Improving generalization performance of electrocardiogram classification models.
Physiological Measurement(2023)
摘要
Recently, many electrocardiogram (ECG) classification algorithms using deep learning have been proposed. Because the ECG characteristics vary across datasets owing to variations in factors such as recorded hospitals and the race of participants, the model needs to have a consistently high generalization performance across datasets. In this study, as part of the PhysioNet/Computing in Cardiology Challenge (PhysioNet Challenge) 2021, we present a model to classify cardiac abnormalities from the 12- and the reduced-lead ECGs.To improve the generalization performance of our earlier proposed model, we adopted a practical suite of techniques, i.e. constant-weighted cross-entropy loss, additional features, mixup augmentation, squeeze/excitation block, and OneCycle learning rate scheduler. We evaluated its generalization performance using the leave-one-dataset-out cross-validation setting. Furthermore, we demonstrate that the knowledge distillation from the 12-lead and large-teacher models improved the performance of the reduced-lead and small-student models.With the proposed model, our DSAIL SNU team has received Challenge scores of 0.55, 0.58, 0.58, 0.57, and 0.57 (ranked 2nd, 1st, 1st, 2nd, and 2nd of 39 teams) for the 12-, 6-, 4-, 3-, and 2-lead versions of the hidden test set, respectively.The proposed model achieved a higher generalization performance over six different hidden test datasets than the one we submitted to the PhysioNet Challenge 2020.
更多查看译文
关键词
electrocardiogram classification models,generalization performance
AI 理解论文
溯源树
样例
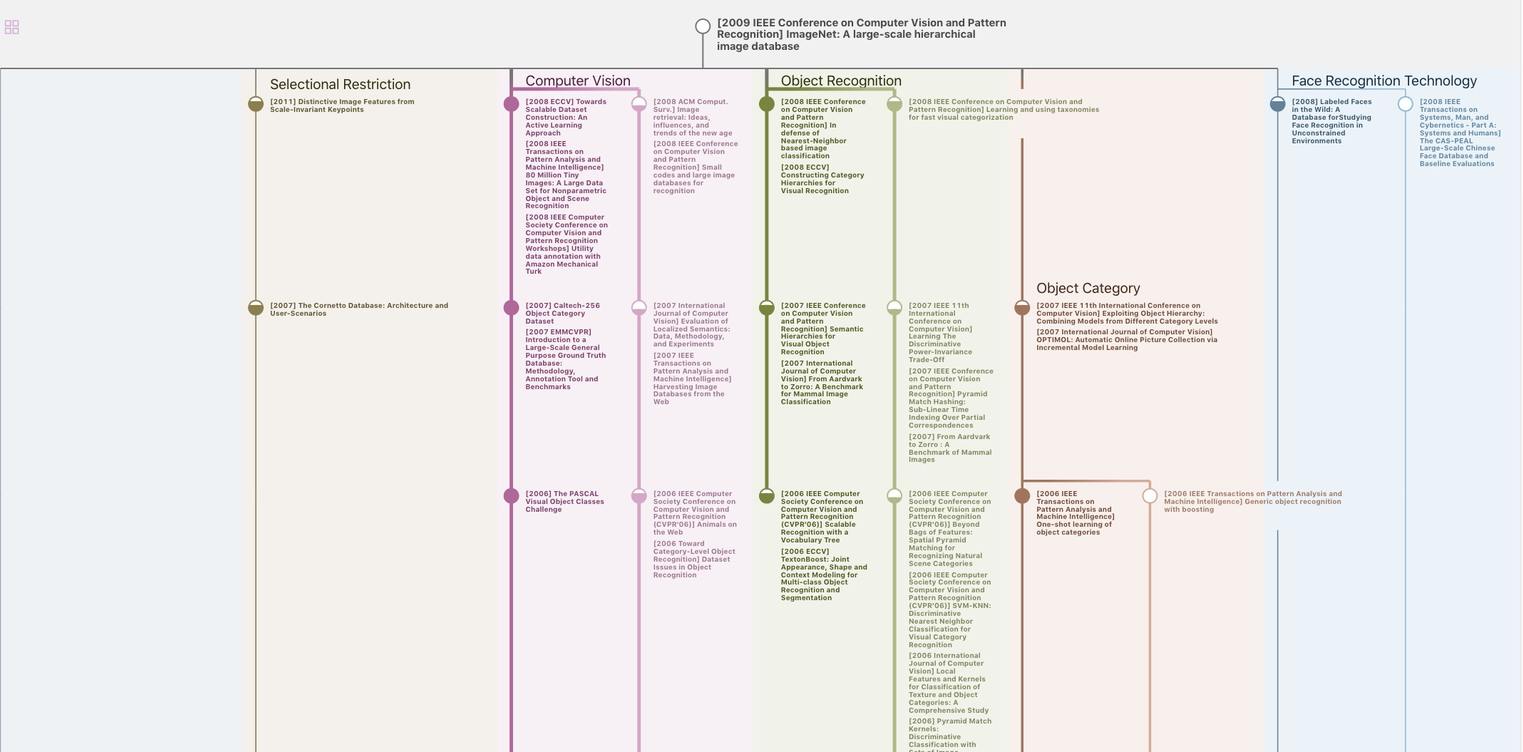
生成溯源树,研究论文发展脉络
Chat Paper
正在生成论文摘要