Neuron-Based Spiking Transmission and Reasoning Network for Robust Image-Text Retrieval
IEEE Transactions on Circuits and Systems for Video Technology(2023)
摘要
Most of the image-text retrieval methods carry out accurate results using fine-grained features for feature alignment. However, extracting the robustness features while maintaining the retrieval accuracy in wireless communication is still a challenge, especially with channel noises and limited transmission bandwidth. Inspired by spike signals of neurons in the human brain, we propose the neuron-based spiking transmission and reasoning network (NSTRN). In this way, the features are compressed into compacted efficient representations. In NSTRN, we construct the feature sender based on spiking activation function to selectively encode only important information in images and sentences into binary codes, and reduce the transmission cost. Moreover, the feature receiver is designed as a recurrent architecture and applies both temporal attention and global attention blocks to memorize long-term information. Finally, to compensate for the loss of visual concepts in transmission, we use the global textual features as coefficients to guide the formation of visual features in the training stage. The traditional CNN-based joint source-channel coding model outputs float-point encoded features, which requires additional quantization steps to convert features into binary bitstreams in the practical wireless communication system. Instead, the spiking neural networks (SNNs) directly use binary spike trains to reduce the computation complexity caused by the quantization steps. More importantly, SNNs can naturally encode the asynchronous event streams and inhibit the discrete noisy events to extract robust information. Even with binary bitstreams, NSTRN shows effectiveness compared with the state-of-the-art image-text retrieval methods. In the wireless communication scenario, NSTRN not only reduces the transmission bandwidth but also alleviates the "cliff effect" to a certain extent in the traditional separate encoding methods. To the best of our knowledge, this is the first work using SNNs on robust image-text retrieval.
更多查看译文
关键词
Image-text retrieval,spiking neural networks,joint source-channel coding
AI 理解论文
溯源树
样例
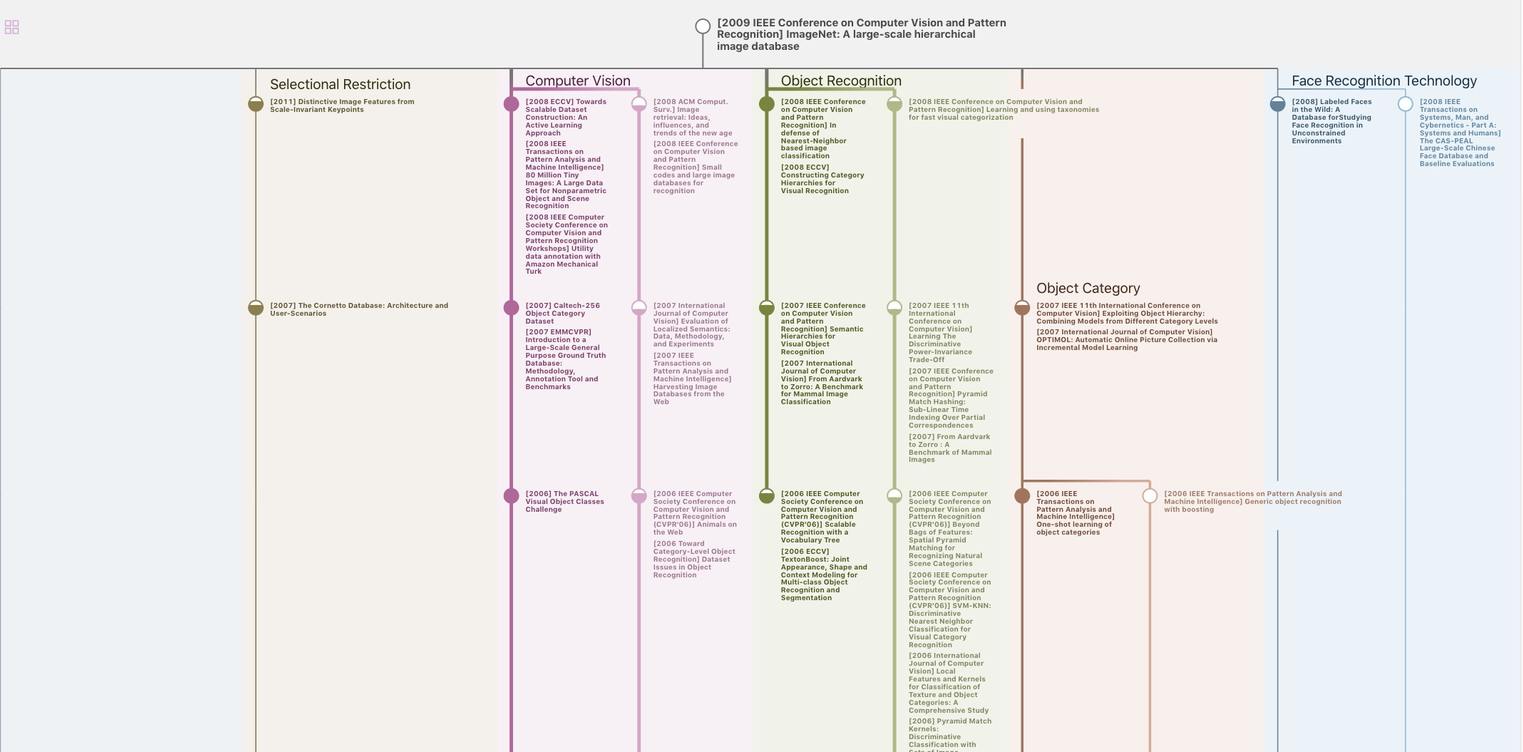
生成溯源树,研究论文发展脉络
Chat Paper
正在生成论文摘要