Measurement error and Regression Calibration method for multiple exposures: a Simulation study within the EXPANSE project
ISEE Conference Abstracts(2022)
摘要
Background and Aim Exposure Measurement error (ME) in air pollution leads to imprecise and biased health effects estimates, while its impact when multiple exposures are considered presents additional challenges. Within the EXPANSE project where multiple exposures are investigated we assessed the application of regression calibration (RC) as an approach to correct effect estimates in multi-exposure Cox models. Methods We simulated data informed by the Cardiovascular Effects of Air Pollution and Noise in Stockholm cohort in order to investigate multiple exposures effects on cardiovascular mortality applying Cox regression models. PM2.5, NO2 and road traffic noise were considered as ME-prone exposures. We simulated the "true” exposure levels for each exposure by subtracting ME from surrogate measures under two different scenarios derived from the exposure assessment methods. The observed correlations between the three exposures ranged from 0.3 to 0.7. We simulated survival data for cardiovascular mortality based on the "true” effect estimates of the multi adjusted exposures under two scenarios. The average surrogate and RC-corrected effect estimates across 1000 repetitions were assessed. Estimates are reported as Hazard Ratios (HR) for 1-unit increase in respective exposures. Results Prevailing classical-type error was defined for noise, while Berkson-type error for PM2.5 and NO2. Under the scenario with average performing exposure assessment (R2: 0.39-0.66), the surrogate estimates underestimated all associations and the RC correction slightly reduced the bias, except for PM2.5. Under the scenario of better exposure assessment (R2:0.68-0.77), the surrogate exposures underestimated the PM2.5 and noise effect while the RC correction reduced bias for all exposures (HR PM2.5: True 1.000 vs. RC 1.000; HR NO2: True 0.998 vs. RC 1.000; HR Noise: True 1.004 vs. RC 1.004). Conclusions RC performed well in both scenarios and reduced the bias of most effect estimates in multi-exposure Cox models. Keywords: Environmental Exposures, Measurement Error, Regression Calibration
更多查看译文
关键词
multiple exposures,regression calibration method,calibration method,measurement
AI 理解论文
溯源树
样例
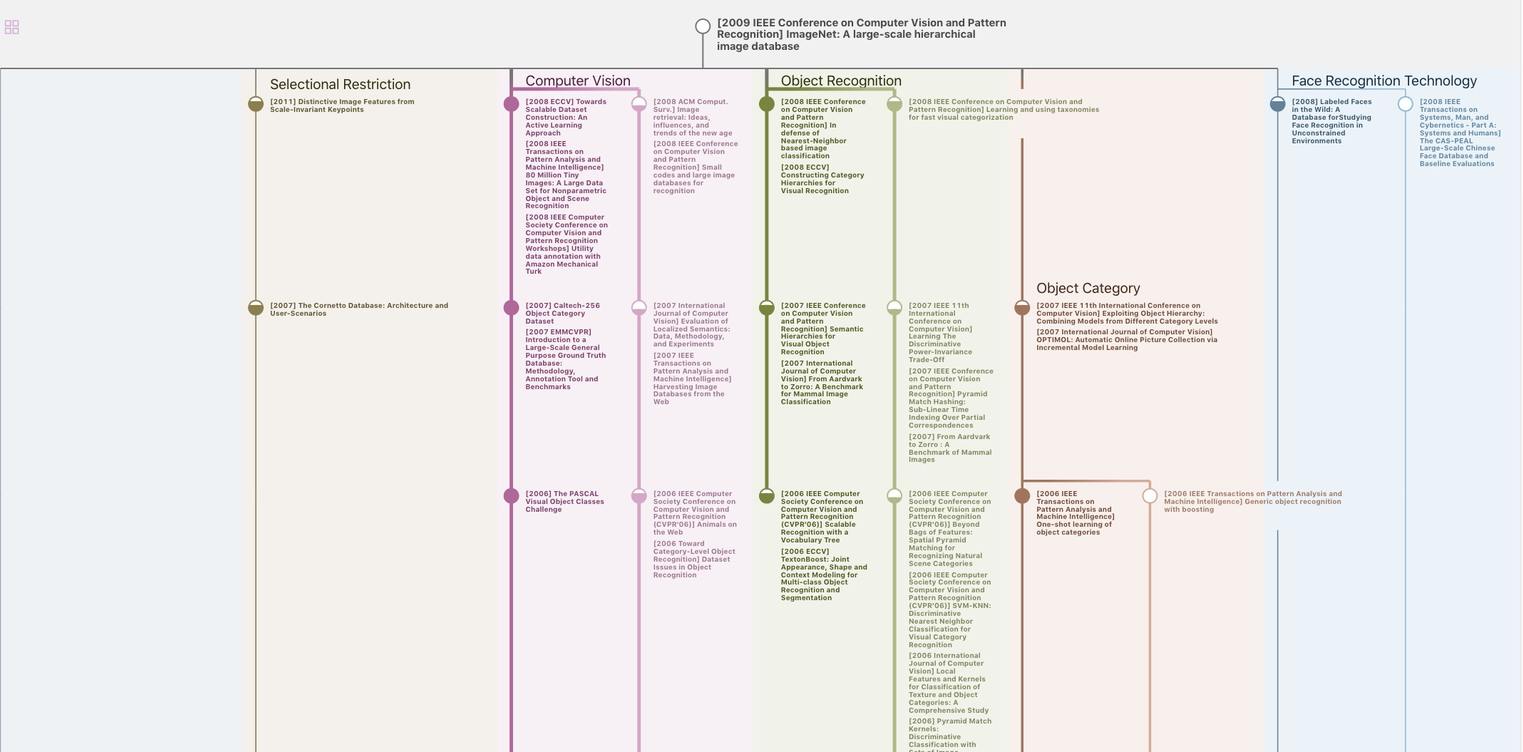
生成溯源树,研究论文发展脉络
Chat Paper
正在生成论文摘要