Biological studies with comprehensive GCxGC-HRMS screening: Exploring the human sweat volatilome
crossref(2022)
摘要
A key issue in GCxGC-HRMS data analysis is how to approach large-sample studies in an efficient and comprehensive way. We have developed a semi-automated data-driven workflow from identification to suspect screening, which allows highly selective monitoring of each identified chemical in a large-sample dataset. The example dataset used to illustrate the potential of the approach consisted of human sweat samples from 40 participants, including field blanks (80 samples). These samples have been collected in a Horizon 2020 project to investigate the capacity of body odour to communicate emotion and influence social behaviour. We used dynamic headspace extraction, which allows comprehensive extraction with high preconcentration capability, and has to date only been used for a few biological applications. We found 325 sweat volatiles compared to 581 known skin volatiles, 107 of which matched perfectly with the literature, and 218 compounds (67%) novel sweat volatiles. Unlike partitioning-based extraction methods, the developed method detects semi-polar (log P < 2) nitrogen and oxygen-containing compounds. However, it is unable to detect certain acids due to the pH conditions of unmodified sweat samples. Using a single analytical method, our coverage of the boiling point and polarity space was comparable to the cumulative coverage of all the available 35 studies investigating skin volatiles with different analytical methods. We believe that our framework will open up the possibility of efficiently using GCxGC-HRMS for large-sample studies in a wide range of applications such as biological and environmental studies.
更多查看译文
AI 理解论文
溯源树
样例
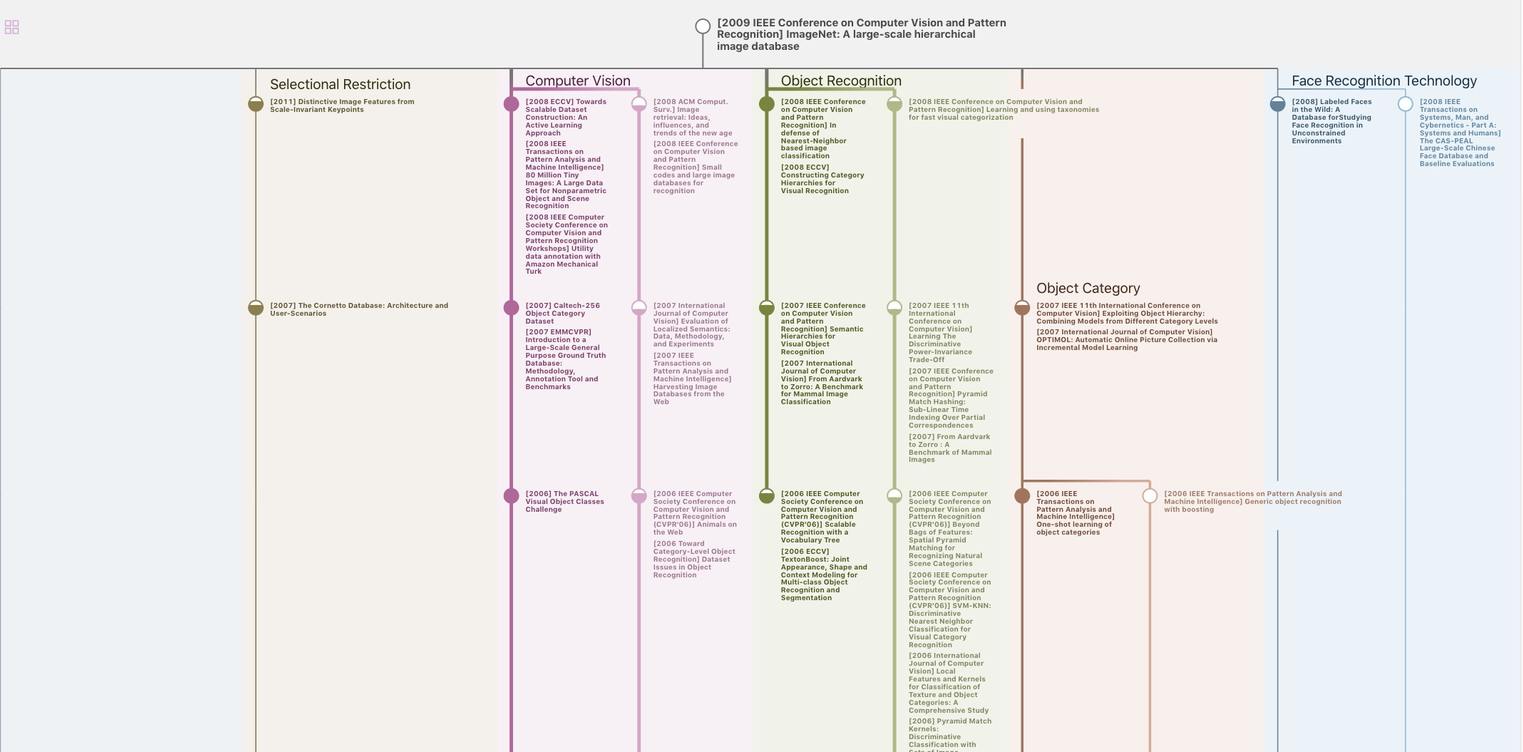
生成溯源树,研究论文发展脉络
Chat Paper
正在生成论文摘要