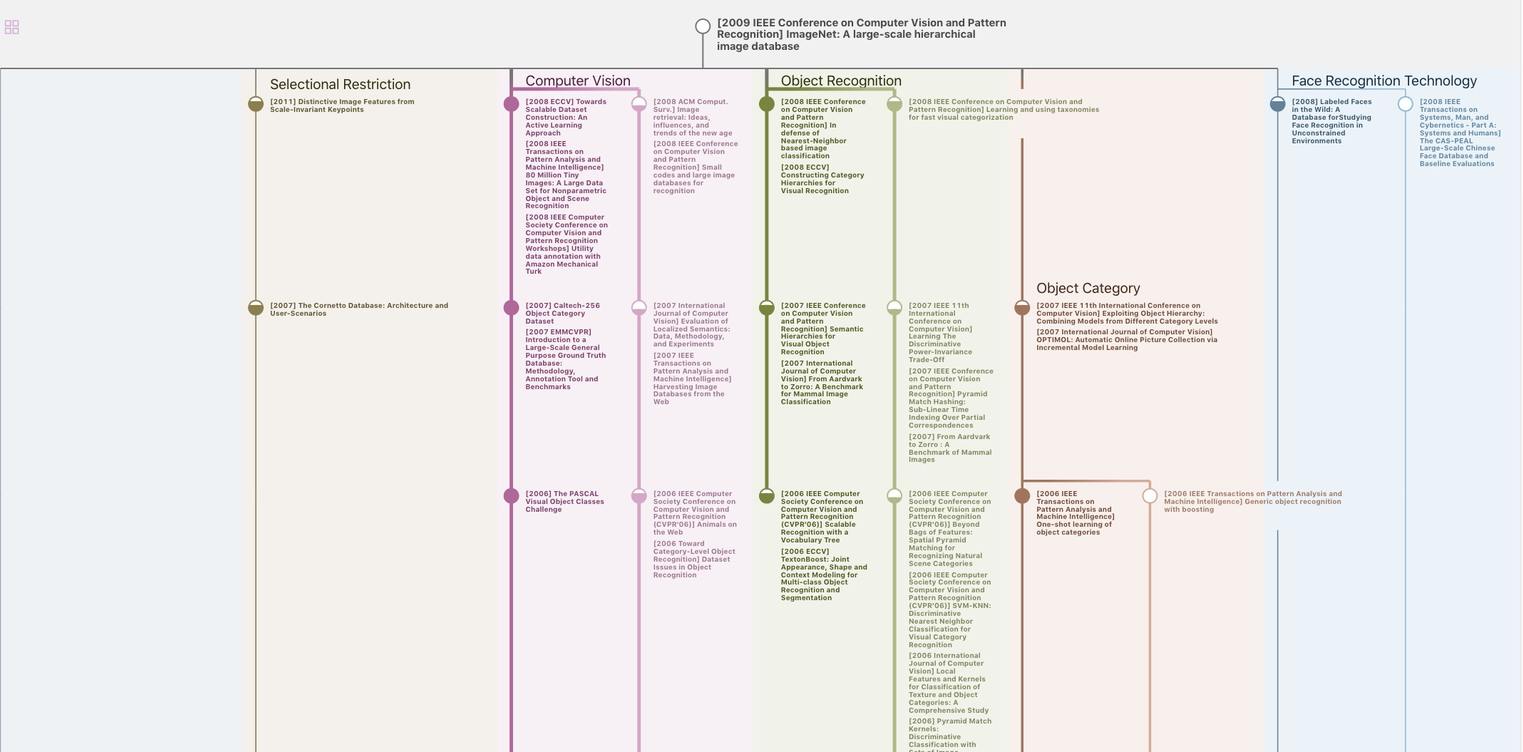
生成溯源树,研究论文发展脉络
Chat Paper
正在生成论文摘要