SVMs-SKSM: Protein Function Multi-label Classification based on SVM-SVM classifiers Fusion and sequences kernel similarity matrix
crossref(2022)
摘要
Abstract Background: The assignment of function to proteins at a large scale is essential for understanding the molecular mechanism of life. However, in UniProtKB, only a very small percentage of the proteins have Gene Ontology (GO) annotations supported by experimental evidence, and some proteins may be performing different reactions and can hence be directly associated with multiple functions. Methods: To achieve more accurate classification, in this paper, we propose a multilabel classification method based on SVM-SVM classifiers fusion strategy and sequences kernel similarity matrix (named SVMs-SKSM). Firstly, we employ position specific scoring matrix (PSSM) and Gaussian Kernel similarity to get the similarity information between sequences, and introduce deep autoencoders to obtain structures interaction information. Secondly, we obtain the class probabilities features of sequences by the maximum similarity probability, and gain the class probabilities features of structures by SVM classifier. Lastly, we fuse the two class probabilities by the linear combination, and input the fused probabilities into a multi-label SVM to classify. Results: The experimental results indicate that the proposed method achieves superior performance with the accuracy of 40.7%, 34.2% and 44.5% in Level1, Level2and Level3 of yeast networks, and 54.6%, 54.4% and 57.1% in MF[101-300], BP[101-300]and CC[101-300] of human networks.Conclusions: In future work, we will consider improving feature extraction as well as building advanced prediction models to achieve higher prediction accuracy.
更多查看译文
AI 理解论文
溯源树
样例
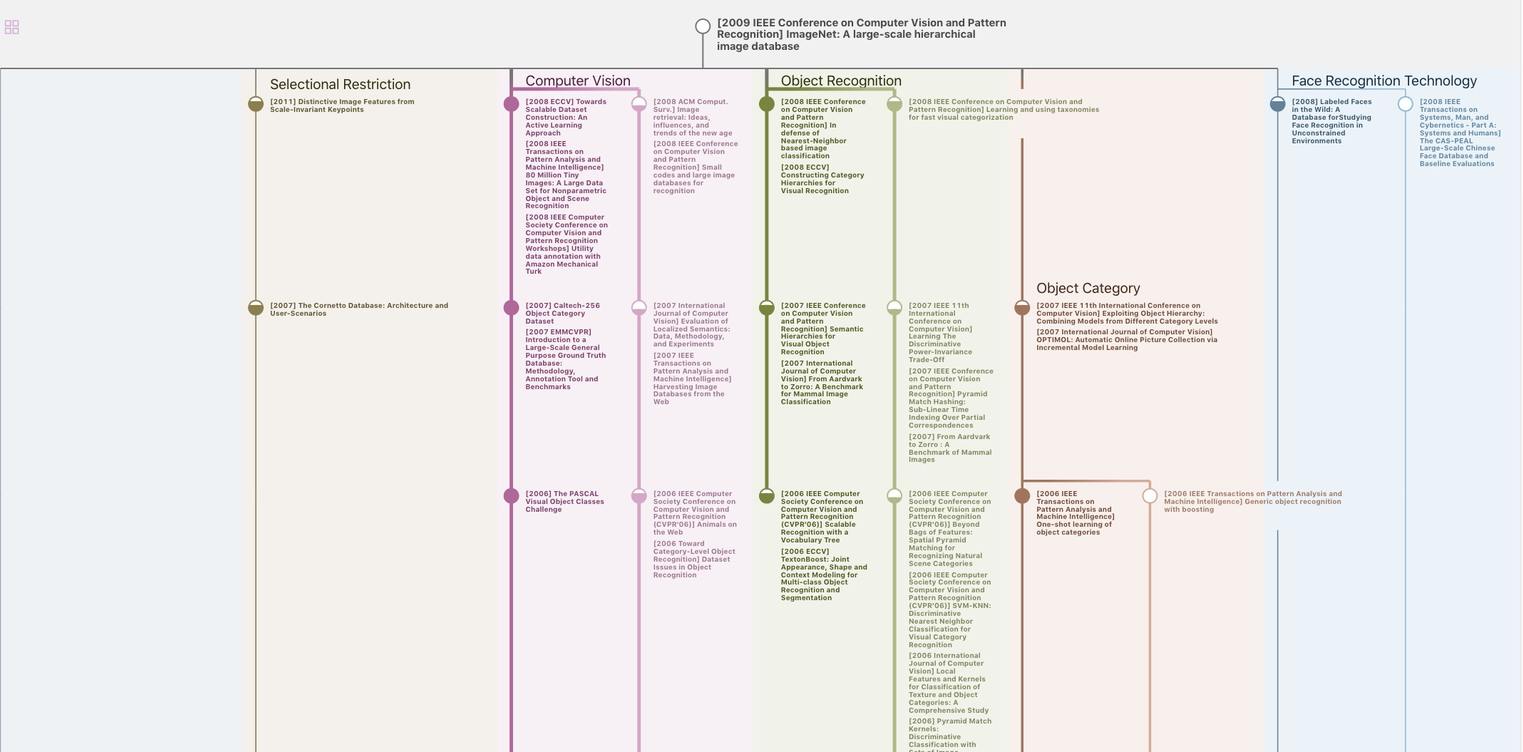
生成溯源树,研究论文发展脉络
Chat Paper
正在生成论文摘要