Memristor based Reservoir Network for Chaotic Time Series Prediction
2022 International Conference on Electrical, Computer, Communications and Mechatronics Engineering (ICECCME)(2022)
摘要
Reservoir Computing (RC) is a highly efficient emerging computing concept in machine learning to process temporal signals and has a low training cost compared to the traditional recurrent neural network. At first, the RC system extracts features from the input dataset and then projects the data in the high dimensional space by creating rich reservoir states. There are two conventional RC approaches such as Echo State Network (ESN) and Liquid State Machine (LSM). In this work, we explore a recent volatile memristor-based RC paradigm with spike encoded input which is attractive for its compact hardware implementation. We use four reported volatile memristors in this paradigm along with two reservoir architectures for discrete-time chaotic time series prediction. Chaotic time series is highly sensitive to the initial condition and slightly change in the initial condition causes eventual divergence in the observed outputs. Logistic map and Henon map are chosen as representative examples of well-known one-dimensional and two-dimensional chaotic maps, respectively. The main goal of this work is to explore and compare the performance of four different memristive RC systems using two different reservoir architectures for chaotic time series prediction.
更多查看译文
关键词
chaotic time series prediction,reservoir network
AI 理解论文
溯源树
样例
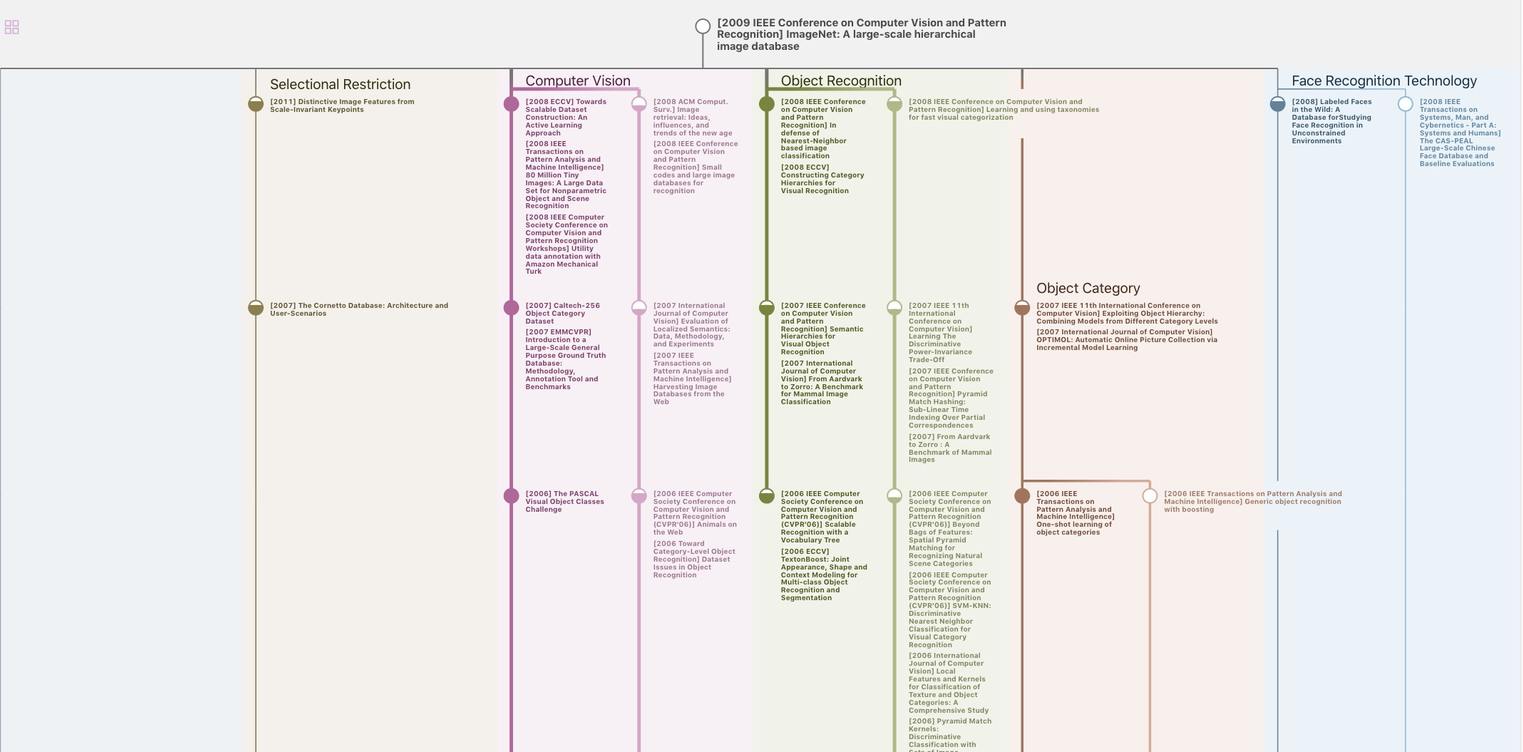
生成溯源树,研究论文发展脉络
Chat Paper
正在生成论文摘要