Hierarchical Group Lasso Regression for Efficiently Identifying Interactions in Healthcare Risk-Adjustment Models
Research Square (Research Square)(2022)
摘要
Abstract Researchers use risk-adjustment models to account for severity of illness in comparing patient outcomes across hospitals. Researchers specify important features as main effects, but they ignore interaction effects or use stratification methods, to account for effect modification, despite its limitations resulting from the number of potential strata. The Agency for Healthcare Research and Quality Inpatient Quality Indicator (IQI) 11, Abdominal Aortic Aneurysm Repair Mortality, is stratified into four groups based on the type of repair and rupture. IQI 09, Pancreatic Resection Mortality Rate, is stratified into two groups based on the presence of pancreatic cancer. The variable performance of these models motivated design of better models. We used hierarchical group lasso regularisation (HGLR) to identify first-order interactions in patient discharge data used in the development of risk-standardised models. HGLR identified unsuspected but clinically meaningful interactions for IQI 11, which has a heterogeneous population, but not for IQI 09. Synergistic interactions, such as between hypertension and respiratory failure, suggest patients merit special attention in perioperative care. Antagonistic interactions, such as between shock at admission and chronic comorbidities, illustrate that naïve main effects models overpredict risk in key population subsets, leading to miscalibrated models that yield biased estimates of hospital performance. HGLR is a useful and necessary step to identify first-order interactions for the development of robust risk-adjustment models used by agencies and stakeholders to rank hospitals in the US.
更多查看译文
关键词
hierarchical group lasso regression,healthcare,models,risk-adjustment
AI 理解论文
溯源树
样例
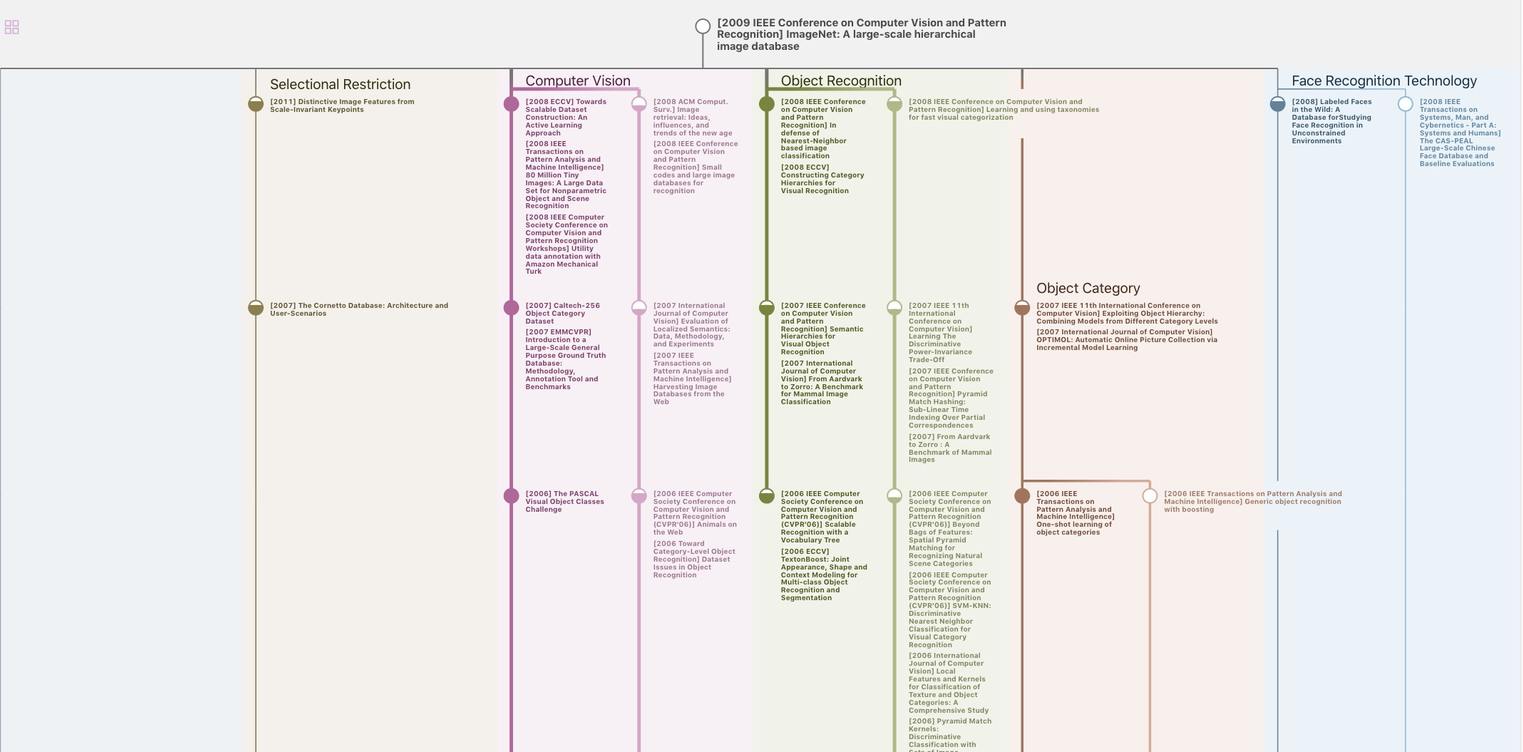
生成溯源树,研究论文发展脉络
Chat Paper
正在生成论文摘要